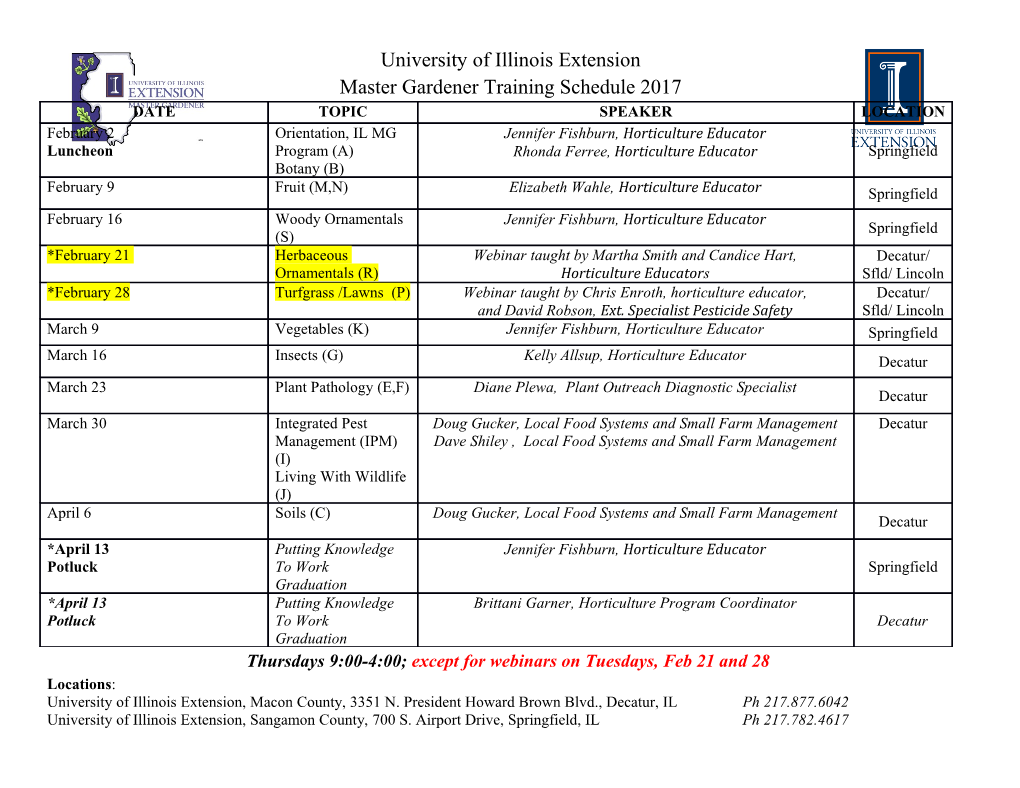
Data structures PDF generated using the open source mwlib toolkit. See http://code.pediapress.com/ for more information. PDF generated at: Thu, 17 Nov 2011 20:55:22 UTC Contents Articles Introduction 1 Data structure 1 Linked data structure 3 Succinct data structure 5 Implicit data structure 7 Compressed data structure 8 Search data structure 9 Persistent data structure 11 Concurrent data structure 15 Abstract data types 18 Abstract data type 18 List 26 Stack 29 Queue 57 Deque 60 Priority queue 63 Map 67 Bidirectional map 70 Multimap 71 Set 72 Tree 76 Arrays 79 Array data structure 79 Row-major order 84 Dope vector 86 Iliffe vector 87 Dynamic array 88 Hashed array tree 91 Gap buffer 92 Circular buffer 94 Sparse array 109 Bit array 110 Bitboard 115 Parallel array 119 Lookup table 121 Lists 127 Linked list 127 XOR linked list 143 Unrolled linked list 145 VList 147 Skip list 149 Self-organizing list 154 Binary trees 158 Binary tree 158 Binary search tree 166 Self-balancing binary search tree 176 Tree rotation 178 Weight-balanced tree 181 Threaded binary tree 182 AVL tree 188 Red-black tree 192 AA tree 207 Scapegoat tree 212 Splay tree 216 T-tree 230 Rope 233 Top Trees 238 Tango Trees 242 van Emde Boas tree 264 Cartesian tree 268 Treap 273 B-trees 276 B-tree 276 B+ tree 287 Dancing tree 291 2-3 tree 292 2-3-4 tree 293 Queaps 295 Fusion tree 299 Bx-tree 299 Heaps 303 Heap 303 Binary heap 305 Binomial heap 311 Fibonacci heap 316 2-3 heap 321 Pairing heap 321 Beap 324 Leftist tree 325 Skew heap 328 Soft heap 331 d-ary heap 333 Tries 335 Trie 335 Radix tree 342 Suffix tree 344 Suffix array 349 Compressed suffix array 352 FM-index 353 Generalised suffix tree 353 B-trie 355 Judy array 355 Directed acyclic word graph 357 Multiway trees 359 Ternary search tree 359 And–or tree 360 (a,b)-tree 362 Link/cut tree 363 SPQR tree 363 Spaghetti stack 366 Disjoint-set data structure 367 Space-partitioning trees 371 Space partitioning 371 Binary space partitioning 372 Segment tree 377 Interval tree 380 Range tree 385 Bin 386 k-d tree 388 Implicit k-d tree 395 min/max kd-tree 398 Adaptive k-d tree 399 Quadtree 399 Octree 402 Linear octrees 404 Z-order 404 UB-tree 409 R-tree 409 R+ tree 415 R* tree 416 Hilbert R-tree 419 X-tree 426 Metric tree 426 vP-tree 427 BK-tree 428 Hashes 429 Hash table 429 Hash function 442 Open addressing 450 Lazy deletion 453 Linear probing 453 Quadratic probing 454 Double hashing 458 Cuckoo hashing 459 Coalesced hashing 463 Perfect hash function 466 Universal hashing 468 Linear hashing 473 Extendible hashing 474 2-choice hashing 480 Pearson hashing 480 Fowler–Noll–Vo hash function 481 Bitstate hashing 483 Bloom filter 483 Locality preserving hashing 494 Morton number 495 Zobrist hashing 500 Rolling hash 501 Hash list 503 Hash tree 504 Prefix hash tree 506 Hash trie 506 Hash array mapped trie 507 Distributed hash table 508 Consistent hashing 513 Stable hashing 515 Koorde 515 Graphs 518 Graph 518 Adjacency list 520 Adjacency matrix 522 And-inverter graph 525 Binary decision diagram 527 Binary moment diagram 531 Zero-suppressed decision diagram 533 Propositional directed acyclic graph 534 Graph-structured stack 535 Scene graph 536 Appendix 541 Big O notation 541 Amortized analysis 551 Locality of reference 553 Standard Template Library 556 References Article Sources and Contributors 566 Image Sources, Licenses and Contributors 575 Article Licenses License 580 1 Introduction Data structure In computer science, a data structure is a particular way of storing and organizing data in a computer so that it can be used efficiently.[1] [2] Different kinds of data structures are suited to different kinds of applications, and some are highly specialized to specific tasks. For example, B-trees are particularly well-suited for implementation of databases, while compiler implementations usually use hash tables to look up identifiers. Data structures are used in almost every program or software system. Data structures provide a means to manage huge amounts of data efficiently, such as large databases and internet indexing services. Usually, efficient data structures are a key to designing efficient algorithms. Some formal design methods and programming languages emphasize data structures, rather than algorithms, as the key organizing factor in software design. Overview • An array stores a number of elements of the same type in a specific order. They are accessed using an integer to specify which element is required (although the elements may be of almost any type). Arrays may be fixed-length or expandable. • Record (also called tuple or struct) Records are among the simplest data structures. A record is a value that contains other values, typically in fixed number and sequence and typically indexed by names. The elements of records are usually called fields or members. • A hash or dictionary or map is a more flexible variation on a record, in which name-value pairs can be added and deleted freely. • Union. A union type definition will specify which of a number of permitted primitive types may be stored in its instances, e.g. "float or long integer". Contrast with a record, which could be defined to contain a float and an integer; whereas, in a union, there is only one value at a time. • A tagged union (also called a variant, variant record, discriminated union, or disjoint union) contains an additional field indicating its current type, for enhanced type safety. • A set is an abstract data structure that can store certain values, without any particular order, and no repeated values. Values themselves are not retrieved from sets, rather one tests a value for membership to obtain a boolean "in" or "not in". • An object contains a number of data fields, like a record, and also a number of program code fragments for accessing or modifying them. Data structures not containing code, like those above, are called plain old data structure. Many others are possible, but they tend to be further variations and compounds of the above. Basic principles Data structures are generally based on the ability of a computer to fetch and store data at any place in its memory, specified by an address—a bit string that can be itself stored in memory and manipulated by the program. Thus the record and array data structures are based on computing the addresses of data items with arithmetic operations; while the linked data structures are based on storing addresses of data items within the structure itself. Many data structures use both principles, sometimes combined in non-trivial ways (as in XOR linking) Data structure 2 The implementation of a data structure usually requires writing a set of procedures that create and manipulate instances of that structure. The efficiency of a data structure cannot be analyzed separately from those operations. This observation motivates the theoretical concept of an abstract data type, a data structure that is defined indirectly by the operations that may be performed on it, and the mathematical properties of those operations (including their space and time cost). Language support Most assembly languages and some low-level languages, such as BCPL, lack support for data structures. Many high-level programming languages, and some higher-level assembly languages, such as MASM, on the other hand, have special syntax or other built-in support for certain data structures, such as vectors (one-dimensional arrays) in the C language or multi-dimensional arrays in Pascal. Most programming languages feature some sorts of library mechanism that allows data structure implementations to be reused by different programs. Modern languages usually come with standard libraries that implement the most common data structures. Examples are the C++ Standard Template Library, the Java Collections Framework, and Microsoft's .NET Framework. Modern languages also generally support modular programming, the separation between the interface of a library module and its implementation. Some provide opaque data types that allow clients to hide implementation details. Object-oriented programming languages, such as C++, Java and .NET Framework use classes for this purpose. Many known data structures have concurrent versions that allow multiple computing threads to access the data structure simultaneously. References [1] Paul E. Black (ed.), entry for data structure in Dictionary of Algorithms and Data Structures. U.S. National Institute of Standards and Technology. 15 December 2004. Online version (http:/ / www. itl. nist. gov/ div897/ sqg/ dads/ HTML/ datastructur. html) Accessed May 21, 2009. [2] Entry data structure in the Encyclopædia Britannica (2009) Online entry (http:/ / www. britannica. com/ EBchecked/ topic/ 152190/ data-structure) accessed on May 21, 2009. Further readings • Peter Brass, Advanced Data Structures, Cambridge University Press, 2008. • Donald Knuth, The Art of Computer Programming, vol. 1. Addison-Wesley, 3rd edition, 1997. • Dinesh Mehta and Sartaj Sahni Handbook of Data Structures and Applications, Chapman and Hall/CRC Press, 2007. • Niklaus Wirth, Algorithms and Data Structures, Prentice Hall, 1985. External links • UC Berkeley video course on data structures (http:/ / academicearth. org/ courses/ data-structures) • Descriptions (http:/ / nist. gov/ dads/ ) from the Dictionary of Algorithms and Data Structures • CSE.unr.edu (http:/ / www. cse. unr. edu/ ~bebis/ CS308/ ) • Data structures course with animations (http:/ / www. cs. auckland. ac. nz/ software/ AlgAnim/ ds_ToC. html) • Data structure tutorials with animations (http:/ / courses. cs. vt. edu/ ~csonline/ DataStructures/ Lessons/ index. html) • An Examination of Data Structures from .NET perspective (http:/ / msdn. microsoft. com/ en-us/ library/ aa289148(VS. 71). aspx) • Schaffer, C. Data Structures and Algorithm Analysis (http:/ / people.
Details
-
File Typepdf
-
Upload Time-
-
Content LanguagesEnglish
-
Upload UserAnonymous/Not logged-in
-
File Pages587 Page
-
File Size-