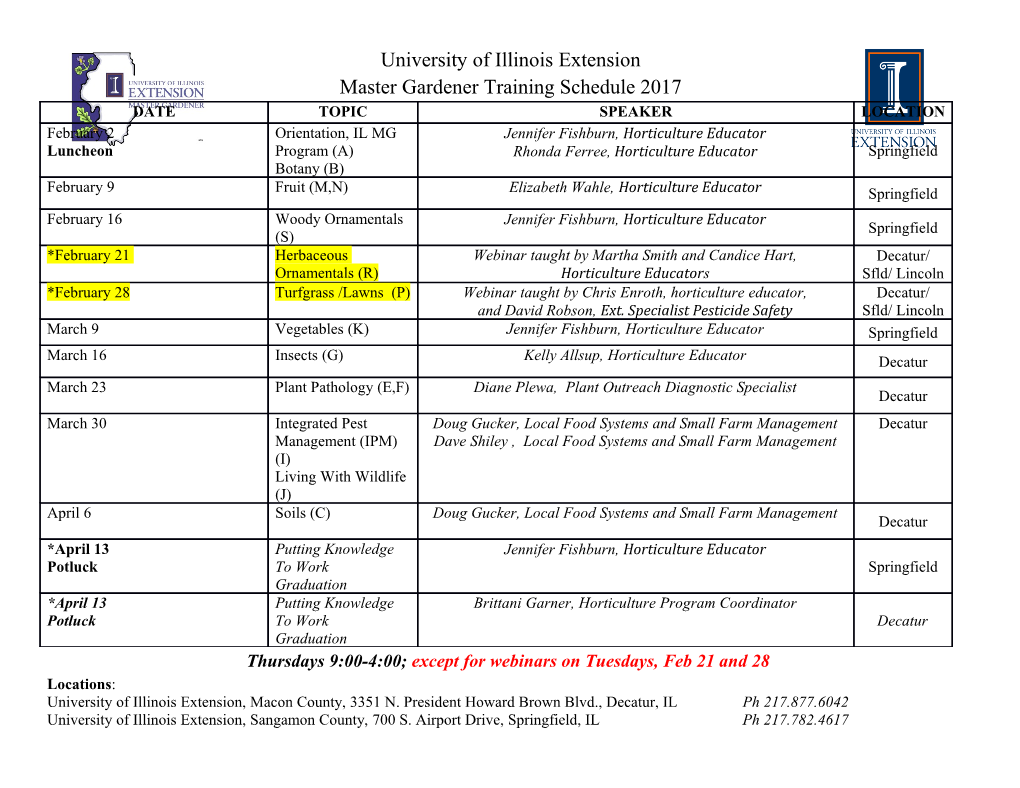
Head Pose Determination from One Image Using a Generic Model ; Ikuko Shimizu1;3 Zhengyou Zhang2 3 Shigeru Akamatsu3 Koichiro Deguchi1 1 Faculty of Engineering, University of Tokyo, 7-3-1 Hongo, Bynkyo-ku, Tokyo 113, Japan 2 INRIA, 2004 route des Lucioles, BP 93, F-06902 Sophia-Antipolis Cedex, France 3 ATR HIP, 2-2 Hikaridai, Seika-cho, Soraku-gun, Kyoto 619-02, Japan e-mail: [email protected] Abstract use a generic model of the human head, which is applicable to many persons and is able to consider the variety of facial We present a new method for determining the pose of a expressions. Such a model is constructed from the results of human head from its 2D image. It does not use any arti®cial intensive measurements on the heads of many people. With markers put on a face. The basic idea is to use a generic model this 3D generic model, we suppose that an image of a head of a human head, which accounts for variation in shape and is the projection of this 3D generic model onto the image facial expression. Particularly, a set of 3D curves are used to plane. Then, the problem is to estimate this transformation, model the contours of eyes, lips, and eyebrows. A technique which is composed of the rigid displacement of the head and called Iterative Closest Curve matching (ICC) is proposed, a perspective projection. which aims at recovering the pose by iteratively minimizing We take a strategy that we de®ne edge curves on the 3D the distances between the projected model curves and their generic model in advance. For edge curves, we use the closest image curves. Because curves contain richer infor- contours of eyes, lips, eyebrows, and so on. They are caused mation (such as curvature and length) than points, ICC is by discontinuity of the re¯ectance and appear in the image both more robust and more ef®cient than the well-known iter- independent of the head pose in 3D space. (We call these ative closest point matching techniques (ICP). Furthermore, edges stable edges.) For each de®ned edge curve on the the image can be taken by a camera with unknown internal generic model, we search its corresponding curves in the parameters, which can be recovered by our technique thanks image. This is done by ®rst extracting every edge from the to the 3D model. Preliminary experiments show that the image and next using the relaxation method. proposed technique is promising and that an accurate pose After we have established the correspondences between estimate can be obtained from just one image with a generic the edges curves on the model and the edges in the image, head model. we are to estimate the head pose. For this purpose, we de- velop ICC (Iterative Closest Curve) method which minimizes the distance between the curves on the model and the corre- 1. Introduction sponding curves in the image. This ICC method is similar This paper deals with techniques for estimating the pose to the ICP (Iterative Closest Point) method [5] [8], which of a human head using its 2D image taken by a camera. They minimizes the distance from points of a 3D model to the cor- are useful for the realization of a new man-machine interface. responding measured points of the object. Because a curve We present a new method for the accurate estimation of a head contains much richer information than a point, curve corre- pose from only one 2D image using a 3D model of human spondences can be established more robustly and with less heads. By a 3D model with characteristic curves, our method ambiguity, and therefore, pose estimation based on curve cor- does not use any makers on the face and uses an arbitrary respondence is thought to be more accurate than that based camera with unknown parameters to take images. on point correspondence. Several methods have been proposed for head pose esti- The ICC method is an iterative algorithm and needs a mation which detect facial feature and estimate pose by loca- reasonable initial guess. To obtain it, prior to applying the tion of these features using 2D face model[1] or by template ICC method, we roughly compute the pose of a head and matching[3]. Jebara[7] tracked facial features in the sequence the camera parameters by using the correspondence of conics of images to generate 3D model of face and estimate pose of ®tted to the stable edges. The computation is analytically face. carried out. Then, a more precise pose are estimated by the We use 3D models of human heads in order to estimate a ICC method. In this step, in addition to the stable edges, we pose from only one 2D image. There are some dif®culties use variable edges, which are pieces of occluding contours with such 3D models; head shapes are different from one of a head, e.g. the contour of the face. person to another person and, furthermore, facial expressions Our method is currently applied for extracted face area may vary even for one person. Nevertheless, it is unrealistic from the natural image or the face image with unicolor back- to have 3D head models for all persons and for all possible ground. Many techniques have been reported in the literature facial expressions. To deal with effectively this problem, we to extract the face from clustered background. Construction of the Generic Mo del 2. Notation 3.1. t =X; Y ; Z The coordinates of a 3D point X in a world We represent the deformation of the 3D shape of a human t =u; v coordinate system and its image coordinates x are head (i.e., shape differences and the changes of the facial V [X ] related by expression) by the mean X and the variance of each point on the face. These variables are calculated from the X x Ä results of measuring heads of many people. To do so, we need =P ; = P X : or simplyx Ä 1 1 1 a method for sampling points consistently for all faces. That is, we need to know which point on a face corresponds to a P where is an arbitrary scale factor, is a 3 4 matrix, called point on another face. Many methods have been proposed for Ä t such a purpose and we can use them; we use the resampling =X; Y ; Z; the perspective projection matrix, and X 1 and t method [4] developed in our laboratory. This method uses =u; v ; P xÄ 1 . The matrix can be decomposed as several feature points (such as the corners of the eyes, the = AT : P (2) vertex of the nose, and so on) as reference points. Using these reference points, the shape of a face is segmented into The matrix A maps the coordinates of the 3D point to the several regions and further each region is resampled. We image coordinates. The general matrix A can be written as choose the sample points using this method. 0 1 3.2. Edge Extraction in the Mo del u o u 0 0 @ A v A = : o 0 v 0 (3) As mentioned earlier, we use two types of edges: stable 0010 edges and variable edges. For stable edges, we extract them beforehand from the 2D image taken at the same time as the v u and are the product of the focal length and the hori- acquisition of the 3D data of a head. They are the contours of u v o zontal and vertical scale factors, respectively. o and are the eyes, lips, and eyebrows. We obtain their corresponding the coordinates of the principal point of the camera, i.e., the curves on the head by back-projecting them onto the 3D intersection between the optical axis and the image plane. model. For variable edges, which are occluding contours and u v o For simplicity of computation, both o and are assumed depend on the head pose and camera parameters, we extract to be 0 in our case, because the principal point is usually at them whenever these parameters change. Figure 1 shows the center of the image. an example of images of the generic model with stable and The matrix T denotes the positional relationship between variable edges. It shows that the stable edges (i.e., the eyes the world coordinate system and the image coordinate system. and lips) do not change under the change of the pose, and the T can be written as variable edge (i.e., the contour of the face ) changes whenever the pose changes. R t T = : (4) 0 1 t R is a 3 3rotationmatrixand is a translation vector. Note that there are eight parameters to be estimated: two v camera parameters u and , three rotation parameters, and three translation parameters. I k = ;:::;K k We use C 1 to denote the -th stable curve k W P l = ;:::;L l in the image, and C 1 ,the -th stable curve l I W C C in the model projected by P .Both and are 2D curves. k l I Figure 1. A generic mo del of a head. In all p oses, C is used to denote the contour of the face in the image. o W the stable edges such as the eyes and lips do P P C is the contour of the face projected by . o I I k not change. The variable edges change b ecause k C x is the 2D the point belonging to the -th curve in k i W they are o ccluding contours.
Details
-
File Typepdf
-
Upload Time-
-
Content LanguagesEnglish
-
Upload UserAnonymous/Not logged-in
-
File Pages6 Page
-
File Size-