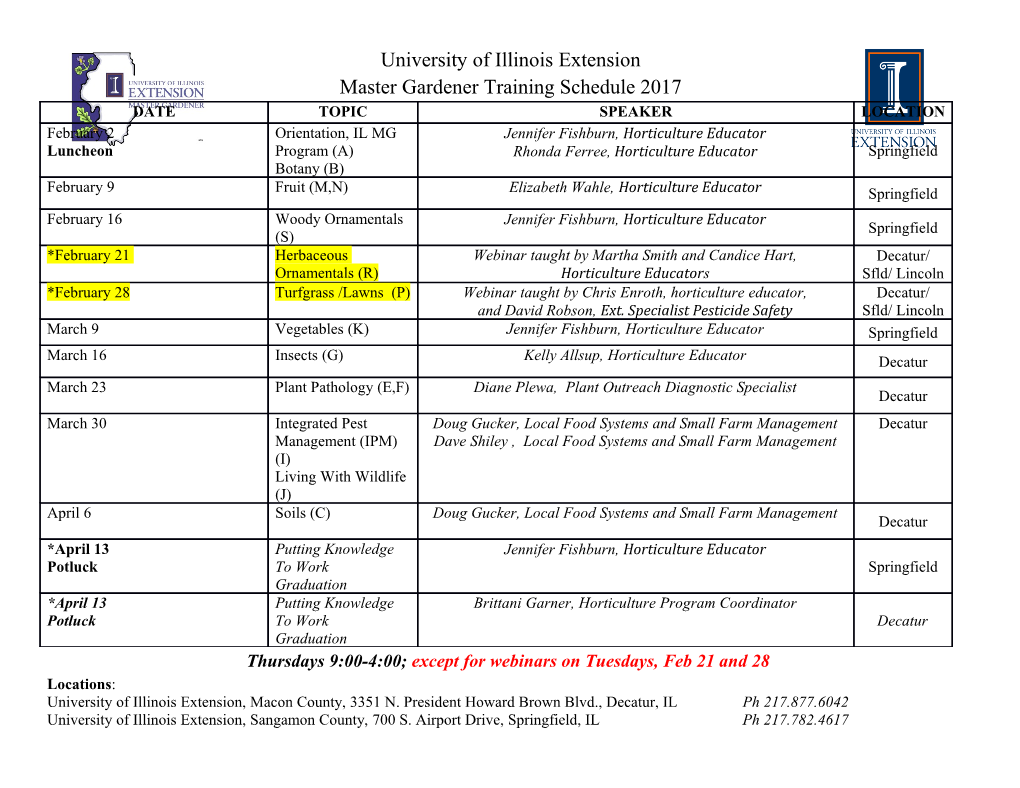
Agricultural Economics Research Review 2018, 31 (1), 29-44 DOI: 10.5958/0974-0279.2018.00003.4 Localized estimates of the incidence of indebtedness among rural households in Uttar Pradesh: an application of small area estimation technique Hukum Chandra ICAR-Indian Agricultural Statistics Research Institute, New Delhi-110012, India Abstract Socioeconomic conditions exhibit significant variation at higher levels of spatial disaggregation, yet these have not received much attention in the economic and statistical investigations in terms of their identification and prioritization for targeting of efforts and investment. Applying small area estimation (SAE) approach, in this paper we have generated estimates of the incidence of indebtedness among social groups across districts of Indian state of Uttar Pradesh. The findings show that the estimates from SAE are more precise and representative of the spatial heterogeneity in the socioeconomic conditions than do the direct survey estimates. Such estimates of spatial inequality in the incidence of indebtedness provide useful information to financial institutions to develop financial products and services suited to the marginalized regions and social groups; and to policymakers to take appropriate measures to address the problem of agrarian distress which is often attributed to the indebtedness. Keywords Socio-economics, Indebtedness, Small area estimates, Diagnostics, Spatial mapping JEL classification C810, C890 1 Introduction that require minimal efforts to achieve the As economic planning becomes more decentralized, developmental goals, neglecting those areas or groups the need for micro-level statistics on socioeconomic for whom these are needed the most. Nonetheless, local conditions, infrastructure and institutions cannot be developmental goals cannot be set and monitored in undermined. Micro-level statistics is essential to target the absence of baseline information. The existing data, social and spatial heterogeneity in the programmes and based on national level socioeconomics surveys, strategies aimed at alleviating the inter-personal and produce estimates that are representative of the macro- inter-regional inequalities. However, our understanding geographical units and cannot be used directly to of the spatial and social inequalities at this level of produce reliable micro or local (also referred as small disaggregation is severely hampered by the lack of area) level estimates because of the small sample sizes availability of statistics. The available statistics at (Pfeffermann 2002; Molina & Rao 2015). national or state level mask variations which is Conducting surveys at higher levels of spatial available at local levels, restricting designing and disaggregation to generate local statistics is time- targeting of heterogeneity at higher levels of spatial consuming and also costly. We, therefore, need special disaggregation, and the scope for monitoring and techniques that can generate estimates at micro-level evaluation of parameters locally within and across utilizing the already available survey data. Small area administrative units. The macro developmental estimation (SAE) is such a technique that can produce planning often leads to sub-optimal interventions and reliable estimates at micro-level. The technique is a outcomes because it often focuses on groups or areas model-based method that links the variable of interest *Corresponding author: [email protected] from survey with the auxiliary information available 30 Chandra H from other data sources for small areas. Depending on area. The state accounts for 16.2% of the country’s the availability of auxiliary information, small area total population with considerable spatial and social models are of two broad types. One, the area level heterogeneity. Agriculture is the main occupation of random effect models that are applied when the majority of the population in the state. Also, it has a auxiliary information is available only at area level. large number of poor belonging to various social These relate small area direct survey estimates to area- groups, viz. scheduled caste (SC), scheduled tribe (ST), specific auxiliary information (Fay & Herriot 1979). other backward castes (OBCs), and other castes Two, the nested error unit level regression models, (Others). The scheduled castes/tribes are at the bottom proposed originally by Battese et al. (1988) that relate of the hierarchy of Indian social structure, followed unit values of a variable to the unit-specific auxiliary by the OBCs and other or upper castes. Thus, it is information. reasonable for us to apply SAE technique to generate In this paper, we apply SAE technique to produce estimates of incidence of indebtedness at district level model-based estimates of the incidence of indebtedness among social groups in Uttar Pradesh. For the purpose, among social groups in different districts of Uttar we require two types of variables: (i) the variable of Pradesh by linking information from the All-India Debt interest for which small area estimates are required. and Investment Survey 2012-13 (AIDIS) and the Our variable of interest in the incidence of Census 2011. Here, we consider small areas as districts, indebtedness, that is, the proportion of indebted and social groups within districts. Such estimates households in a social group or in a district. We extract provide useful information to financial institutions to information on indebtedness from AIDIS 2012-13. A develop financial products and services for the household is defined as indebted if it has an outstanding marginalized regions and social groups at a higher level loan (from respective source) as on June 30, 2012. (ii) of spatial disaggregation; and to policymakers to take auxiliary (covariates) variables that are available for appropriate measures to address the problem of agrarian the population. The covariates that we in this analysis distress which is often attributed to indebtedness. are available at district level in the Census 2011. Rest of the paper is organized as follows. In section 2, However, there could be an issue of concordance of we discuss data used in this paper. Section 3 provides information between Census 2011 and AIDIS 2012- an overview of SAE technique that we use to generate 13. But, the covariates that we use from Census are incidence of indebtedness among social groups across unlikely to change much in such a shorter period. districts. In section 4, we introduce diagnostic The estimates of indebtedness in AIDIS are based on procedures to examine model assumptions and validate stratified multi-stage random sampling; districts as small area estimates, and discuss the results. strata, census villages as first stage units and households Concluding remarks are made in the last section. as ultimate stage units. In Uttar Pradesh, there are a total 8587 surveyed households (including both 2 Data indebted and non-indebted) spread over 71 districts. Uttar Pradesh is the most populous state of Indian Table 1 presents sample sizes of different social groups, Union having 828 persons per sq.km of geographical and the sample sizes of social groups across districts Table 1. Social groups and their sample sizes in AIDIS 2012-13 in Uttar Pradesh Social group Description of households Sample size type Min Max Average Total All All 56 195 121 8587 ST Scheduled tribe 1 19 3 81 SC Scheduled caste 4 88 33 2327 OBC Other Backward class 20 118 64 4528 Others Upper castes 4 55 24 1651 Source: AIDIS, 2012-13. Localized estimates of rural indebtedness 31 are shown in table 2. Across districts, the sample size from 81 households Theoretically, SAE technique ranges between 56 to 195 with an average of 121. The enables us to produce estimates for the districts that do sample sizes become too small if sub-grouped further not have any sample of this social group. Practically, by social groups. For example, the sample size of ST this social group does not require estimates of varies from 1 to 19 across district with an average of indebtedness in these districts. Furthermore, of the 27 3. Further, the sample comes only from 27 districts districts, 19 districts have sample size of not more than Table 2. Distribution of sample size by social group across districts in Uttar Pradesh District Total ST SC OBC Others District Total ST SC OBC Others Saharanpur 140 0 35 90 15 Lalitpur 56 1 4 45 6 Muzaffarnagar 168 0 36 99 33 Hamirpur 56 0 7 34 15 Bijnor 154 0 42 76 36 Mahoba 56 0 15 34 7 Moradabad 168 0 50 85 33 Banda 84 0 22 53 9 Rampur 112 1 17 72 22 Chitrakoot 56 0 12 40 4 Jyotiba Phule Nagar 84 0 29 34 21 Fatehpur 140 0 34 65 41 Meerut 105 0 26 58 21 Pratapgarh 168 0 39 82 47 Baghpat 61 0 14 32 15 Kaushambi 84 0 38 31 15 Ghaziabad 83 1 25 44 13 Allahabad 193 4 50 118 21 Gautam B. Nagar 56 0 18 22 16 Barabanki 168 1 72 76 19 Bulandshahar 140 0 35 50 55 Faizabad 112 0 27 46 39 Aligarh 140 0 37 60 43 Ambedkar Nagar 112 0 50 32 30 Hathras 84 0 35 36 13 Sultanpur 168 1 40 86 41 Mathura 98 2 28 49 19 Bahraich 168 0 30 94 44 Agra 138 0 27 60 51 Shrawasti 56 0 6 22 28 Firozabad 112 2 21 81 8 Balrampur 112 0 13 62 37 Etah 84 0 14 47 23 Gonda 168 0 38 76 54 Mainpuri 83 1 31 47 4 Siddharth Nagar 153 2 26 90 35 Budaun 168 0 25 104 39 Basti 140 0 40 84 16 Bareilly 168 0 26 118 24 Sant Kabir Nagar 82 0 29 47 6 Pilibhit 112 2 24 71 15 Mahrajganj 140 1 26 96 17 Shahjahanpur 140 3 29 94 14 Gorakhpur 168 4 52 99 13 Kheri 168 17 45 72 34 Kushinagar 168 1 41 103 23 Sitapur 168 0 88 63 17 Deoria 168 0 35 101 32 Hardoi 168 1 49 87 31 Azamgarh 192 0 83 88 21 Unnao 140 3 45 41 51 Mau 112 1 41 51 19 Lucknow 84 0 49 20 15 Ballia 168 3 18 115 32 Rae Bareli 168 0 61 60 47 Jaunpur 195 0 63 107 25 Farrukhabad 84 0 8 51 25 Ghazipur 167 0 36 115 16 Kannauj 84 1 13 59 11 Chandauli 112 0 47 49 16 Etawah 84 0 18 39 27 Varanasi 108 2 36 56 14 Auraiya 83 2 35 35 11 Sant R.
Details
-
File Typepdf
-
Upload Time-
-
Content LanguagesEnglish
-
Upload UserAnonymous/Not logged-in
-
File Pages16 Page
-
File Size-