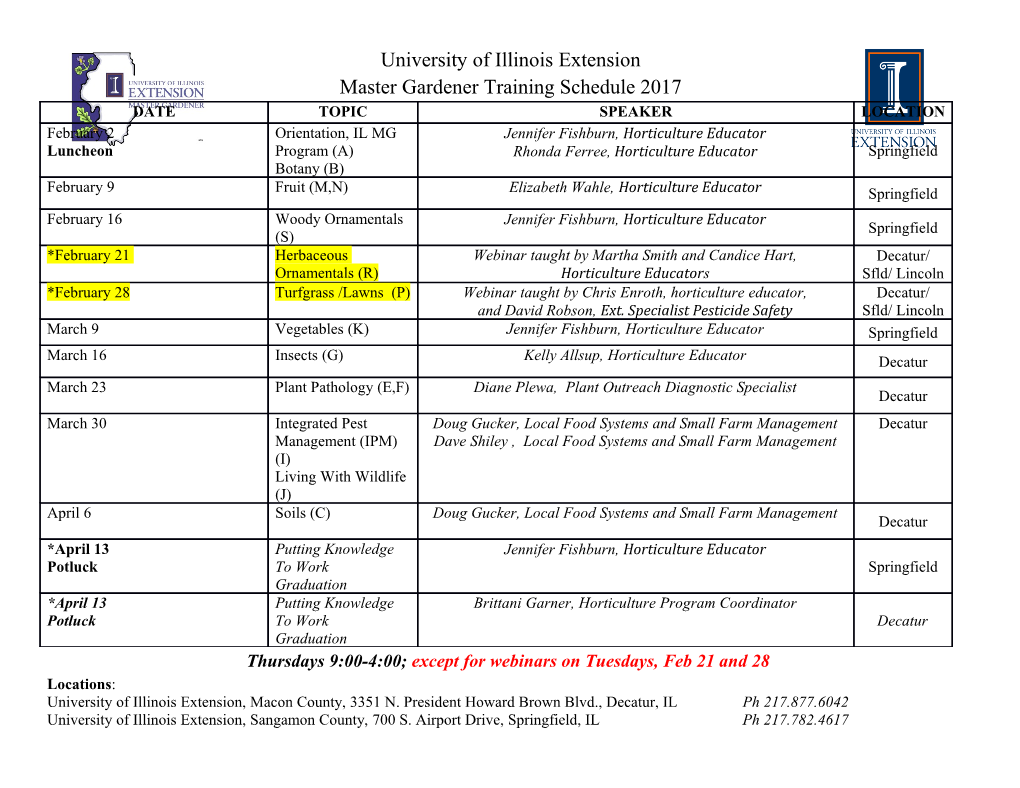
International Conference on Intelligent Computational Systems (ICICS'2012) Jan. 7-8, 2012 Dubai Artificial Neural Network (ANN) Approach for an Intelligent System: A Case Study in Carnatic Classical Music (CCM) Prof. Dr. P. K. Srimani and Y. G. Parimala crucial for the purpose. Abstract—ANNs are invaluable in cognitive studies of Particular care has been taken to set up the same. In intelligent, knowledge- based systems. The immense features addition a sensitivity analysis of the input data has been of ANN makes it very attractive for a wide range of made to study the effects of variation in the various applications. In the present paper a unique ANN approach for inputs on the expected output values. The experimental studying cognitive aspects of CCM has been undertaken for a typical case of ‘tonic- shifting’ CCM uses a heptatonic scale of results are presented and discussed. seven notes – S,R,G,M,P,D, N. Variants in notes result in 72 heptatonic scales.‘Tonic-shift’.is a phenomenon where staying II. ARTIFICIAL NEURAL NETWORKS AND in the domain of an original scale, new scales are obtained by COGNITION shifting the base pitch from S to other notes of the original ANNs have massive parallelism, high connectivity, raga. This was studied using Multilayer percepteron(MLP) and linear regression(LR), varying number of hidden layers, which tries to emulate the Biological neurons and their processing conditions, learning algorithms. Input data was synapses. NNs are especially useful for classification frequencies in each heptatonic scale before and after tonic and function approximation/mapping problems. The shifting. 100% accurate results were obtained. Sensitivity networks use Input/Out put layers and none or few analysis was performed to study effects of inputs on results. hidden layers (HLs). Every hidden and output unit has its own bias term Learning algorithms can be supervised Keywords— Cognitive, Heptatonic, Multi-layer or unsupervised. In supervised learning, the correct Percepteron, Tonic shift. results (desired outputs) are known and are given to the NN during training so that the NN can adjust its weights I. INTRODUCTION to try match its outputs to the target values. After OR the first time in literature, ANN approach has training, NN is tested by giving only input values, to see F been used to study the tonic-shift(TS) phenomenon how closely it predicts target values. LM, Momentum in CCM. Some of the previous pioneering work on TS Step are common learning algorithms. Performances of are found in some papers [11]-[15]. Recently, “Pattern different network topologies can be compared by recognition and unique Raga classification and evaluating the error function. Various networks are identification using artificial network” was studied by trained by minimization of an appropriate error function the authors[15]. Scales(ragas) in CCM are theoretically defined with respect to a training data set. Performance infinite and the complex phenomenon of TS needs of networks are compared by evaluating the error extensive research. Hence a new dimension of approach function using an independent cross- validation(CV) set. has been considered using ANN which throws new light Network having smallest error with respect to CV set is on both the concerned case study as well as the selected (hold out method). Performance of the selected performances of different networks in such studies. network is confirmed using a third independent set of ANN can have various architectures, which require data called a test set to avoid overfitting [1]-[5]. different types of algorithms [1], [2]. In the present paper we have chosen MLP and LR networks. The A. Multilayer Percepteron )MLP) cases of one or two hidden layers, using batch and MLP has one or more HLs with a linear combination online processing, and Levenberg-Marquardt (LM) or function is the inner product of inputs,weights and a momentum algorithms have been investigated in detail. bias. Activation function is logistic or tanh function. Proper setting of input data and expected results is very Inputs are fully connected to the first HL, each HL is fully connected to the next, and the last HL is fully Prof. Dr. P. K. Srimani, F.N.A.Sc is with R&D (CS), Bangalore, connected to the outputs. MLPs use supervised learning INDIA; As Director; Y. G. Parimala, M.E.(Canada), (Ph.D) is with City Engg College, or backpropagation. Designing and training an MLP VTU, Bangalore, INDIA Phone:9341212972, (e-mail: requires i) selecting number of HLs, ii) number of [email protected]). 101 International Conference on Intelligent Computational Systems (ICICS'2012) Jan. 7-8, 2012 Dubai neurons to be used in each HL, iii)avoiding local ascending order of frequencies. It is not equitempered minima, iv)converging to an optimal solution in a scale like the Western, since notes have varying ratios reasonable period of time, v)validating NN to test for with their successive notes. Two notes S and P are overfitting. MLPs are used in classification problems, inherently stable with no variants. The remaining 5 in fitness approximations[3],[4]. notes R,G,M,D,N have variants. M has 2 variants -M1 and M2. They result in 12 semitones as shown in B. Linear-Regression TABLE I. These details have been discussed by the Linear regression assumes that expected value of the authors in previous papers [13]-[15] output given an input, is linear. BAYES rule is used to Delineation of the 7 notes in a particular flow, with S obtain posterior distribution for given data. Maximum- as the base pitch gives the melodious expressions called likelihood estimation helps in predictions [3]-[5]. RAGA, which depends on creativity and proficiency of the musician. In CCM a full scale (heptatonic) [9] –[15] III. TONIC-SHIFT-PROPERTY consists of all the 7 notes in both ascending and CCM is a highly scientific and evolved form of descending called a ‘melakartha’(‘Janaka’) raga if i)all classical music which uses a heptatonic scale of seven the 7 notes in ascending and descending are identical notes symbolically given as S,R,G,M,P,D,N in TABLE I RELATIVE FREQUENCIES OF THE 12 SEMITONES [6]-[8],[15] S R1 R2 G2 G3 M1 M2 P D1 D2 N2 N3 1 256/243 9/8 192/162 81/64 4/3 729/512 3/2 128/81 27/16 16/9 243/128 ii)frequencies occur in order. Exactly 72 such scales shifted to every note present in a given scale. The new are possible. They are given characteristic names and scales generated were verified to evaluate if a new valid are serially arranged in a table. This is found extensively raga was obtained. Since there are 72 scales, TS to in literature and in previous papers of the authors[6]-[8], remaining 6 notes of each scale theoretically gives 432 [13]-[15]. Beauty of a raga delineation takes a new combinations. But only 122 of these are valid scales. dimension when performer sings or plays another raga Hence a total of 194 exemplars were used as inputs. by staying in the domain of the original raga. This is 100% accurate results were obtained with MLP and LR possible by temporarily shifting the reference note to a models,using LM and momentum learning, 2 HLs. new note existing in the original raga. Hence all the Sensitivity analysis was performed to study the effects of remaining semitones will take different relative values the inputs on target values. MLP used here was hetero- leading to a new raga. This is called ‘tonic-shift’. Every associative, supervised learning since correct results such shift may not give a new raga. For eg 29th (desired outputs) were known, so that during training the melakartha raga called ‘DhiraShankarabharana’. has NN could adjust its weights to match its outputs to the scale S, R2,G3,M1,P,D2, N3., i.e., relative target values. After training, NN was tested giving only frequencies are 1 9/8 81/64 4/3 3/2 27/16 and 243/128. input values LR method allows user to test a network on Shifting reference from S to R2 makes the frequency a chosen data set Best network weights were used to ratios of R2, G3, M1,P,D2,N3 and upper octave S,=1, minimise CV error. 9/8 192/162 4/3 3/2 27/16 and 16/9 respectively. This corresponds to another new raga (the 22nd raga ‘Kharaharapriya’). Continuing the process of shifting to various notes give raise to 4 other ragas using G3, M1, P and D2 as reference, [11]. When reference is shifted to N3, relative frequencies give rise to both M1 and M2 in the new scale which does not correspond to any valid scale. Fig 1 Multilayer Percepteron (MLP) model IV. FORMULATION OF THE PROBLEM V. EXPERIMENTS AND RESULTS CCM being highly scientific, shows very interesting phenomena which are intriguing researchers from a The present paper concerns with the study of an long time. One such phenomenon known as modal tonic intelligent system to analyse the performance of MLP shifting exhibited by ragas of CCM have been and LR for a classification and regression problem. A investigated in the present paper using ANN. MLP and case study was taken up to study the unique LR models were constructed using inputs of frequencies phenomenon of tonic shifts in CCM. Input data present in all possible heptatonic ragas of CCM. consisted of relative frequency ratios of the notes in Applying TS on them, frequencies of the base pitch was heptatonic scales and their TS. MLP neural network was constructed with one and two HLs and studied for 102 International Conference on Intelligent Computational Systems (ICICS'2012) Jan.
Details
-
File Typepdf
-
Upload Time-
-
Content LanguagesEnglish
-
Upload UserAnonymous/Not logged-in
-
File Pages5 Page
-
File Size-