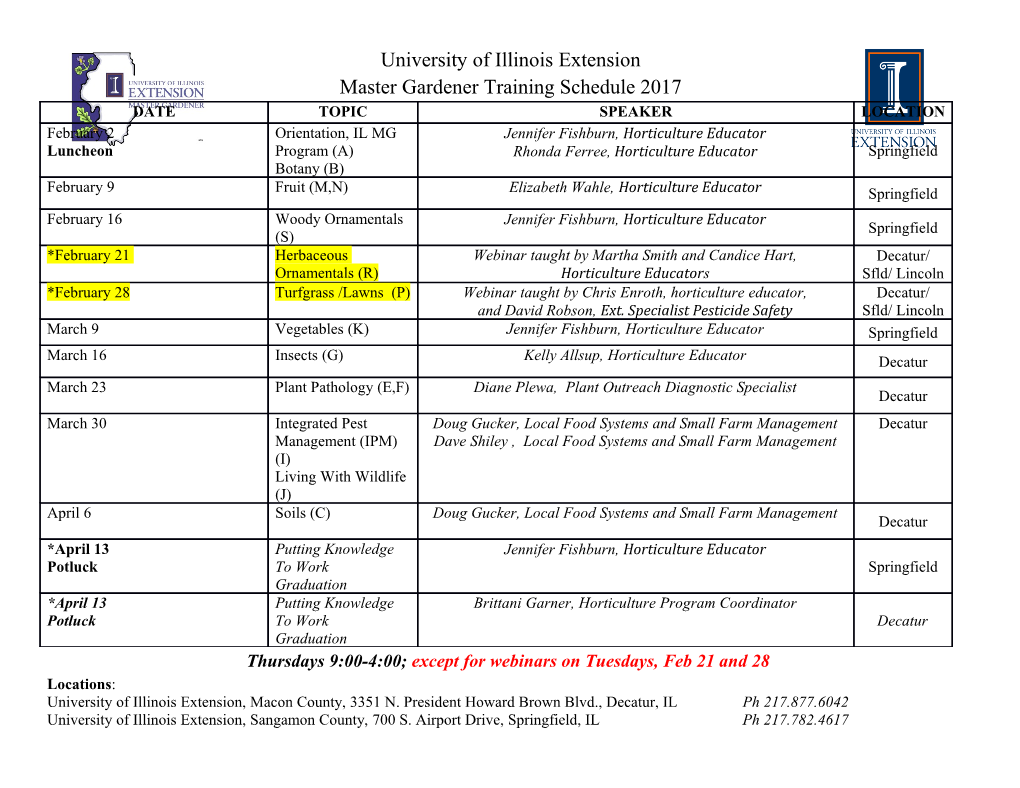
Modeling and Searching for Non-Coding RNA W.L. Ruzzo http://www.cs.washington.edu/homes/ruzzo Outline • Why RNA? • Examples of RNA biology • Computational Challenges – Modeling – Search – Inference The “Central Dogma” DNA RNA Protein gene Protein DNA (chromosome) RNA (messenger) cell “Classical” RNAs • mRNA • tRNA • rRNA • snRNA (small nuclear - spli cing) • snoRNA (small nucleolar - guides for t/rRNA modifications) • RNAseP (tRNA maturation; ribozyme in bacteria) • SRP (signal recognition particle; co-translational targeting of proteins to membranes) • telomerases Non-coding RNA • Messenger RNA - codes for proteins • Non-coding RNA - all the rest – Before, say, mid 1990’s, 1-2 dozen known (critically important, but narrow roles: e.g. tRNA) • Since mid 90’s dramatic discoveries – Regulation, transport, stability/degradation – E.g. “microRNA”: ≈ 100’s in humans • By some estimates, ncRNA >> mRNA RNA Secondary Structure: RNA makes helices too U C A A Base pairs G C C G A A U G C U A C G C G A U G C A A CA AU RNA on the Rise • In humans – more RNA- than DNA-binding proteins? – much more conserved DNA than coding – MUCH more transcribed DNA than coding • In bacteria – regulation of MANY genes involves RNA – dozens of classes & thousands of new examples in just last 5 years Human Predictions • Evofold – S Pedersen, G Bejerano, A Siepel, K Rosenbloom, K Lindblad-Toh, ES Lander, J Kent, W Miller, D Haussler, "Identification and classification of conserved RNA secondary structures in the human genome." PLoS Comput. Biol., 2, #4 (2006) e33. – 48,479 candidates (~70% FDR?) • RNAz – S Washietl, IL Hofacker, M Lukasser, A H殳 tenhofer, PF Stadler, "Mapping of conserved RNA secondary structures predicts thousands of functional noncoding RNAs in the human genome." Nat. Biotechnol., 23, #11 (2005) 1383- 90. – 30,000 structured RNA elements – 1,000 conserved across all vertebrates. – ~1/3 in introns of known genes, ~1/6 in UTRs – ~1/2 located far from any known gene • FOLDALIGN – E Torarinsson, M Sawera, JH Havgaard, M Fredholm, J Gorodkin, "Thousands of corresponding human and mouse genomic regions unalignable in primary sequence contain common RNA structure." Genome Res., 16, #7 (2006) 885-9. – 1800 candidates from 36970 (of 100,000) pairs • CMfinder – unpublished – 6500 candidates in ENCODE alone (again high FDR) Fastest Human Gene? Origin of Life? Life needs information carrier: DNA molecular machines, like enzymes: Protein making proteins needs DNA + RNA + proteins making (duplicating) DNA needs proteins Horrible circularities! How could it have arisen in an abiotic environment? Origin of Life? RNA can carry information too (RNA double helix) RNA can form complex structures RNA enzymes exist (ribozymes) The “RNA world” hypothesis: 1st life was RNA-based ncRNA Example: Xist • large (12kb?) • unstructured RNA • required for X-inactivation in mammals ncRNA Example: 6S • medium size (175nt) • structured • highly expressed in e. coli in certain growth conditions • sequenced in 1971; function unknown for 30 years 6S mimics an open promoter E.coli Barrick et al. RNA 2005 Trotochaud et al. NSMB 2005 Willkomm et al. NAR 2005 ncRNA Example: IRE Iron Response Element: a short conserved stem- loop, bound by iron response proteins (IRPs). Found in UTRs of various mRNAs whose products are involved in iron metabolism. E.g., the mRNA of ferritin (an iron storage protein) contains one IRE in its 5' UTR. When iron concentration is low, IRPs bind the ferritin mRNA IRE. repressing translation. Binding of multiple IREs in the 3' and 5' UTRs of the transferrin receptor (involved in iron acquisition) leads to increased mRNA stability. These two activities form the basis of iron homeostasis in the vertebrate cell. Iron Response Element IRE (partial seed alignment): Hom.sap. GUUCCUGCUUCAACAGUGUUUGGAUGGAAC Hom.sap. UUUCUUC.UUCAACAGUGUUUGGAUGGAAC Hom.sap. UUUCCUGUUUCAACAGUGCUUGGA.GGAAC Hom.sap. UUUAUC..AGUGACAGAGUUCACU.AUAAA Hom.sap. UCUCUUGCUUCAACAGUGUUUGGAUGGAAC Hom.sap. AUUAUC..GGGAACAGUGUUUCCC.AUAAU Hom.sap. UCUUGC..UUCAACAGUGUUUGGACGGAAG Hom.sap. UGUAUC..GGAGACAGUGAUCUCC.AUAUG Hom.sap. AUUAUC..GGAAGCAGUGCCUUCC.AUAAU Cav.por. UCUCCUGCUUCAACAGUGCUUGGACGGAGC Mus.mus. UAUAUC..GGAGACAGUGAUCUCC.AUAUG Mus.mus. UUUCCUGCUUCAACAGUGCUUGAACGGAAC Mus.mus. GUACUUGCUUCAACAGUGUUUGAACGGAAC Rat.nor. UAUAUC..GGAGACAGUGACCUCC.AUAUG Rat.nor. UAUCUUGCUUCAACAGUGUUUGGACGGAAC SS_cons <<<<<...<<<<<......>>>>>.>>>>> ncRNA Example: MicroRNAs • short (~22 nt) unstructured RNAs excised from ~75nt precursor hairpin • approx antisense to mRNA targets, often in 3’ UTR • regulate gene activity, e.g. by destabilizing (plants) or otherwise suppressing (animals) message • several hundred, w/ perhaps thousands of targets, are known ncRNA Example: T-boxes ncRNA Example: Riboswitches • UTR structure that directly senses/binds small molecules & regulates mRNA • widespread in prokaryotes • some in eukaryotes Gene Regulation: The MET Repressor SAM Protein Alberts, et al, 3e. DNA . e 3 The , l a t e protein , s t r e b way l A Riboswitch alternatives (2 of 4 shown) Corbino et al., Genome Biol. 2005 Example: Glycine Regulation • How is glycine level regulated? • Plausible answer: g gce protein g g g TF g TF glycine cleavage enzyme gene DNA transcription factors (proteins) bind to DNA to turn nearby genes on or off The Glycine Riboswitch • Actual answer (in many bacteria): gce protein g g g g 5′ 3′ gce mRNA glycine cleavage enzyme gene DNA Mandal et al. Science 2004 Why? • RNA’s fold, and function • Nature uses what works Impact of RNA homology search (Barrick, et al., 2004) glycine riboswitch operon B. subtilis L. innocua A. tumefaciens V. cholera M. tuberculosis (and 19 more species) Impact of RNA homology search (Barrick, et al., 2004) Using our glycine techniques, we riboswitch operon found… B. subtilis L. innocua A. tumefaciens V. cholera M. tuberculosis (and 19 more species) (and 42 more species) RNA Informatics • RNA: Not just a messenger anymore – Dramatic discoveries – Hundreds of families (besides classics like tRNA, rRNA, snRNA…) – Widespread, important roles • Computational tools important – Discovery, characterization, annotation – BUT: slow, inaccurate, demanding Q: What’s so hard? A A G A A A A A A U G C G U U C U C A C G C G C U C U G A G G C A A G G G G U A G C C G G A G C U C G C C A A G A G G G A A G G A G A A G G A C C C A C U G U A C U C C C G A A A A A GG C G U C A A C A A A U A A G A G A C G G A U U A C U C U U U C U C U G G G U U C G C G A C G G G U G C C G A G A G C U U C G A A A U U G U A C C G U U G U G U A G G C G A: Structure often more important than sequence Computational Challenges • Search - given • CM-based search related RNA’s, find more • Modeling - describe • Hand-curated a related family alignments -> CMs • Meta-modeling - • Covariance Models what’s a good modeling framework? Predict Structure from Multiple Sequences … GA … UC … Compensatory … GA … UC … mutations reveal … GA … UC … structure, but in … CA … UG … usual alignment algorithms they are … CC … GG … doubly penalized. … UA … UA … How to model an RNA “Motif”? • Conceptually, start with a profile HMM: – from a multiple alignment, estimate nucleotide/ insert/delete preferences for each position – given a new seq, estimate likelihood that it could be generated by the model, & align it to the model mostly G del ins all G How to model an RNA “Motif”? • Covariance Models (aka “profile SCFG”) – Probabilistic models, like profile HMMs, but adding “column pairs” and pair emission probabilities for base-paired regions <<<<<<< >>>>>>> … p a ire d c o lu m n s … “RNA sequence analysis using covariance models” Eddy & Durbin Nucleic Acids Research, 1994 vol 22 #11, 2079-2088 What • A probabilistic model for RNA families – The “Covariance Model” – ≈ A Stochastic Context-Free Grammar – A generalization of a profile HMM • Algorithms for Training – From aligned or unaligned sequences – Automates “comparative analysis” – Complements Nusinov/Zucker RNA folding • Algorithms for searching Main Results • Very accurate search for tRNA – (Precursor to tRNAscanSE - current favorite) • Given sufficient data, model construction comparable to, but not quite as good as, human experts • Some quantitative info on importance of pseudoknots and other tertiary features Probabilistic Model Search • As with HMMs, given a sequence, you calculate llikelihood ratio that the model could generate the sequence, vs a background model • You set a score threshold • Anything above threshold => a “hit” • Scoring: – “Forward” / “Inside” algorithm - sum over all paths – Viterbi approximation - find single best path (Bonus: alignment & structure prediction) Example: searching for tRNAs Alignment Quality Comparison to TRNASCAN • Fichant & Burks - best heuristic then a i t r – 97.5% true positive n e t e i r – 0.37 false positives per MB r e c f f i n d • CM A1415 (trained on trusted alignment) o i t y l a – > 99.98% true positives t u h l g a – <0.2 false positives per MB i l v • Current method-of-choice is “tRNAscanSE”, a CM- S e based scan with heuristic pre-filtering (including TRNASCAN?) for performance reasons. CM Structure • A: Sequence + structure • B: the CM “guide tree” • C: probabilities of letters/ pairs & of indels • Think of each branch being an HMM emitting both sides of a helix (but 3’ side emitted in reverse order) Overall CM Architecture • One box (“node”) per node of guide tree • BEG/MATL/INS/DEL just like an HMM • MATP & BIF are the key additions: MATP emits pairs of symbols, modeling base-pairs; BIF allows multiple helices CM Viterbi Alignment th xi = i letter of input xij = substring i,..., j of input Tyz = P(transition y " z) ! E y P(emission of x ,x from state y) xi ,x j = i j y Sij = max# logP(xij generated starting in state y via path #) ! Viterbi, cont.
Details
-
File Typepdf
-
Upload Time-
-
Content LanguagesEnglish
-
Upload UserAnonymous/Not logged-in
-
File Pages121 Page
-
File Size-