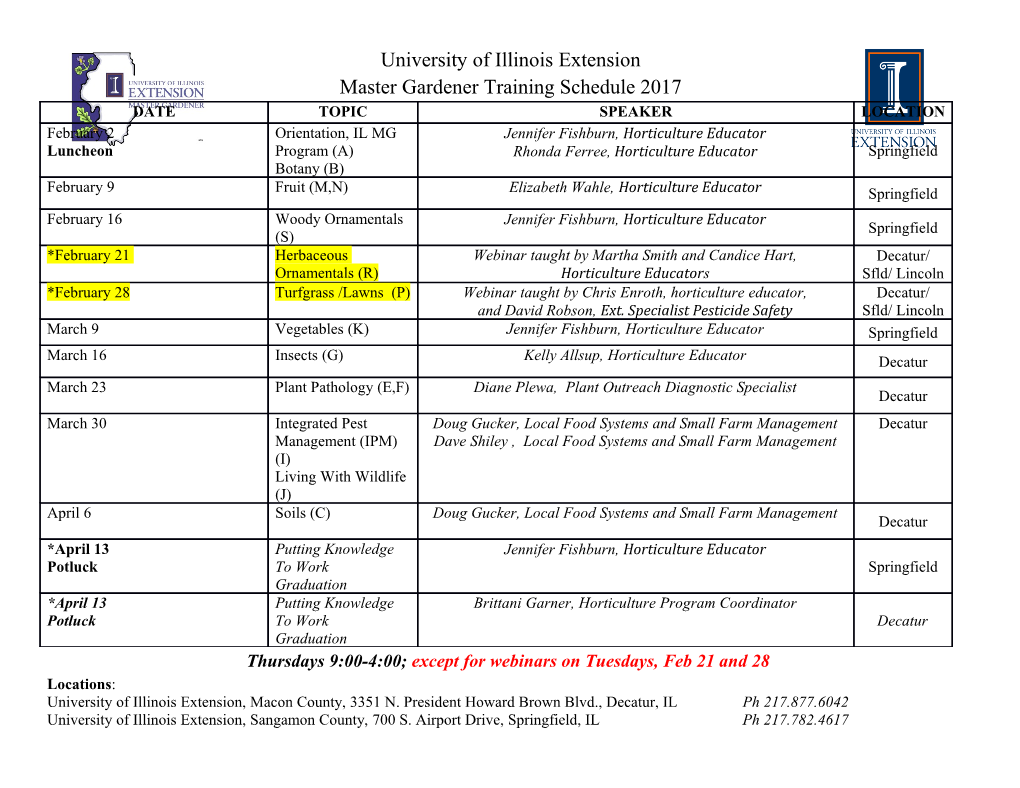
MUCS@ - Machine Translation for Dravidian Languages using Stacked Long Short Term Memory Asha Hegde Ibrahim Gashaw H. L. Shashirekha Dept of Computer Science Dept of Computer Science Dept of Computer Science Mangalore University Mangalore University Mangalore University [email protected] [email protected] [email protected] Abstract are small literary languages. All these languages except Kodava have their own script. Further, these The Dravidian language family is one of 1 the largest language families in the world. languages consists of 80 different dialects namely In spite of its uniqueness, Dravidian lan- Brahui, Kurukh, Malto, Kui, Kuvi, etc. Dravid- guages have gained very less attention due ian Languages are mainly spoken in southern In- to scarcity of resources to conduct language dia, Sri Lanka, some parts of Pakistan and Nepal technology tasks such as translation, Parts- by over 222 million people (Hammarstrom¨ et al., of-Speech tagging, Word Sense Disambigua- 2017). It is thought that Dravidian languages are tion etc. In this paper, we, team MUCS, native to the Indian subcontinent and were origi- describe sequence-to-sequence stacked Long nally spread throughout India1. Tamil have been Short Term Memory (LSTM) based Neural Machine Translation (NMT) models submit- distributed to Burma, Indonesia, Malaysia, Fiji, ted to “Machine Translation in Dravidian lan- Madagascar, Mauritius, Guyana, Martinique and guages”, a shared task organized by EACL- Trinidad through trade and emigration. With over 2021. The NMT models are applied for trans- two million speakers, primarily in Pakistan and two lation using English-Tamil, English-Telugu, million speakers in Afghanistan, Brahui is the only English-Malayalam and Tamil-Telugu corpora Dravidian language spoken entirely outside India provided by the organizers. Standard evalua- (Ethnologue)2. Rest of the Dravidian languages are tion metrics namely Bilingual Evaluation Un- derstudy (BLEU) and human evaluations are extensively spoken inside India and by south Indi- used to evaluate the model. Our models exhib- ans settled throughout the world. These languages ited good accuracy for all the language pairs share similar linguistic features and few of them and obtained 2nd rank for Tamil-Telugu lan- are listed below (Unnikrishnan et al., 2010): guage pair. • Verbs have a negative as well as an affirmative 1 Introduction voice. Human being is a social entity and they love to • Root word can be extended using one or more communicate with each other and live together suffixes or prefixes according to the comfort from ancient times. They communicate with each level of the speaker. other through different means of communication and exchange their data/information/thoughts with • Phonology of all Dravidian languages follow each other. Initially, sign language was the means similar strategy. of communication and it was used to exchange • Though these languages follow Subject- thoughts with each other, but now, in this era of Object-Verb word order, they are considered technology, there are many ways to communicate as free word order languages as meaning of out of which language plays a major role. There the sentence will not change when the word are more than thousands of languages used all over order is changed. the world, even in India, due to different religion, culture and tradition. Among Indian languages Dra- 1https://www.shh.mpg.de/870797/dravidian-languages 1 vidian is a language family consisting of four long https://www.mustgo.com/ worldlanguages/dravidian-language-family literary languages, namely, Tamil, Kannada, Telugu 2http://self.gutenberg.org/articles/ and Malayalam along with Tulu and Kodava which eng/Languages_of_Pakistan 340 Proceedings of the First Workshop on Speech and Language Technologies for Dravidian Languages, pages 340–345 April 20, 2021 ©2021 Association for Computational Linguistics • Gender classification is made based on suffix. the most popular architectures namely Encoder- Decoder, Sequence-to-Sequence or Recurrent Neu- • Nouns are declined, showing case and num- ral Network (RNN) models (Sutskever et al., 2014). ber. In addition, all parts of the neural translation model • These languages have their own alphabets re- are trained jointly (end-to-end) to optimise trans- lated to the Devanagari alphabet that is used lation efficiency unlike traditional translation sys- for Sanskrit. tems (Bahdanau et al., 2014). In an NMT system, a bidirectional RNN, known as encoder is used to Though these languages have rich collection encode a source sentence and another RNN known of resources, they are still considered as under- as decoder is used to predict the target language 4 resourced languages due to the availability of terms. With multiple layers, this encoder-decoder very less digital resources and tools. Most of the architecture can be built to improve the translation south Indians speak/understand only their native performance of the system. The rest of the paper language as a means of communication. Further, is organized as follows: Related work is presented because of migration people need to learn the lo- in Section 2 followed by Methodology and Dataset cal languages to survive. In this regard, Language in Section 3. Experiments and Results are given in Translation (LT) technology gains importance as Section 4 and the paper concludes in Section 5. it provides the easy means of communication be- tween people when language barrier is a major is- 2 Related Work sue. Various LT technologies are available, namely, manual translation or human translation, Machine Many attempts are being carried out by the re- aided translation and automatic translation or Ma- searchers to give special attention for Dravidian chine Translation (MT). As MT is fast, inexpensive, language family and several researches have made reliable and consistent compared to other LT tech- noticeable work in this direction (Chakravarthi nologies, they are gaining popularity. et al., 2019a). NMT is a promising technique to establish sentence level translation and suitable pre- 1.1 Machine Translation Approaches processing techniques will share their contribution MT is an area in Natural Language Processing and for better performance. Observing this (Choud- does translation of information from one natural hary et al., 2018) have applied Byte Pair Encoding language to another natural language by retaining (BPE) for their model to resolve Out-of-Vocabulary the meaning of source context. Initially, MT task problem. They used EnTam V2.0 dataset that was treated with dictionary matching techniques contains English-Tamil parallel sentences. Their and upgraded slowly to rule-based approaches model exhibited improvement in BLEU score of (Dove et al., 2012). In order to address information 8.33 for Bidirectional LSTM+Adam (optimizer) acquisition, corpus-based methods have become + Bahdanau (attention) + BPE + word Embed- popular and bilingual parallel corpora have been ding. Multi-modal multilingual MT system uti- used to acquire knowledge for translation (Britz lizing phonetic transcription was implemented by et al., 2017). Hybrid MT approaches have also be- (Chakravarthi et al., 2019b) using under-resourced come popular along with corpus-based approaches, Dravidian languages. As a part of this work they as these approaches promise state-of-the-art perfor- have released MMDravi - a Dravidian language mance. dataset that comprises of 30,000 sentences. They The recent shift to large-scale analytical tech- have conducted both Statistical Machine Transla- niques has led to substantial changes in the ef- tion (SMT) and NMT and the NMT model outper- ficiency of MT. It has attracted the attention of formed SMT in terms of Bilingual Evaluation Un- MT researchers through a corpus based approach. der Study (BLEU) score. (Pareek et al., 2017) have NMT has now become an important alternative to proposed a novel Machine Learning based transla- conventional Statistical Machine Translation based tion approach for Kannada-Telugu Dravidian lan- on phrases (Patil and Davies, 2014). It is the guage pair considering wikipedia dataset. Fur- task of translating text from one natural language ther, they considered English-Kannada and English- (source) to another natural language (target) using Telugu language pairs for illustrating the efficacy 4https://en.unesco.org/news/towards-world-atlas- of their model. They have proposed n-grams based languages connecting phrase extraction and these extracted 341 phrases are trained in multilayered neural network. the decoder uses conditional probability to produce For testing, the phrases are extracted from test the predicted sentences b1, b2, b3, ... , bn word- dataset and alignment score is computed and then by-word. While decoding, next word is predicted these phrases are used for post-processing. They using previously predicted word vectors and source have observed a considerable accuracy of 91.63%, sentence vectors in equation 1 and equation 2 is 90.65% and 88.63% for English-Kannada, English- derived from the equation 1. Each term in the dis- Telugu and Kannada-Telugu, respectively. Dictio- tribution is represented with a softmax over all the nary based MT for Kannada to Telugu is developed words in the vocabulary (Neubig, 2017). by (Sindhu and Sagar, 2017) and as a part of this work, a bilingual dictionary with 8000 words is P (R jS) = P (R jAs1;As2; :::; Asn) (1) developed. Providing suffix mapping table at the P (R jS) = P (b ; b ; ::::b ; s ; s ; :::; s ) (2) tag level, this
Details
-
File Typepdf
-
Upload Time-
-
Content LanguagesEnglish
-
Upload UserAnonymous/Not logged-in
-
File Pages6 Page
-
File Size-