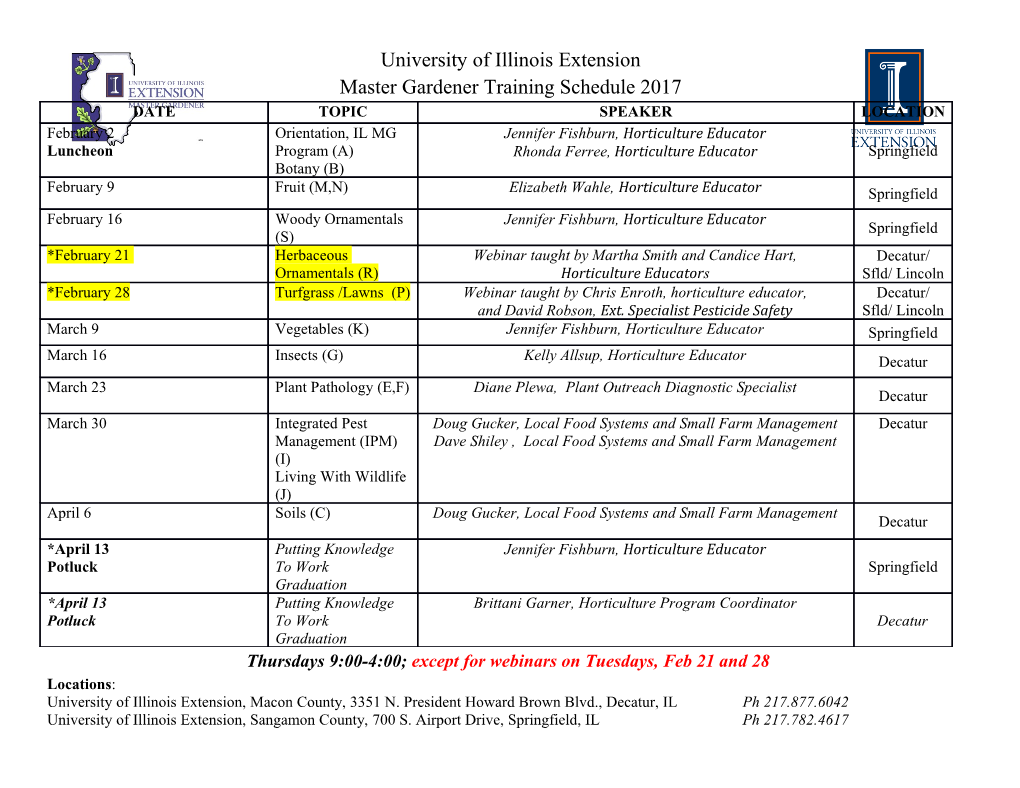
PRIMER Genome- wide association studies Emil Uffelmann 1, Qin Qin Huang 2, Nchangwi Syntia Munung 3, Jantina de Vries3, Yukinori Okada 4,5, Alicia R. Martin6,7,8, Hilary C. Martin2, Tuuli Lappalainen9,10,12 and Danielle Posthuma 1,11 ✉ Abstract | Genome-wide association studies (GWAS) test hundreds of thousands of genetic variants across many genomes to find those statistically associated with a specific trait or disease. This methodology has generated a myriad of robust associations for a range of traits and diseases, and the number of associated variants is expected to grow steadily as GWAS sample sizes increase. GWAS results have a range of applications, such as gaining insight into a phenotype’s underlying biology, estimating its heritability, calculating genetic correlations, making clinical risk predictions, informing drug development programmes and inferring potential causal relationships between risk factors and health outcomes. In this Primer, we provide the reader with an introduction to GWAS, explaining their statistical basis and how they are conducted, describe state- of- the art approaches and discuss limitations and challenges, concluding with an overview of the current and future applications for GWAS results. Polygenic risk scores Genome- wide association studies (GWAS) aim to iden- be allowed for clinical use as a stratification tool and a 7 (PRSs). Scores that provide tify associations of genotypes with phenotypes by testing genetically based biomarker . an indication of an individual’s for differences in the allele frequency of genetic variants More than 5,700 GWAS have now been conducted genetic liability to a trait or between individuals who are ancestrally similar but dif- for more than 3,300 traits8 and a push for more statistical disease, calculated using an individual’s genome, weighted fer phenotypically. GWAS can consider copy- number power has thrust GWAS sample sizes well beyond a mil- 9,10 by effect sizes obtained from variants or sequence variations in the human genome, lion participants , yielding numerous associated and genome- wide association although the most commonly studied genetic variants replicable variants for many heritable traits. Now that studies (GWAS). in GWAS are single- nucleotide polymorphisms (SNPs). reliable genetic associations for various phenotypes are GWAS typically report blocks of correlated SNPs that all known, we are faced with the next big challenge: inter- Linkage disequilibrium The non- independent show a statistically significant association with the trait preting these associations in a biological and genomic association of two alleles of interest, known as genomic risk loci. After 15 years of context. Previous GWAS have shown that most traits are in a population. GWAS 1, many replicated genomic risk loci have been influenced by thousands of causal variants11 that indi- associated with diseases and traits1, such as FTO2 for vidually confer very little risk, are often associated with obesity and PTPN22 (REF.3) for autoimmune diseases. many other traits8 and are correlated with causal and These results have sometimes provided hints into dis- non- causal variants that are physically close as a result ease biology; for example, a GWAS implicated the of linkage disequilibrium12, making direct biological, causal IL-12/IL-23 pathway in the development of Crohn’s inferences complicated13. Further, genetic associations disease4, which supported subsequent clinical trials for may differ across ancestries, complicating direct compar- drugs targeting the IL-12/IL-23 pathway5. isons between groups of individuals. Some of these limi- Results from GWAS can be used for a range of appli- tations hamper drawing unambiguous conclusions about cations. For example, trait- associated genetic variants the biological meaning of GWAS results, sometimes lim- can be used as control variables in epidemiology studies iting their utility to produce mechanistic insights or to to account for confounding genetic group differences6. serve as starting points for drug development1. Further, results can be used to predict an individual’s risk In this Primer, we aim to provide the reader with a for physical and mental disease based on their genetic comprehensive overview of GWAS, covering practical profile. Indeed, a recent study showed that genomic considerations, such as experimental design, robust risk prediction using genome- wide polygenic risk scores data analysis and data deposition, ethical implications (PRSs) for coronary artery disease, atrial fibrillation, and reproducibility of results. We also provide guidance type 2 diabetes, inflammatory bowel disease and breast on how to interpret results from GWAS using several ✉e- mail: d.posthuma@vu.nl cancer can identify disease risk as well as monogenic post- GWAS strategies and functional follow- up exper- https://doi.org/10.1038/ risk prediction strategies based on rare, highly pene- iments, as well as a discussion of the above- mentioned s43586-021-00056-9 trant mutations7. Genomic risk prediction may soon limitations and future challenges of GWAS. NATURE REVIEWS | METHODS PRIMERS | Article citation ID: (2021) 1:59 1 0123456789();: PRIMER Author addresses sample size, the experimental question and the availabil- ity of pre- existing data or the ease with which new data 1 Department of Complex Trait Genetics, Center for Neurogenomics and Cognitive can be collected. GWAS can be conducted using Research, Amsterdam Neuroscience, Vrije Universiteit Amsterdam, Amsterdam, data from resources such as biobanks or cohorts with Netherlands. disease- focused or population- based recruitment, or 2Human Genetics Programme, Wellcome Sanger Institute, Wellcome Genome Campus, Hinxton, UK. through direct to consumer studies. Assembling data 3Faculty of Health Sciences, University of Cape Town, Cape Town, South Africa. sets of a sufficient size to run a well-powered GWAS for 4Department of Statistical Genetics, Osaka University Graduate School of Medicine, a complex trait requires major investments of time and Osaka, Japan. money that go beyond the capacity of most individual 5Laboratory of Statistical Immunology, Immunology Frontier Research Center laboratories. However, there are several excellent public (WPI- IFReC), Osaka University, Osaka, Japan. resources available that provide access to large cohorts 6Analytic & Translational Genetics Unit, Massachusetts General Hospital, Boston, MA, with both genotypic and phenotypic information, and the USA. majority of GWAS are conducted using these pre-existing 7 Program in Medical and Population Genetics, Broad Institute of MIT and Harvard, resources. Even when new data have been collected in- Cambridge, MA, USA. house, these will typically be co-analysed with data from 8Stanley Center for Psychiatric Research, Broad Institute of MIT and Harvard, Cambridge, MA, USA. pre- existing resources; collecting new data is usually 9New York Genome Center, New York, NY, USA. required when more refined phenotyping is desired. 10Department of Systems Biology, Columbia University, New York, NY, USA. For all study designs, recruitment strategies must be 11Department of Child and Adolescent Psychiatry and Pediatric Psychology, Section carefully considered as these can induce collider bias and Complex Trait Genetics, Amsterdam Neuroscience, Vrije Universiteit Medical Center, other forms of bias in the resultant data16. For example, Amsterdam, Netherlands. widely used research cohorts such as the UK Biobank 12Science for Life Laboratory, Department of Gene Technology, KTH Royal Institute of recruit participants through a volunteer- based strategy, Technology, Stockholm, Sweden. which results in participants who are, on average, health- ier, wealthier and more educated than the general popu- Experimentation lation17. Further, cohorts that enrol participants from The experimental workflow of a GWAS involves several hospitals based on their disease status (such as BioBank steps, including the collection of DNA and phenotypic Japan) will have different selection biases to cohorts information from a group of individuals (such as dis- recruited from the general population18. Different eth- ease status and demographic information such as age nicities can be included in the same study, as long as and sex); genotyping of each individual using available the population substructure is considered to avoid false GWAS arrays or sequencing strategies; quality control; positive results. Individual cohorts with detailed clinical imputation of untyped variants using haplotype phasing measures may not be able to meet the required sample and reference populations; conducting the statistical test size; in these cases, ‘proxy’ phenotypes that are easier to for association; conducting a meta- analysis (optional); measure and for which there are more data can be used seeking an independent replication; and interpreting (for example, educational attainment can be used as a the results by conducting multiple post- GWAS analyses proxy for intelligence, or depressive symptoms can be (FIG. 1). At each step, possible biases and errors may enter used as a proxy for a clinical diagnosis of depression)19. the study, and therefore careful planning is required when setting up a GWAS, and adherence to standard- Genotyping. Genotyping of individuals is typically ized quality control and analysis protocols is advised. We done using microarrays for common variants or next- detail these steps below. We note that most of the issues generation sequencing methods such
Details
-
File Typepdf
-
Upload Time-
-
Content LanguagesEnglish
-
Upload UserAnonymous/Not logged-in
-
File Pages21 Page
-
File Size-