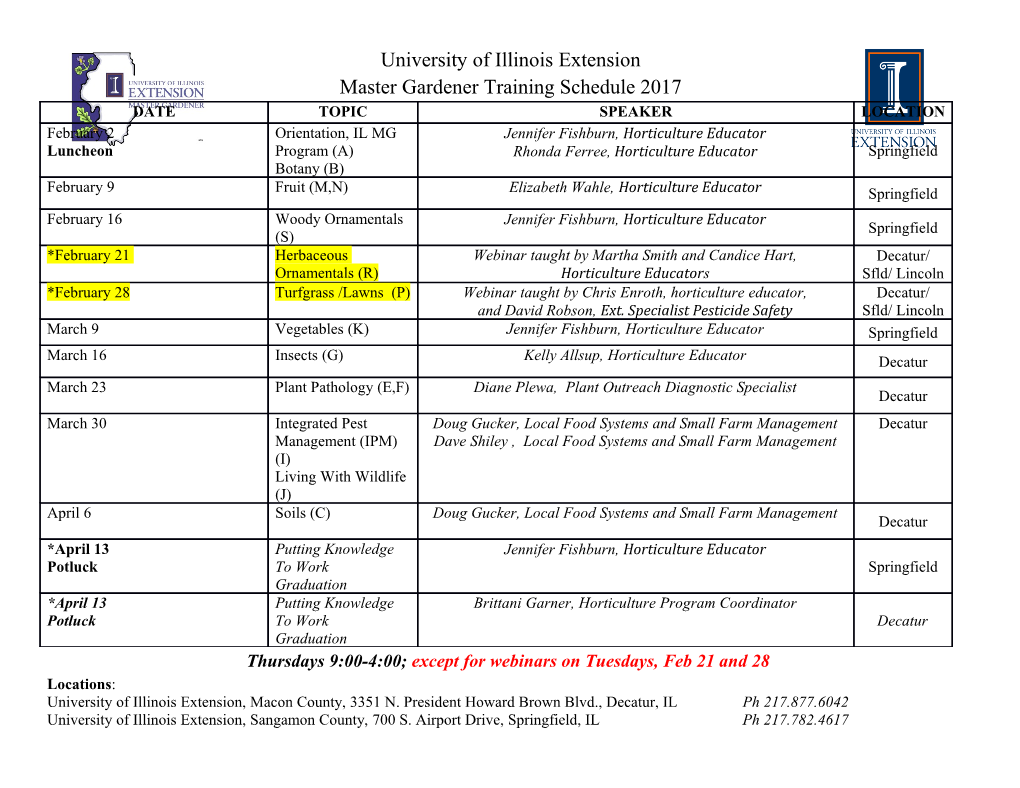
Biometrika (2011), 98,4,pp. 979–985 doi: 10.1093/biomet/asr057 C 2011 Biometrika Trust Printed in Great Britain Miscellanea False discovery rate for scanning statistics BY D. O. SIEGMUND, N. R. ZHANG Department of Statistics, Stanford University, 390 Serra Mall, Stanford, California 94305-4065, U.S.A. [email protected] [email protected] Downloaded from AND B. YAKIR Department of Statistics, The Hebrew University of Jerusalem, Jerusalem 91905, Israel [email protected] http://biomet.oxfordjournals.org/ SUMMARY The false discovery rate is a criterion for controlling Type I error in simultaneous testing of multi- ple hypotheses. For scanning statistics, due to local dependence, clusters of neighbouring hypotheses are likely to be rejected together. In such situations, it is more intuitive and informative to group neighbouring rejections together and count them as a single discovery, with the false discovery rate defined as the pro- portion of clusters that are falsely declared among all declared clusters. Assuming that the number of false discoveries, under this broader definition of a discovery, is approximately Poisson and independent of the number of true discoveries, we examine approaches for estimating and controlling the false discovery rate, and provide examples from biological applications. at University of Pennsylvania Library on May 30, 2013 Some key words: False discovery rate; Multiple comparisons; Poisson approximation; Scan statistic. 1. INTRODUCTION In a pioneering paper, Benjamini & Hochberg (1995) initiated a fruitful line of research into the false discovery rate as a method to evaluate Type I error when simultaneously testing large numbers of hypothe- ses. We use their notation, so R is the number of discoveries that emerge as a result of a particular statistical procedure, and V is the number of false discoveries among them. Then S = R − V is the num- ber of true discoveries. The false discovery rate is the expected relative proportion of false discoveries, FDR = E(V/R; R > 0). These quantities are defined implicitly in terms of the specific procedure that is used to make discoveries. We are concerned with estimation and control of false discovery rates when there is substantial local correlation among the statistics used for testing the hypotheses. Due to local correlation, large values of the statistic tend to occur in clumps, and multiple rejections within a clump may constitute only a single discovery, as it relates to model identification. Yet a possibly large number of correct rejections at some locations can inflate the denominator in the definition of false discovery rate, hence artificially creating a small false discovery rate, and lowering the barrier to possibly false detections at distant locations. Scanning statistics to detect sparsely distributed signals provide typical examples. In the examples that follow, there is an underlying set of observations yt , where t varies over an indexing set having some geometric structure. The yt are often assumed to be independent, but this is not necessary, providing the dependence between them is local with respect to the geometric structure. The test statistics {Zt : t ∈ D}, where Zt is a function of t and of the ys for s ∈ Nt , an appropriate neighbourhood of t, are related by a measure of distance within the scanning index set D. Hence, values of Zt and Zs for nearby t and s in D 980 D. O. SIEGMUND,N.R.ZHANG AND B. YAKIR are correlated, so a large value at a specific τ ∈ D causes a cluster of large values at t close to τ.Thus,a group of large values of Zt within close proximity are often associated with a single signal. Example 1. The random fields to detect local activity in an fMRI scan as discussed in a series of papers by Worsley, for example, Worsley et al. (1992)orSiegmund & Worsley (1995). Example 2. Massively parallel paired end DNA re-sequencing used to detect structural variation in genomic sequences. For a review, see Medvedev et al. (2009). The data come in the form of distances yt between mapped positions of relatively short paired reads from the ends of DNA sequences of approxi- mately w base pairs in length, with the leftmost read mapped to position xt in the genome. Where there are no structural variations one observes, after subtracting w and standardizing, yt that are independent and have a standard normal distribution. For read pairs that straddle the breakpoint τ of a structural variation, the distribution of some percentage of the yt ,forτ<xt τ + w, is shifted by an unknown amount δ, which is related to the size of the variant. The score statistic with respect to δ to test for a breakpoint at τ is Downloaded from | τ+w |/w1/2 τ τ+1 yt . A scan is conducted with varying over the genomic region of interest to find putative breakpoint locations. Example 3. The scan statistics of variable window width used in Zhang et al. (2010) and Siegmund et al. 2011 to detect common regions of copy number variation in a set of subjects. An appropriate likeli- http://biomet.oxfordjournals.org/ hood based statistic for the special case of a single sequence (Olshen et al., 2004) is similar to that of the preceding example, but the window width is unknown, so the scan involves two-dimensional maximization with respect to τ and w. Example 4. A genome scan to detect either linkage or association between a phenotype and related genetic variation, e.g., Lander & Botstein (1989), Siegmund & Yakir (2007). The main results of this paper are methods for estimating and controlling the false discovery rate of a given procedure, with discovery defined in the sense of detection of sparse, local signals. In order to focus on the conceptual aspect of how one defines a discovery, our assumptions are given in general, at University of Pennsylvania Library on May 30, 2013 abstract terms, and we avoid, except for a few comments, the necessarily technical and application spe- cific discussion of methods to ensure and test the validity of those assumptions. Motivated by a different genomic application, Zhang (2008) contains a similar approach without any theoretical analysis. In an unpublished 2011 manuscript in the Harvard University Biostatistics Working Paper Series A. Schwartz- man, Y. Gavrilov and R. J. Adler discuss a different approach to the same general issue under specific technical assumptions motivated by and apparently limited to a one-dimensional process having the struc- ture of Example 1. Our two central assumptions are (i) the distribution of the number of false discoveries, V , is Poisson, with expected value λ; and (ii) the number of false discoveries is independent of the number of true discov- eries, S. The number of true discoveries must be nonnegative, but otherwise may follow any distribution. The Poisson assumption is valid asymptotically in a variety of applications. These include the examples given above under the assumptions made in the cited references, or more generally if one makes suitable adjustments when there are local dependencies in the underlying observations. See Aldous (1988)for numerous examples, Lindgren et al. (1983) for relevant general theorems under different sets of condi- tions, and Arratia et al. (1989) for a flexible Poisson approximation theorem that applies quite generally to processes involving local dependence. Methods for determining λ depend on the specific problem. Illustrative examples based on more explicit assumptions about the underlying process are discussed below. The assumption of independence between V and S is more subtle. If we treat the locations of the signals in D as fixed but unknown quantities, then D can be partitioned into disjoint sets D0 ∪ D1, where D0 are hypotheses that, if rejected, would be considered part of a false discovery, and D1 are hypotheses that, if rejected, would be considered part of a true discovery. For example, in the simple case of a one-dimensional scan with fixed window size w as in Example 2, suppose the true signals are a set of intervals I within the scan region. If we count those windows that overlap with any interval in I towards true discoveries, and the rest towards false discoveries, then D0 ={t : (t, t + w] ∩ ι =∅,ι∈ I} and D1 = D \ D0. Then, V Miscellanea 981 would be a function solely of {Zt : t ∈ D0}, while S would be a function solely of {Zt : t ∈ D1}. At least when the true signals are sparse, approximate independence between V and S would follow if long-range dependencies between {Zt : t ∈ D0} and {Zt : t ∈ D1} are negligible. In practice, near overlap of detected signals is a danger sign regarding possible violation of this hypothesis of independence. For a more detailed discussion, see § 3·1. Significant long range dependence between {Zt } may cause nonnegligible dependence between V and S. Scanning procedures that are based on a collection of localized tests are inherently designed for problems where dependence can be assumed to be local, since if long range independence does not hold, then procedures that account for that dependence would be preferred on the basis of greater power. The estimator that we propose for the false discovery rate is FDRˆ = λ/(R + 1), (1) where λ is the expected number of false discoveries and R is the total number of discoveries. This esti- Downloaded from mator has been considered by Efron (2010) in the framework of hypothesis testing with a large number of independent hypotheses and, except for the constant 1 in the denominator, is the same as that suggested by Zhang (2008). In some cases, the parameter λ can be derived analytically. In other cases it, can be computed via permutations or simulations conducted under the null assumption. In § 2, we show that the estimator (1) is unbiased under assumptions (i) and (ii).
Details
-
File Typepdf
-
Upload Time-
-
Content LanguagesEnglish
-
Upload UserAnonymous/Not logged-in
-
File Pages8 Page
-
File Size-