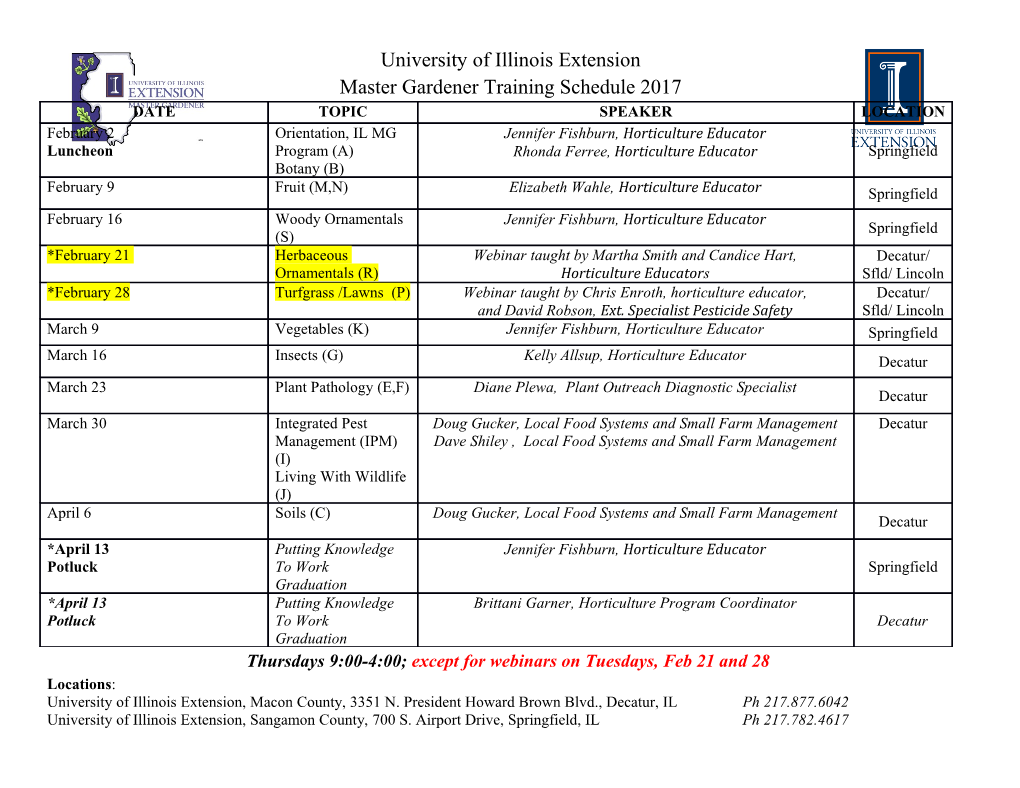
Available online at www.sciencedirect.com ScienceDirect A process-oriented approach to respecting privacy in the context $ of mobile phone tracking Gabriella M Harari Mobile phone tracking poses challenges to individual privacy governmental organizations, corporations). Consider the because a phone’s sensor data and metadata logs can reveal mobile phone — a ubiquitous behavioral tracking tech- behavioral, contextual, and psychological information about nology carried by an estimated five billion people around the individual who uses the phone. Here, I argue for a process- the world [1], with ownership at high rates in advanced oriented approach to respecting individual privacy in the economies and growing steadily in emerging economies context of mobile phone tracking by treating informed consent [2]. As part of their core functionality, mobile phones can as a process, not a mouse click. This process-oriented collect information about who a person communicates approach allows individuals to exercise their privacy with and how often (via call and SMS logs) and where a preferences and requires the design of self-tracking systems person spends their time (via GPS and WiFi data). Other that facilitate transparency, opt-in default settings, and information about what a person spends their time doing individual control over personal data, especially with regard to: (via app logs) can also be collected because these devices (1) what kinds of personal data are being collected and (2) how mediate much of our everyday activity (e.g. browsing, the data are being used and shared. In sum, I argue for the navigating, shopping, banking, information searching). development of self-tracking systems that put individual user privacy and control at their core, while enabling people to Technological advancements continue to push the harness their personal data for self-insight and behavior boundaries of what mobile phones can track about human change. This approach to mobile phone privacy is a radical behavior — at a personal [3] and societal level [4]. The departure from current standard data practices and has immense potential for using mobile phone data to under- implications for a wide range of stakeholders, including stand human behavior has been heralded across a range of individual users, researchers, and corporations. scientific disciplines, including psychology [5 ,6], psy- chiatry [7], medicine [8], communication [9], sociology Address [10], and computer science [11]. However, mobile phones Department of Communication, Stanford University, Stanford, CA also introduce unique privacy challenges for developers 94305, United States who create mobile phone tracking systems, for academic Corresponding author: Harari, Gabriella M ([email protected]) and corporate stakeholders who collect and model mobile phone data, and for the billions of people around the world who own a mobile phone [12]. These privacy Current Opinion in Psychology 2020, 31:141–147 challenges stem from the depth and temporal scale of This review comes from a themed issue on Privacy and disclosure, the devices’ sensing capabilities, the richness of their online and in social interactions sensors and metadata logs, and the tendency for devices Edited by Leslie k John, Michael Slepian, and Diana Tamir to be physically present with their owners much of the For a complete overview see the Issue and the Editorial time [13 ]. Available online 20th September 2019 To outline a few of the privacy challenges in this context, https://doi.org/10.1016/j.copsyc.2019.09.007 I start my review by describing how passive sensing 2352-250X/ã 2019 Published by Elsevier Ltd. technologies and mobile phones can be used to directly collect or indirectly infer personal information, focusing on information about people’s behaviors, contexts, and psychological characteristics. Next, I outline some of the privacy concerns people may experience in the context of mobile phone tracking, operationalizing privacy as the Introduction ability to control information about the self [14] and In many societies today, information about human behav- assuming privacy to be a universal human right [15]. I ior is routinely tracked (i.e. collected and modelled) by then provide my view on how we can begin to address mobile phones, wearables, smart home devices and the some of the privacy challenges by adopting a process- various stakeholders who own and operate such sensing oriented approach to obtaining informed consent to technologies (e.g. individual users, researchers, mobile phone data tracking. In particular, I argue that $ My thanks go to Ruth Appel, Sam Gosling, Sandra Matz, Sandrine Mu¨ ller, Ramona Schoedel, Clemens Stachl, Sanaz Talaifar, and Sumer Vaid for helpful feedback on earlier drafts of this manuscript and for many discussions about the privacy implications of mobile phone tracking. www.sciencedirect.com Current Opinion in Psychology 2020, 31:141–147 142 Privacy and disclosure, online and in social interactions privacy preferences can be respected in the context of which refers to ‘any kind of log or sensor data that directly mobile phone tracking by designing self-tracking systems describes an individual’ (pg. 452; [18]). Much of this per- that facilitate transparency, opt-in default settings, and sonal data is typically generated and stored as part of the individual control over personal data throughout the standard functioning of the device (e.g., text message logs tracking process. Specifically, I describe a need for facili- arestoredonthedevicesothe usercanaccessthedataatany tating transparency with regard to: (1) what kinds of time). However, these personal data can also be collected personal data are being collected from the phone, (2) by device manufacturers and by third-party applications how the mobile phone data are being used and shared. that people download to their device, which run as a This approach to mobile phone privacy is a radical depar- background process on the device to passively collect ture from current standard practices and has implications sensor data and metadata logs and/or make inferences for a wide range of stakeholders, including individual based on the sensing data. For example, many popular users, researchers, and corporations. By adopting these gaming and social media apps routinely collect information approaches to transparency, stakeholders involved in about a device’s location via GPS data, even when knowing mobile phone tracking can respect individual privacy a person’s location is not central to the service provided by rights and provide individuals with a means of harnessing the app.In research settings, specially designed apps can be their personal data for self-insight and behavior change. used to obtain behavioral and contextual information about participants during a study [5 ]. Sensing technologies can directly collect and indirectly infer personal information Sensing data can reveal personal information about an Passive sensing refers to the unobtrusive collection and individual’s behaviors, situational contexts, and psycholog- modeling of sensor data and metadata generated by ical characteristics (for more detailed reviews on this topic, sensing technologies, such as mobile phones, wearables, see Refs. [19 ,20]). Generally, personal information can be smart cars, and smart home appliances. In mobile phones obtained from sensing data in two ways, personal informa- today, the sensor hardware that comes embedded in a tion may be: (1) directly collected (e.g. latitude and longi- typical device includes accelerometers, microphones, tude coordinates collected using the GPS showing the Global Positioning Systems (GPS), Bluetooth, and WiFi device’s precise location), and/or (2) indirectly inferred scanners [16]. Metadata from mobile phones include (e.g. inferring contextual information about where a person timestamped system logs that contain a record of infor- is, such as being at home or work). When considering mation about how a device is used [17], such as call and privacy challenges and possible solutions, this distinction SMS logs, application use logs, and battery logs. Figure 1 is important because people may be generally aware of the provides an illustration of some of the common types of types of data that are being collected by applications on their mobile sensors and metadata that can be collected from device (e.g. an application on their phone having access to mobile phones. their location via GPS), but may not be aware of other types of personal information that can be inferred about them Sensing technologies have enabled the continuous and using their personal data (e.g. their routine travel patterns, unobtrusive collection of vast amounts of personal data, places they spend time in). Figure 1 Sources of Personal Data in Mobile Phones Mobile Sensors Metadata Accelerometer Call Logs Microphone SMS Logs Bluetooth App Use Logs Ambient Light Screen State Logs GPS Battery Logs Current Opinion in Psychology Summary of commonly collected sources of personal data from mobile phones. Current Opinion in Psychology 2020, 31:141–147 www.sciencedirect.com Privacy in the context of mobile phone tracking Harari 143 Sensing personal information from mobile phone data a given situation; [20]) can be collected and inferred from The personal information that can be directly collected and smartphone data. Location information can be inferred indirectly inferred from mobile phone data make respect- from GPS data to identify a person’s place of residence
Details
-
File Typepdf
-
Upload Time-
-
Content LanguagesEnglish
-
Upload UserAnonymous/Not logged-in
-
File Pages7 Page
-
File Size-