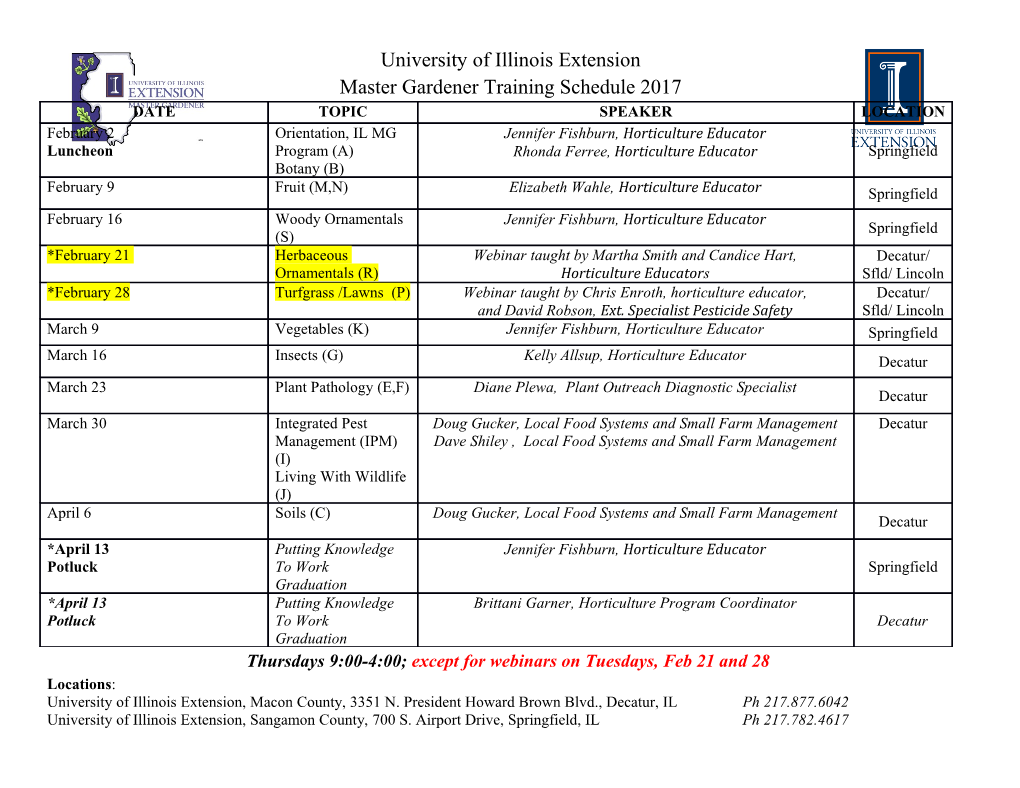
manager Editor: Donald J. Reifer I Reifer Consultants [email protected] Safe and Simple Software Cost Analysis Barry Boehm “Everything should be as simple as pos- COCOMO II Productivity Ranges sible, but no simpler.” – Albert Einstein Figure 1 shows the relative productivity ranges (PRs) of the major COCOMO II imple software cost-analysis meth- cost-driver factors, as compared to those in ods are readily available, but they the original COCOMO 81 model. A fac- aren’t always safe. The simplest tor’s productivity range is the ratio of the method is to base your cost estimate project’s productivity for the best possible on the typical costs or productivity factor rating to its worst possible factor rat- S rates of your previous projects. That ing, assuming that the ratings for all other approach will work well if your factors remain constant. Here, we define rel- new project doesn’t have any ative “productivity” in either source lines of cost-critical differences from code (SLOCs) or function points per person- those previous projects. But it month. The term “part” in Figure 1 reflects won’t be safe if some critical the fact that the COCOMO 81 development cost driver has degraded. mode involved a combination of develop- Simple history-based soft- ment flexibility and precedentedness. ware cost-analysis methods For example, suppose your business soft- would be safer if you could ware group has been developing similar ap- identify which cost driver fac- plications for 10 years on mainframe com- tors were likely to cause critical puters and their next application is to run cost differences and estimate on a micro-based client-server platform. In how much cost difference would result if a this case, the only cost-driver factor likely to critical cost driver changed by a given de- cause significant cost differences is the gree. In this column, I’ll provide a safe and group’s platform experience (assuming that simple method for doing both of these by such factors as platform volatility and use of using some recently published cost estimat- software tools do not change much). Figure ing relationships (Software Cost Estima- 1 shows that the productivity range for the tion with COCOMO II, by Barry Boehm platform experience factor is 1.40. Thus, et al., Prentice Hall, 2000). COCOMO II is changing from the best level of platform ex- an updated and recalibrated version of the perience (over 6 years) to the worst level Constructive Cost Model (COCOMO) (less than 2 months) will increase the originally published in Software Engineer- amount of effort required for the project by ing Economics (by Barry Boehm, Prentice a factor of 1.40, or by 40%. Hall, 1981). I’ll also show how the CO- If we compare the productivity ranges for COMO II cost drivers let you perform cost platform experience between COCOMO II sensitivity and trade-off analyses, and dis- and COCOMO 81, we see that they are cuss how you can use similar methods with fairly close (1.40 versus 1.34). That’s true for other software cost-estimation models. most of the cost-driver factors. In particular, 14 IEEE SOFTWARE September/October 2000 0740-7459/00/$10.00 © 2000 IEEE MANAGER personnel and team capability Figure 1. Comparing CO- remains the single strongest Software productivity range COMO 81 and COCOMO II. *Development flexibility 1.26 influence on a software pro- *Development mode (part) 1.32 (*Varies by size; see ject’s productivity. Its produc- *Team cohesion 1.29 COCOMO II Table 1. Values for 100 Developed for reuse 1.31 COCOMO 81 tivity range in COCOMO II is *Precedentedness 1.33 KSLOC products.) somewhat smaller than in Development mode (part) 1.32 *Architecture & risk resolution 1.39 COCOMO 81 (3.53 versus Platform experience 1.40 4.18), but that might be be- 1.34 but it will improve produc- Data base size 1.42 cause COCOMO II has added 1.23 tivity by 71% on a 1,000- two more people-related fac- Required development schedule 1.43 KSLOC project, mostly by re- 1.23 tors (team cohesion and per- Language & tools experience 1.43 ducing rework. These ranges sonnel continuity), which Language experience 1.20 probably under-estimate the *Process maturity 1.43 might account for some of the Modern programming practices 1.51 effect of high maturity levels, variance previously associated Storage constraint 1.46 as they normalize out the ef- 1.56 with personnel capability. Platform volatility 1.49 fects of other productivity fac- COCOMO II has some ad- 1.49 tors such as the use of software Use of software tools 1.50 ditional new cost drivers be- 1.49 tools and reusable software sides team cohesion and per- Applications experience 1.51 components. Details are avail- 1.57 sonnel continuity: Personnel continuity 1.51 able in Bradford Clark’s USC Documentation match to life cycle needs 1.52 PhD dissertation, “The Effects I Multisite development 1.53 software developed for reuse; Turnaround time 1.32 of Software Process Maturity I architecture and risk reso- Required software reliability 1.54 on Software Development Ef- 1.87 lution; Time constraint 1.63 fort,” USC-CSE-TR-97-510, I software process maturity 1.66 available via the USC-CSE Product complexity 2.38 (somewhat replacing use 2.36 COCOMO II Web site (see of modern programming Personnel/team capability 3.53 the “Leading Software Cost- practices); 4.18 Estimation Tools” box), under I documentation match to 02.00 4.00 “Related Research.” life cycle needs; and I multisite development (somewhat they enter the COCOMO II estima- Safe and Simple Software Cost Analysis replacing turnaround time). tion formula as exponential func- To return to our platform experi- tions of size rather than as effort ence example, you might find that Each of these new factors has a sta- multipliers. The productivity ranges you can do better than a cost increase tistically significant greater-than-1.0 shown in Figure 1 for these size-sen- of 40% if you can find a few people productivity range in the multiple re- sitive variables are for a product with some microprocessor-based gression analysis of the 161 projects whose size is 100,000 source lines of client-server platform experience for in the COCOMO II database. The code (100 KSLOC). Table 1 shows your project. For each cost-driver one exception was the “developed how the productivity ranges for these factor, COCOMO II provides rating for reuse” factor, which had insuffi- factors vary by size. scales and tables showing how pro- cient dispersion in its rating levels to Thus, for example, the effect of ductivity will vary for each rating produce a statistically significant re- varying a project’s process maturity level. Table 2 shows the resulting ef- sult. We determined its productivity from a low Level 1 to a Level 5 on the fort multipliers by rating scale for range of 1.31 (and the productivity SEI Capability Maturity Model scale each of the personnel-experience cost ranges of the other COCOMO II fac- will typically improve productivity by factors in COCOMO II. tors) using a Bayesian weighted aver- only 20% on a 10-KSLOC project, These tables will let you perform age of data-determined multiple re- gression results and expert-deter- mined Delphi results. When the Table 1 data-determined values are weakly Size-Dependent Productivity Ranges determined, the weighted average will give a higher weight to the ex- Size (KSLOC) pert-determined values. (See Chapter Factor 10 100 1,000 4 of the COCOMO II book for a de- Development flexibility 1.12 1.26 1.42 tailed discussion.) Team cohesion 1.13 1.29 1.46 Some factors in Figure 1 have an Precedentedness 1.15 1.33 1.53 asterisk, indicating that their produc- Architecture and risk resolution 1.18 1.39 1.63 tivity ranges vary by size, because Process maturity 1.20 1.43 1.71 September/October 2000 IEEE SOFTWARE 15 MANAGER Simple Sensitivity and Trade-off Analysis Table 2 You can extend this approach to COCOMO II Effort Multipliers Versus Length of Experience cover sensitivity and trade-off analy- Average length of experience sis among several cost-driver factors. Type of experience < 2 mo 6 mo 1 year 3 years > 6 years PR For example, suppose you also had With platform 1.19 1.09 1.00 0.91 0.85 1.40 an Option B to add a couple of ex- With languages and tools 1.20 1.09 1.00 0.91 0.84 1.43 tremely experienced client-server de- With application area 1.22 1.10 1.00 0.88 0.81 1.51 velopers, increasing your average platform experience to 1 year. How- ever, they have very little business ap- plications experience, decreasing Table 3 your average applications experience from > 6 years to 3 years. In this The Effect of Adding Experienced Client-Server Developers case, Table 3 shows the comparison Effort multipliers to the previous Option A. platform x application = product This tables shows that Option A Options Platform Application Product Person-months and B are roughly equivalent in effort A 1.09 0.81 0.88 51 and cost (unless there are major dif- B 1.00 0.88 0.88 51 ferences in salary levels), in which case the project can use other criteria to choose between A and B (such as the opportunity for client-server Table 4 expert-mentoring and risk reduction The Effect of Changing Programming Languages and Tools with Option B).
Details
-
File Typepdf
-
Upload Time-
-
Content LanguagesEnglish
-
Upload UserAnonymous/Not logged-in
-
File Pages4 Page
-
File Size-