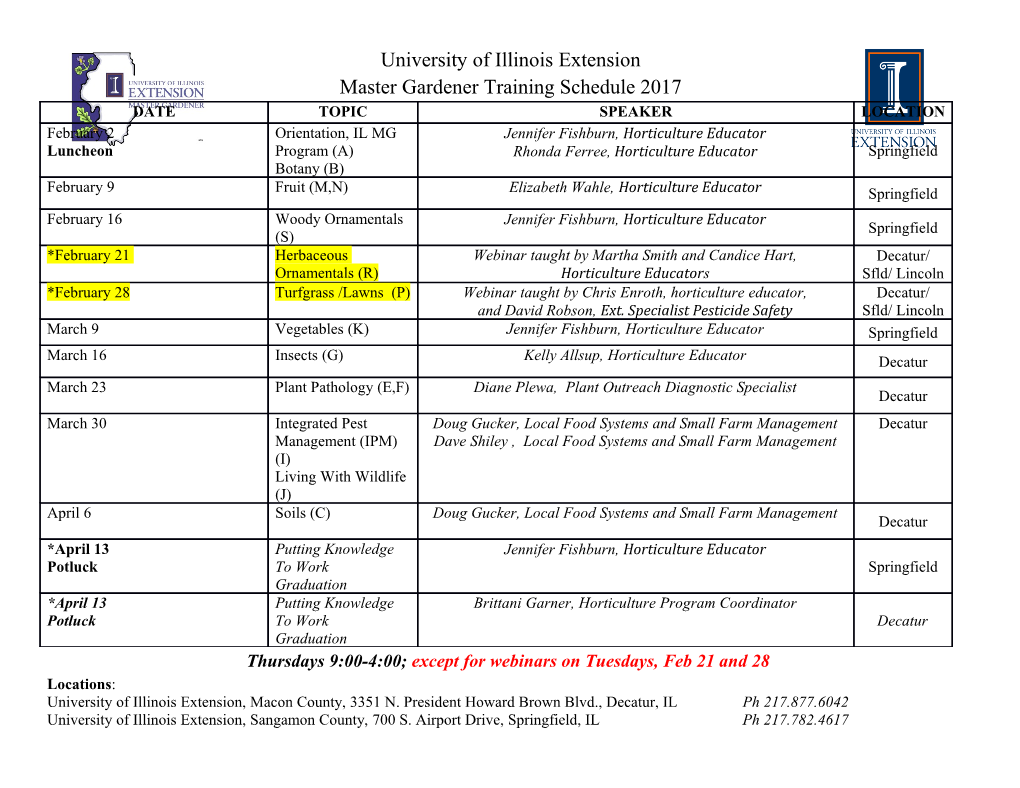
Institute of Mathematical Statistics LECTURE NOTES-MONOGRAPH SERIES The Likelihood Principle (Second Edition) James O. Berger Purdue University Robert L. Wolpert Duke University THE LIKELIHOOD PRINCIPLE: A REVIEW, GENERALIZATIONS, AND STATISTICAL IMPLICATIONS SECOND EDITION Institute of Mathematical Statistics LECTURE NOTES-MONOGRAPH SERIES Shanti S. Gupta, Series Editor Volume 6 The Likelihood Principle (Second Edition) James O. Berger Purdue University Robert L. Wolpert Duke University Institute of Mathematical Statistics Hayward, California Institute of Mathematical Statistics Lecture Notes-Monograph Series Series Editor, Shanti S. Gupta, Purdue University The production of the IMS Lecture Notes-Monograph Series is managed by the IMS Business Office: Nicholas P. Jewell, IMS Treasurer, and Jose L. Gonzalez, IMS Business Manager. Library of Congress Catalog Card Number: 88-81456 International Standard Book Number 0-940600-13-7 Copyright © 1988 Institute of Mathematical Statistics All rights reserved Printed in the United States of America To my parents, Orvis and Thelma James Berger To my wife, Ruta Robert Wolpert PREFACE This monograph began with research designed to provide a generali- zation of the Likelihood Principle (LP) to quite arbitrary statistical situations. The purpose of seeking such a generalization was to partially answer certain criticisms that had been levied against the LP, criticisms which seemed to prevent many statisticians from seriously considering the LP and its implications. The research effort seemed worthwhile because of the simplicity, central importance, and far reaching implications of the LP. Background reading for the research revealed a wider than expected range of published criticisms of the LP. In an attempt to be complete and address all such criticisms, the research paper expanded considerably. Eventually it seemed sensible to enlarge the paper to a monograph. This also allowed for discussion of conditioning ideas in general and for a review of the implications of the LP. It was decided, however, to stop short of a general review of conditional methods in statistics. In particular, the monograph does not discuss the many likelihood based statistical methodologies that have been developed, although references to these methodologies will be given. This limitation was, in part, because such an endeavor would be far too ambitious, and, in part, because we feel (and indeed argue in Chapter 5) that Bayesian implementation of the LP is the correct conditional methodology. The mathematical level of the monograph is, for the most part, kept at a nontechnical level. The main exception is the generalization of the LP in Section 3.4, which is (necessarily) presented at a measure-theoretic level, but can be skipped with no loss in continuity. Also, the monograph vii viii PREFACE presupposes no familiarity with conditioning concepts. Indeed Chapter 2 provides an elementary review of conditioning, with many examples. This second edition was produced under the rather severe constraint that the original manuscript, used for photo-offset printing, was inadvertantly destroyed; only the photos were kept. Thus changes could only be made by retyping entire pages or inserting new pages. A list of corrections that were too minor to justify the retyping of an entire page is given at the end of the monograph. Inserted pages received decimal page numbers: e.g. 74.1, 74.2. A list of additional references was added, and new discussions were kindly contri- buted by M. J. Bayarri and M. H. DeGroot, Bruce Hill, and Lucien Le Cam. Substantial changes or additions were made in Sections 3.1, 3.5, 4.2.1, 4.4, and 4.5. The changes in Section 4.4 correct a glaring oversight in the first edition: the failure to emphasize the misleading conclusions that can result from violation of the Likelihood Principle in significance testing of a precise hypothesis. Another very weak part of the first edition was Section 3.5, which discussed prediction, design, and nuisance parameters. The new material incorporates recent substantive insights from the literature. Numerous other minor changes and literature updatings were made throughout the monograph. We did not attempt complete coverage of recent lit- erature, however. We are grateful to a number of people for valuable discussions on this subject and/or for comments and suggestions on original drafts or the first edition of the monograph. In particular, we would like to thank George Barnard, M. J. Bayarri, Mark Berliner, Lawrence Brown, George Casella, Morris DeGroot, J. L. Foulley, Leon Gleser, Prem Goel, Clyde Hardin, Bruce Hill, Jiunn Hwang, Rajeev Karandikar, Lucien Le Cam, Ker-Chau Li, Dennis Lindley, George McCabe, Georges Monette, John Pratt, Don Rubin, Herman Rubin, Myra Samuels, Steve Samuels, and Tom Sellke. We are especially grateful to M. J. Bayarri and M. H. DeGroot for an exceptionally complete and insightful set of corrections and comments on the first edition. We are also grateful to Shanti Gupta for the encouragement to turn the material into a monograph. PREFACE ix Thanks are also due to the Alfred P. Sloan Foundation, the National Science Foundation (Grants MCS-7801737, MCS-8101670A1, and DMS-8702620), and the Center for Stochatic Processes at the University of North Carolina for support of the research in the monograph. Finally, we are extremely grateful to Norma Lucas, Teena Chase, and Betty Gick for excellent typing of the manuscript. March, 1988 JAMES 0. BERGER Purdue University, West Lafayette ROBERT WOLPERT Duke University, Durham TABLE OF CONTENTS CHAPTER 1. INTRODUCTION 1 CHAPTER 2. CONDITIONING 5 2.1. Simple Examples 5 2.2. Relevant Subsets 11 2.3. Ancillarity 13 2.4. Conditional Frequentist Procedures 14 2.4.1. Conditional Confidence 14 2.4.2. Estimated Confidence 16 2.5. Criticisms of Partial Conditioning 16 CHAPTER 3. THE LIKELIHOOD PRINCIPLE AND GENERALIZATIONS . 19 3.1. Introduction 19 3.2. History of the Likelihood Principle 22 3.3. Birnbaum's Development - the Discrete Case 24 3.3.1. EvidenceΛ Conditionally, and Sufficiency 24 3.3.2. Axiomatic Development 26 3.4. Generalizations Beyond the Discrete Case 28 3.4.1. Difficulties in the Nondiscrete Case 29 3.4.2. Evidencej Conditionality> and Sufficiency 31 3.4.3. The Relative Likelihood Principle 32 3.5. Prediction, Design, Nuisance Parameters, and the LP 36 3.5.1. Introduction 36 3.5.2. Unobserved Variables: Prediction and Design 37 3.5.3. Nuisance Variables and Parameters 41 x TABLE OF CONTENTS xi 3.6. Criticisms of Birnbaum's Axiomatic Development 42 3.6.1. The Model Assumption 43 3.6.2. The Evidence Assumption 45 3.6.3. The Weak Conditionality Principle 45 3.6.4. The Sufficiency Principle 46 3.7. Violation of the Likelihood Principle: Inadmissibility and Incoherency 50 3.7.1. Introduction 50 3.7.2. Decision Theoretic Evaluation 52 3.7.3. Betting Evaluation 59 CHAPTER 4. CONSEQUENCES AND CRITICISMS OF THE LIKELIHOOD PRINCIPLE AND THE RELATIVE LIKELIHOOD PRINCIPLE 65 4.1. Incompatibility with Frequentist Concepts 65 4.1.1. Introduction 65 4.1.2. Objectivity 67 4.1.3. Procedures for Nonspecialists 68 4.1.4. Repeatability 70 4.1.5. The Confidence Principle 71 4.2. The Irrelevance of Stopping Rules 74 4.2.1. Introduction 74 4.2.2. The (Discrete) Stopping Rule Principle 75 4.3.2. Positive Implications 77 4.2.4. Criticisms 80 4.2.5. Stopping Rules and Inadmissibility 83 4.2.6. The General Stopping Rule Principle 86 4.2.7. Informative Stopping Rules 88 4.3. The Irrelevance of Censoring Mechanisms 4.3.1. Introduction 90 4.3.2. Fixed Censoring and Equivalent Censoring Mechanisms ... 92 4.3.3. Random Censoring 95 4.3.4. Informative Censoring 103 xϋ TABLE OF CONTENTS 4.4. Significance Testing 104 4.4.1. Conflict with the Likelihood Principle 104 4.4.2. Averaging Over "More Extreme" Observations 105 4.4.3. Testing a Single Null Model 109 4.4.4. Conclusions 109.3 4.5. Randomization Analysis 110 4.5.1. Introduction 110 4.5.2. Finite Population Sampling 112 4.5.3. Randomization Tests 117 CHAPTER 5. IMPLEMENTATION OF THE LIKELIHOOD PRINCIPLE 121 5.1. Introduction 121 5.2. Non-Bayesian Likelihood Methods 122 5.3. Arguments for Bayesian Implementation 124 5.3.1. General Considerations 124 5.3.2. The Fraser-Monette-Ng_, Stone, and Stein Examples . 127 5.4. Robust Bayesian Analysis 136 5.5. Conclusions 141 REFERENCES 143 ADDITIONAL OR UPDATED REFERENCES 160 DISCUSSION BY M. J. BAYARRI AND M. H. DEGROOT 160.3 DISCUSSION BY BRUCE HILL 161 DISCUSSION BY DAVID LANE 175 DISCUSSION BY LUCIEN LE CAM 182 REPLY TO THE DISCUSSION 186 ADDITIONAL REFERENCES IN THE DISCUSSION 198 INDEX OF EXAMPLES 200 AUTHOR INDEX 201 SUBJECT INDEX 204 ERRATA AND CLARIFICATIONS 207 CHAPTER 1. INTRODUCTION Among all prescriptions for statistical behavior, the Likelihood Principle (LP) stands out as the simplest and yet most farreaching. It essen- tially states that all evidence, which is obtained from an experiment, about an unknown quantity θ, is contained in the likelihood function of θ for the given data. The implications of this are profound, since most non-Bayesian approaches to statistics and indeed most standard statistical measures of evidence (such as coverage probability, error probabilities, significance level, frequentist risk, etc.) are then contraindicated. The LP was always implicit in the Bayesian approach to statistics, but its development as a separate statistical principle was due in large part to ideas of R. A. Fisher and G. Barnard (see Section 3.2 for references). It received major notice when Birnbaum (1962a) showed it to be a consequence of the more commonly trusted Sufficiency Principle (that a sufficient statistic summarizes the evidence from an experiment) and Conditionality Principle (that experiments not actually performed should be irrelevant to conclusions). Since then the LP has been extensively debated by statisticians interested in founda- tions, but has been ignored by most statisticians.
Details
-
File Typepdf
-
Upload Time-
-
Content LanguagesEnglish
-
Upload UserAnonymous/Not logged-in
-
File Pages252 Page
-
File Size-