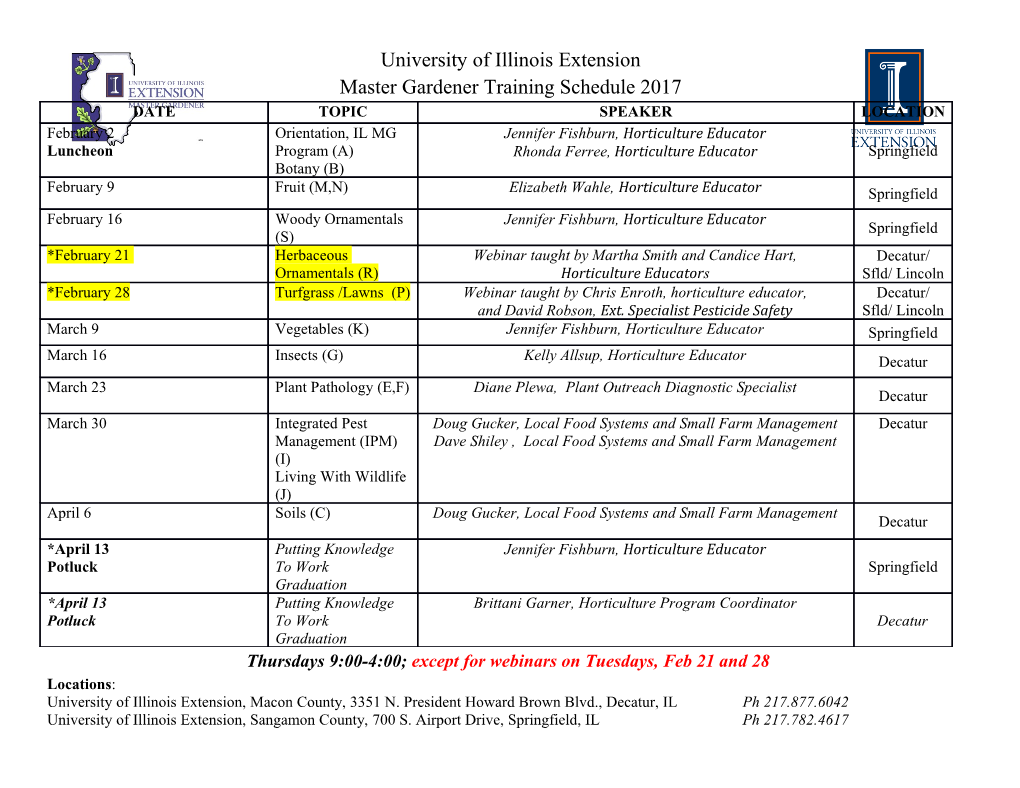
Identification of cell surface markers from multidimensional microarray data utilizing spherical Self Organizing Maps March 2016 Yuh Sugii The Graduate School of Natural Science and Technology (Doctor’s Course) OKAYAMA UNIVERSITY ABBREVIATIONS BSA: bovine serum albumin DMEM: Dulbecco’s modified Eagle’s medium EGFR: epithelial growth factor receptor FBS: fetal bovine serum FITC: fluorescein isothiocyanate GPI: glycosylphosphatidylinositol HEPES: 4-(2-HydroxyEthyl)-1-Piperazineethanesulfonic acid IP: ideal point MEM: minimum essential medium PBS: phosphate-buffered saline PCR: polymerase chain reaction qPCR: quantitative RT-PCR RFI: relative fluorescent intensity RPMI: Roswell Park Memorial Institute RT-PCR: reverse transcription polymerase chain reaction SDS: sodium dodecyl sulfate SSC: standard saline citrate sSOM: spherical Self-Organizing Map Table of contents GENERAL INTRODUCTION -------------------------------------------------- 1 CHAPTERⅠ ------------------------------------------------------------------------ 2 Identification of cell surface markers on glioma cells Abstract -------------------------------------------------------------------------------------------- 3 Introduction -------------------------------------------------------------------------------------- 4 Materials and Methods ------------------------------------------------------------------------ 5 Cell lines and culturing ---------------------------------------------------------------------- 5 RNA preparation ------------------------------------------------------------------------------ 5 Reverse transcription and qPCR ----------------------------------------------------------- 6 DNA microarray ------------------------------------------------------------------------------ 7 sSOM analysis --------------------------------------------------------------------------------- 7 Immunostaining ------------------------------------------------------------------------------- 8 Results and Discussion ------------------------------------------------------------------------- 9 Design of microarray for cell surface proteins ----------------------------------- 9 Cell surface markers commonly expressed in glioma -------------------------------- 10 Gene expression profiles on sphere surfaces ------------------------------------------- 11 Mining genes commonly expressed in glioma cell lines but not in brain tissues --------------------------------------------------------------------- 13 Evaluation of genes commonly expressed in glioma cell lines --------------------- 15 Conclusion --------------------------------------------------------------------------------------- 20 References ---------------------------------------------------------------------------------------- 20 CHAPTERⅡ ----------------------------------------------------------------------- 25 A spherical self-organizing map clustering of human breast cancer cell lines Abstract ------------------------------------------------------------------------------------------- 25 Introduction ------------------------------------------------------------------------------------- 26 Materials and Methods ----------------------------------------------------------------------- 27 Cell lines and cell culture ------------------------------------------------------------------- 27 Preparation of total RNA and cDNA synthesis ---------------------------------------- 27 Microarray analysis -------------------------------------------------------------------------- 28 Data filtering in breast cancer cell -------------------------------------------------------- 29 sSOM analysis of gene expression ------------------------------------------------------- 29 Results and discussion ------------------------------------------------------------------------ 30 sSOM clustering of human breast cancer cell lines ----------------------------------- 30 Conclusions -------------------------------------------------------------------------------------- 34 References ---------------------------------------------------------------------------------------- 35 ACKNOWLEDGEMENTS ----------------------------------------------------------------- 39 APPENDIX ----------------------------------------------------------------------------------------- 40 GENERAL INTRODUCTION The technology of high throughput screening is nowadays widely available in life science especially in the fields of molecular diagnosis and drug discovery. This is due to the establishment of microarray procedure, which deals with huge number of genes at one time. Cluster analysis is usually performed on the results of DNA microarray experiments. However, the routine procedure of data mining dealing with the huge number of signal information obtained from microarray is not fixed yet. We can find various way of clustering in hierarchical and non-hierarchical methods, which are applied for the analyses. The most popular ones appear non-hierarchical clustering such as k-means (MacQueen, 1965), partitioning around medoids (Kaufman and Rousseeuw, 1990) and cluster affinity search technique (Ben-Dor et al., 1999). We have employed spherical self-organizing map (sSOM), which is also a non-hierarchical clustering, to cluster genes by gene expression profiles of cells and tissues (Tuoya et al., 2008). Analyzing various types of carcinoma cells and normal tissues, we could find interesting cell surface molecules, which should serve as the molecular markers. This procedure, which we are demonstrating, is rather new to the data analyses of gene clustering from the gene expression profiles obtained from DNA microarray technique. Flexible arrangement of the data obtained allows us to cluster cells and tissues as well as genes to find definitely fantastic direction of further advancement of study. In the future this 1 procedure should be extremely helpful in the field of drug discovery as well as those of molecular biology and oncology. References Ben-Dor A, Shamir R, Yakhini Z. Clustering gene expression patterns. J Comput Biol; 1999:6(3-4)281-297. Kaufman L, Rousseeuw PJ. Finding Groups in Data: An Introduction to Cluster Analysis. John Wiley & Sons; 1990;ISBN:978-0-471-73578-6, New York. MacQueen J. Some Methods for Classification and Analysis of Multivariate Observations. In: Proceedings of the 5th Berkeley Symposium on Mathematical Statistics and Probability; 1965:281-297. Tuoya, Sugii Y, Satoh H, Yu D, Matsuura Y, Tokutaka H, Seno M. Spherical self-organizing map as a helpful tool to identify category-specific cell surface markers. Biochem Biophys Res Commun; 2008: 376(2)414-418. 2 CHAPTERⅠ Identification of cell surface markers on glioma cells Abstract To identify cell-specific markers, we designed a DNA microarray platform with oligonucleotide probes for human membrane-anchored proteins. Human glioma cell lines were analyzed using microarray and compared with normal and fetal brain tissues. For the microarray analysis, we employed a spherical self-organizing map, which is a clustering method suitable for the conversion of multidimensional data into two-dimensional data and displays the relationship on a spherical surface. Based on the gene expression profile, the cell surface characteristics were successfully mirrored onto the spherical surface, thereby distinguishing normal brain tissue from the disease model based on the strength of gene expression. The clustered glioma-specific genes were further analyzed by polymerase chain reaction procedure and immunocytochemical staining of glioma cells. Our platform and the following procedure were successfully demonstrated to categorize the genes coding for cell surface proteins that are specific to glioma cells. Our assessment demonstrates that a spherical self-organizing map is a valuable tool for distinguishing cell surface markers and can be employed in marker discovery studies for the treatment of cancer. 3 Introduction Currently, the high-throughput screening technology is widely available in the field of life science, especially in relation to molecular diagnosis and drug discovery. The versatility of the microarray technology enables the simultaneous analysis of a large number of genes at any given time. Cluster analysis is usually performed on the results of DNA microarray experiments. However, the routine procedure of data mining dealing with huge number of signals obtained from microarray has not yet been optimized. Various methods of hierarchical and non-hierarchical clustering have been employed in previous analyses. The most popular methods include non-hierarchical clustering such as k-means (MacQueen 1965), partitioning around medoids (Kaufman et al. 1990), and the cluster affinity search technique (Ben-Dor et al. 1999). We have employed a spherical self-organizing map (sSOM), which is also a non-hierarchical clustering method, to cluster genes by the gene expression profiles of cells and tissues (Ritter. 1999; Kihato et al. 2008; Tuoya et al. 2005, 2008; Abou-Sharieha et al. 2009; Sugii et al. 2011). In this study, we attempted to identify human glioma-specific cell surface markers by analyzing nine glioma-derived cell lines and compared normal adult and fetal brain tissues. Because the information relating to marker proteins on cells are extremely useful in identifying and targeting cells specifically, a sophisticated and conventional procedure to identify cell specific surface markers is highly desirable. 4 Our procedure proposed here is rather new and innovative in the analysis of data from gene clustering of the expression profiles obtained
Details
-
File Typepdf
-
Upload Time-
-
Content LanguagesEnglish
-
Upload UserAnonymous/Not logged-in
-
File Pages68 Page
-
File Size-