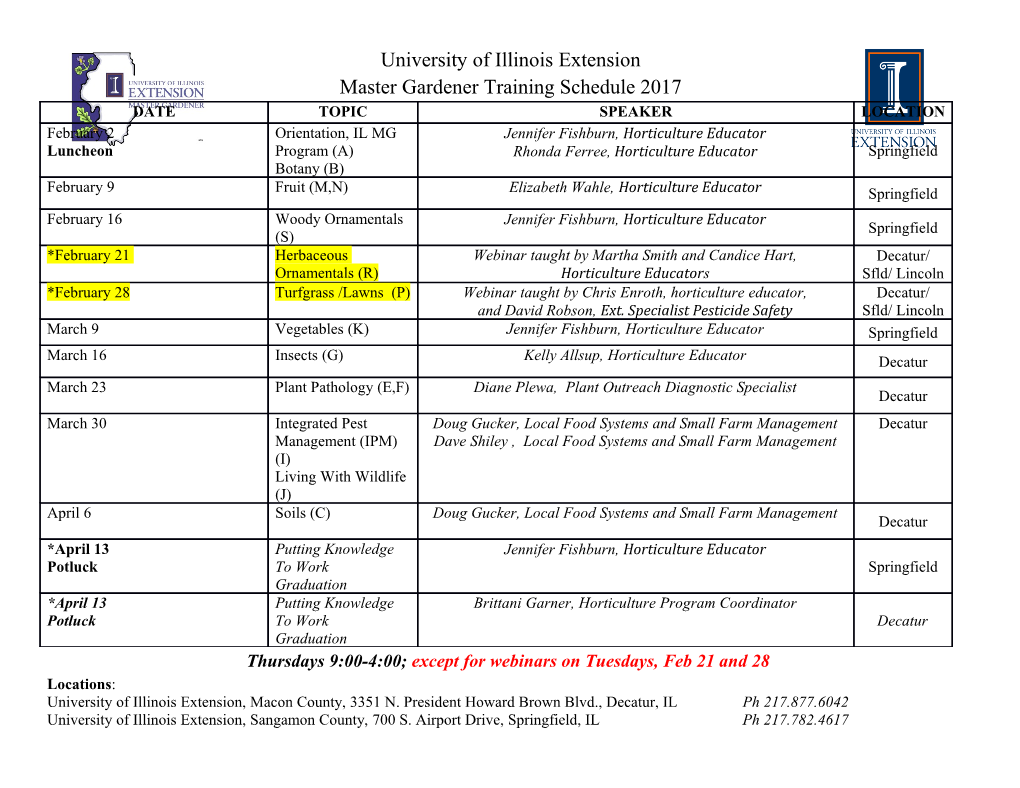
.... ... ... ... .. ........ .. ....... ... The EXIST HET Imaging Technical Working Group .. .... .. .. Gerry Skinner (working group leader) NASA- GSFC / CRESST/ Univ Md Scott Barthelmy NASA-GSFC • : ' Computing challenges in Steve Sturner NASA-GSFC / CRESST/ Univ Md .: • Coded Mask Imaging .: Mark Finger NASA-MSFC / NSSTC Huntsville Garrett Jernigan Univ California, Berkeley .... Gerry Skinner ' . ' • ' JaeSub Hong Harvard-Smithsonian CfA Brandon Allen Harvard-Smithsonian CfA . .. ... .. .. Josh Grindlay (Exist PI) Harvard-Smithsonian CfA G.K.Skinner High Performace Computing i n Observational Astronomy, IUCAA, Pune, Oct 2009 •1 What is a coded mask The Coded Mask Technique telescope? is the worst possible way of making a telescope open/opaque Ô i l Õ Except when you can Õt do anything better ! Each detector pixel records the sum of the ¥ Wide fields of view signals from a different combination of incident ¥ Energies too high for focussing, or too low directions or Ôsky pixels’ for Compton/Tracking detector techniques (plus background) Very good angular resolution ¥ Spatially resolving detector, physically or logically divided into Ô pixelsÕ 1) The simplist Õs approach to weighing 3 objects 2) Weighing of 3 objects by a member of the Club of the Difficult Approach A B A B A C ac wa t wb t wab t w t t 0.85 8 C B C A= ( wab+wac- w bc )/2 t 0.85 8 B= ( wab-wac+w bc )/2 t 0.85 C= (-wab+wac-w bc)/2 wc t wbc t Mask In general:- Patterns N objects (unknowns) - Hexagonal or rectangular N measures of selected combinations - Cylclic or non-cyclic Uncertainty reduced by factor ~ N 1/2/2 - Random, URA, MURA, É Only works because we have supposed that the uncertainty Construction is independent of the quantity being measured - the equivalent of a Low energies (e.g. 10-20 keV) background limited observation - etched metal foil (often gold-plated to increase absorbtion) - usually self supporting (grid or bars connect isolated elements) - Additional ‘ Spider’ or supporting bars part of the mask pattern High energies (e.g. 1 MeV) Blocks of Tungsten a few cm thick - can have a ‘ substrate’ (e.g. Carbon fibre honeycomb) Position sensitive detectors for coded mask telescopes Detector Technology Approx Examples Notes Energy Range (keV) CCD 0.5-10 HETE-2 SXC 1-d 33 arc sec resolution ! 6as-filled Proportional 2-50 Spacelab-2 Space Shuttle Counter TTM, Mir-Kvant Space Station SAX-WFC RXTE ASM 1-d HETE-2 WXM 1-d Integral : JEM-X Arrays of semiconductor 5-100 Legri (CZT) MiniSat-01 detectors Integral : ISRI (CdTe) Anger Camera 50-1000 Sigma On 6ranat Exite-2 Balloon Array of Scintillator 100-10000 New Hampshire D6T Balloon detectors Integral : PIXIT Energy: 20-8000 keV SPI 770 mm dia Aray of 6ermanium 20-10000 SA6E Balloon 3 cm thick Tungsten detectors Integral : SPI 60 mm pitch Resolution ~ 2.5¡ Point Source The Point Source Response Function Response 3 Function Blurring can always be removed by image processing But 1) Deblurring is always done at the expense of noise tR ',If 2) For a coded mask telescope, every point in the image is affected by the noise from the whole A coded mask telescope has the worst PSF imaginable detector plane The response to a point source isn’t just ‘a bit blurred’, it fills the whole detector plane ! r ’Optimum coded ’ designs How to recover an image or ’URAs’ Basic method : Ô Correlation with the Mask PatternÕ (Uniformly Redundant Arrays) ^'- Certain patterns have the properties: ^i i) Their DISCRETE, CYCLIC Recorded pattern is Convolution of source distribution 1I I autocorrelation function is indeed a Delta function, and the mask pattern, plus some background B r PLUS A FLAT LEVEL. D = S M + B 1) ii) For uniform background, M B is not zero, but it is Suppose we form an image as t at least FLAT. I = M D = M S M + MB = M M S + MB If you can: Arrange that coding is cyclic = ACF(M) S + M B Use Binned (discrete) arrays where ACF indicated the Autocorrellation function. Be prepared to subtract a DC level If ACF(M) were a Delta function and if M B were zero Then this is just what is needed ’Optimum coded ’ designs or ’URAs’ Some aspects of real systems ¥ Non cyclic ¥ Mask Closed element absorbtion Mask ¥ Mask Open element transparency ¥ Mask Element Thickness ¥ Obstructions in Mask Plane ¥ Detector finite position resolution ¥ Detector efficiency non-uniformities Detector ¥ Detector response dependent on off-axis angle ¥ Detector background non-uniform ¥ Gaps in the detector plane ¥ Dead/inactive pixels in the detector plane Other ¥ Shielding (collimation) imperfect ¥ Obstructions between detector and mask ¥ Leaks onto detector from far outside the fov Correlation with the Mask Pattern used with Real (Imperfect) Coded Mask Telescopes Correlation methods are often used even for real, imperfect, non-optimum, systems based. ' It is can be fast, taking advantage of Fast Fourier Transforms ' It always gives some sort of an image, even for non-ideal systems ' For a single point source it yields the best possible sensitivity (smallest uncertainty on the intensity estimate) Detected count rate versus source / detector-pixel transmission factor ce . Strong Sour ^^ Weak Source c UO N O 0 0.5 P 1 i1 Peak height in Correlation map Slope of line Source strength Intercept Background per pixel Data points with P ij ~ 0.5 (Õ grey` mask elements) are of little value in defining the slope Patching-up the Correlation approach The realities lead to PSF ' Ghosts/Sidelobes Handle non-cyclic systems by extending arrays Substitute estimates based on means for missing data (simple approach to reconstruction) Correct for background variations i Correct for sensitivity variations etc, etc ' Additional noise Y (more sophisticated reconstruction) ' Interpixel correlations J Iterative removal of sources (IROS) Assume all sources are pointlike Fortunately coded mask telescopes are not very sensitive ! Identify the brightest one using a correlation map Fit the source position and subtract the data predicted for that source taking into account all the effects in the REAL system ' 100 detection, 5% ghosts - important Form a correlation map from the residuals ' 5 detection , 10% ghosts - who cares? Coded Mask Telescopes - Matrix Approach Matrix approach - the case of M=N One wants to obtain the intensity of the sky in each of M ‘pixels’ ; number of unknowns = number of measurements S , S , S , S , ... S e 1 2 3 M-1, S , One measures N linear combinations of the j 1 Starting from Di= h S (i=0,N-1) G o j [D ] = [H ][S ] Objective - given the D, deduce the S If M= N, in principle, it Õs easy. Using matrices we can write one can obtain the intensities S of the sky pixels utilising the inverse matrix 1 [ S ] = [H ] [D] D h h ... h S 0 00 0 0 0 h D, = 0 S, ¥ For an imaginary (ideal, URA/optimum) cyclic system, this is (almost) D h h S , N , N -,,0 N-, _ exactly equivalent to the correlation method [D ] = [H ][S ] • For a real system, in principle it allows you to get rid of ALL ghosts/sidelobes. Matrix approach - 1st problem: M>N Matrix approach 2 nd problem: Noise Amplification i.e. fewer measurements than Sky pixels Starting from This is the usual situation if you make a simple staring observation [D ] = [H] [S] (More sky pixels than independent detector positions + unknowns associated with detector background) one can obtain the intensities S of the sky pixels utilising = theinversem S [HT• [ An under-determined problem D] Solution - fit different masks and make an observation through each BUT, in fact 05 }btpins . "+UF. p with uncertainties added y or (more practicable) make more observations with offset pointing directions where [n] is JSJ16 Ô1ji"I CQo"1ntRje has a OVER determined problem a Use Moore-Penrose Generalised Inverse and if there are large values in H-1 the noise can become enormous Minimising Noise Amplification Other approaches to image reconstruction ¥ In good signal-to-noise data a (generalised) inverse matrix approach ¥ Maximum Entropy allows for all instrumental effects and removes ghosts Allows all instrumental effects to be taken - but it adds noise into account and finds image which is consistent ¥ In low signal-to-noise cases, a matrix method equivalent to the correlation with the data which has no information which is approach minimises the effects of noise not Ô required Õ by the data but it adds noise Iterative - each iteration uses a correlation to find ¥ Minimum Error Matrix Methods how image should be modified provide the optimum compromise between the two ¥ Back Projection If all exposure and coding efficiency effects are taken int account Equivalent to correlation methods Fast for few The scale of the problem Extracting Spectra SPI-like IBIS-like Measurements So far haven Õt considered spectra Detector pixels per pointing 19 1 100 104 1 104 Pointings 25 200 1 25 6 Can divide events into Ô pulse height Õ bins and do Total N 475 105 105 2.5 10 Unknowns all of the above for each bin Sky pixels 400 500 2 104 2 104 Backrounds 19 500 10 10 Total M 419 1000 2 104 2 104 Or identify sources, then solve best fit intensity in Number of operations ME Matrix Approach each pulse height bin 4 16 21 To invert matrix (brute force) N 6.1010 2 10 17 10 4 10 To invert matrix (iterative) M N 2 108 4 10 11 2 1012 10 15 9 In either case, end up with a spectrum in a new To multiply by the matrix M.N 105 5 108 2 108 5 10 observation space.
Details
-
File Typepdf
-
Upload Time-
-
Content LanguagesEnglish
-
Upload UserAnonymous/Not logged-in
-
File Pages14 Page
-
File Size-