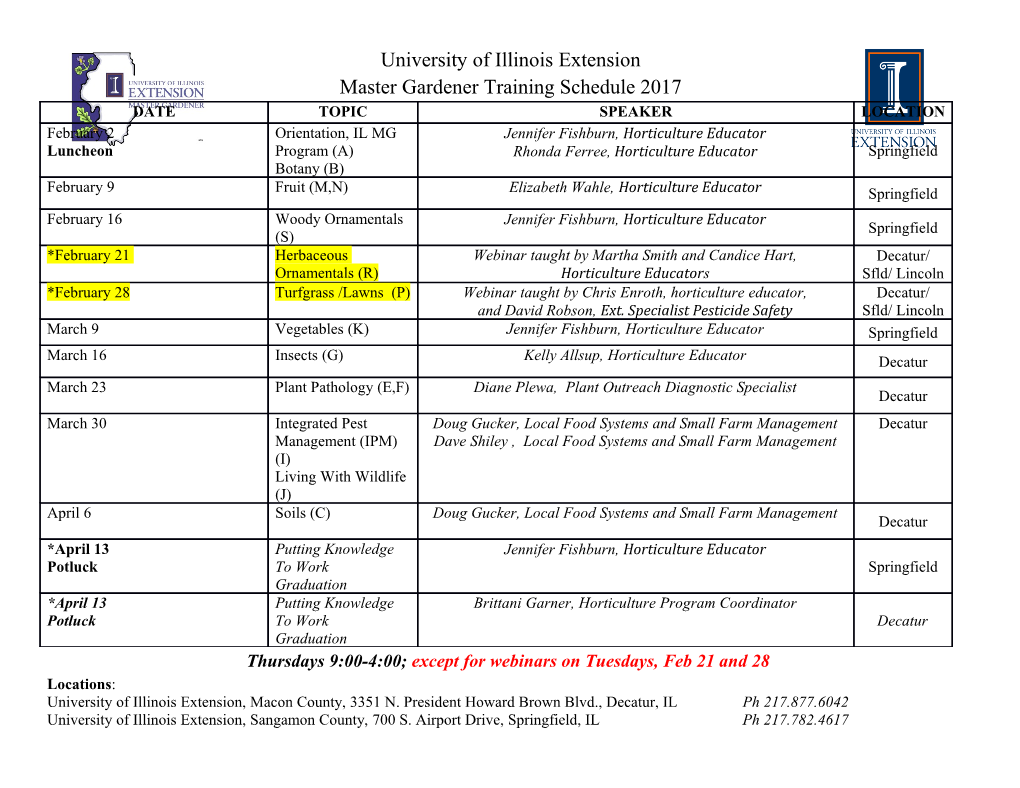
UNIVERSITY OF CALIFORNIA SAN DIEGO Machine Learning in IoT Systems: From Deep Learning to Hyperdimensional Computing A dissertation submitted in partial satisfaction of the requirements for the degree Doctor of Philosophy in Computer Science (Computer Engineering) by Mohsen Imani Committee in charge: Professor Tajana Simunic Rosing, Chair Professor Chung-Kuan Cheng Professor Ryan Kastner Professor Farinaz Koushanfar Professor Steven Swanson 2020 Copyright Mohsen Imani, 2020 All rights reserved. The dissertation of Mohsen Imani is approved, and it is ac- ceptable in quality and form for publication on microfilm and electronically: Chair University of California San Diego 2020 iii DEDICATION To my wife, Haleh, and my parents, Fatemeh, and Habibollah. iv EPIGRAPH Science without religion is lame; Religion without science is blind. — Albert Einstein v TABLE OF CONTENTS Signature Page . iii Dedication . iv Epigraph . .v Table of Contents . vi List of Figures . ix List of Tables . xi Acknowledgements . xii Vita ............................................. xiv Abstract of the Dissertation . xxi Chapter 1 Introduction . .1 1.1 Deep Learning Acceleration . .3 1.2 Brain-Inspired Hyperdimensional Computing . .4 Chapter 2 Deep Learning Acceleration with Processing In-Memory . .6 2.1 Introduction . .7 2.2 Related Work . .9 2.3 Background . 10 2.3.1 DNN Training . 11 2.3.2 Digital Processing In-Memory . 12 2.4 FloatPIM Overview . 14 2.5 CNN Computation in FloatPIM Block . 16 2.5.1 Building Blocks of CNN Training and Inference . 17 2.5.2 Feed-Forward Acceleration . 21 2.5.3 Back-Propagation Acceleration . 22 2.6 FloatPIM Architecture . 24 2.6.1 Block Size Scalability . 25 2.6.2 Inter-layer Communication . 26 2.6.3 FloatPIM Parallelism . 28 2.7 In-Memory Floating Point Computation . 29 2.7.1 FloatPIM Multiplication . 30 2.7.2 FloatPIM Addition . 30 2.8 Evaluation . 32 2.8.1 Experimental Setup . 32 vi 2.8.2 Workload . 33 2.8.3 FloatPIM & Data Representation . 34 2.8.4 FloatPIM Training . 35 2.8.5 FloatPIM Testing . 37 2.8.6 Impacts of Parallelism . 38 2.8.7 Computation/Power Efficiency . 39 2.8.8 Endurance Management . 42 2.9 Conclusion . 42 Chapter 3 Hyperdimensional Computing for Efficient and Robust Learning . 44 3.1 Introduction . 45 3.2 Hyperdimensional Processing System . 46 3.3 Classification in Hyperdimensional Computing . 48 3.3.1 Encoding Module . 49 3.3.2 HD Model Training . 50 3.3.3 Associative Search . 51 3.4 Algorithm-Hardware Optimizations of HD Computing . 52 3.4.1 QuantHD: Model Quantization in HD Computing . 52 3.4.2 SearcHD: Fully Binary Stochastic Training . 58 3.5 Hardware Acceleration of HD Computing . 63 3.5.1 D-HAM: Digital-based Hyperdimensional Associative Memory 64 3.5.2 R-HAM: Resistive Hyperdimensional Associative Memory . 66 3.5.3 A-HAM: Analog-based Hyperdimensional Associative Search 71 3.5.4 Comparison of Different HAMs . 76 3.6 Conclusion . 79 Chapter 4 Collaborative Learning with Hyperdimensional Computing . 81 4.1 Introduction . 82 4.2 Motivational Scenario . 85 4.3 Secure Learning in HD Space . 86 4.3.1 Security Model . 86 4.3.2 Proposed Framework . 87 4.3.3 Secure Key Generation and Distribution . 88 4.4 SecureHD Encoding and Decoding . 90 4.4.1 Encoding in HD Space . 91 4.4.2 Decoding in HD Space . 93 4.5 Collaborative Learning in HD Space . 98 4.5.1 Hierarchical Learning Approach . 98 4.5.2 HD Model-Based Inference . 100 4.6 Evaluation . 101 4.6.1 Experimental Setup . 101 4.6.2 Encoding and Decoding Performance . 102 4.6.3 Evaluation of SecureHD Learning . 102 vii 4.6.4 Data Recovery Trade-offs . 105 4.7 Conclusion . 107 Chapter 5 Summary and Future Work . 108 5.1 Thesis Summary . 109 5.2 Future Direction . 110 Bibliography . 111 viii LIST OF FIGURES Figure 2.1: DNN computation during (a) feed-forward and (b) back-propagation. 10 Figure 2.2: Digital PIM operations. (a) NOR operation. (b) 1-bit addition. 13 Figure 2.3: Overview of FloatPIM. 15 Figure 2.4: Overview of CNN Training. 17 Figure 2.5: Vector-matrix multiplication. 18 Figure 2.6: Convolution operation. 19 Figure 2.7: Back-propagation of FloatPIM. 23 Figure 2.8: FloatPIM memory architecture. 25 Figure 2.9: FloatPIM training parallelism in a batch. 29 Figure 2.10: In-memory implementation of floating point addition. 31 Figure 2.11: FloatPIM energy saving and speedup using floating point and fixed point representations. 36 Figure 2.12: FloatPIM efficiency during training. 36 Figure 2.13: FloatPIM efficiency during the testing. 38 Figure 2.14: The impact of parallelism on efficiency. 40 Figure 2.15: (a) FloatPIM area breakdown, (b) efficiency comparisons. 41 Figure 3.1: (a) Overview of the HD classification consist of encoding and associative memory modules. (b) The encoding module maps a feature vector to a high- dimensional space using pre-generated base hypervectors. (c) Generating the base hypervectors. 51 Figure 3.2: (a) QuantHD framework overview. (b) Binarizing and ternarizing the trained HD model. 53 Figure 3.3: Energy consumption and execution time of QuantHD, conventional HD and BNN during training . 57 Figure 3.4: Energy consumption and execution time of QuantHD during inference . 58 Figure 3.5: Overview of SearcHD encoding and stochastic training. 59 Figure 3.6: Classification accuracy of SearcHD, kNN, and the baseline HD algorithms. 61 Figure 3.7: Language classification accuracy with wide range of errors in Hamming distance using D = 10;000. 64 Figure 3.8: Overview of D-HAM. 65 Figure 3.9: Overview of R-HAM: (a) Resistive CAM array with distance computation; (b) A 4 bits resistive block; (c) Sensing circuitry with non-binary code generation. 67 Figure 3.10: Match line (ML) discharging time and its relation to detecting Hamming distance for various CAMs. 69 Figure 3.11: Energy saving of R-HAM using structured sampling versus distributed volt- age overscaling. 71 Figure 3.12: Overview of A-HAM: (a) Resistive CAM array with LTA comparators; (b) Circuit details of two rows. 72 Figure 3.13: Minimum detectable distance in A-HAM. 75 ix Figure 3.14: Multistage A-HAM architecture. 76 Figure 3.15: Energy-delay of the HAMs with accuracy. 77 Figure 3.16: Area comparison between the HAMs. 78 Figure 3.17: Impact of process and voltage variations for the minimum detectable Ham- ming distance in A-HAM. 79 Figure 4.1: Motivational scenario . 84 Figure 4.2: Execution time of homomorphic encryption and decryption over MNIST dataset . 85 Figure 4.3: Overview of SecureHD . 86 Figure 4.4: MPC-based key generation . 88 Figure 4.5: Illustration of SecureHD encoding and decoding procedures . 88 Figure 4.6: Value extraction example . 91 Figure 4.7: Iterative error correction procedure . 94 Figure 4.8: Relationship between the number of metavector injections and segment size 96 Figure 4.9: Illustration of the classification in SecureHD . 97 Figure 4.10: Comparison of SecureHD efficiency to homomorphic algorithm in encoding and decoding . 100 Figure 4.11: SecureHD classification accuracy . 103 Figure 4.12: Scalability of SecureHD classification . 105 Figure 4.13: Data recovery accuracy of.
Details
-
File Typepdf
-
Upload Time-
-
Content LanguagesEnglish
-
Upload UserAnonymous/Not logged-in
-
File Pages144 Page
-
File Size-