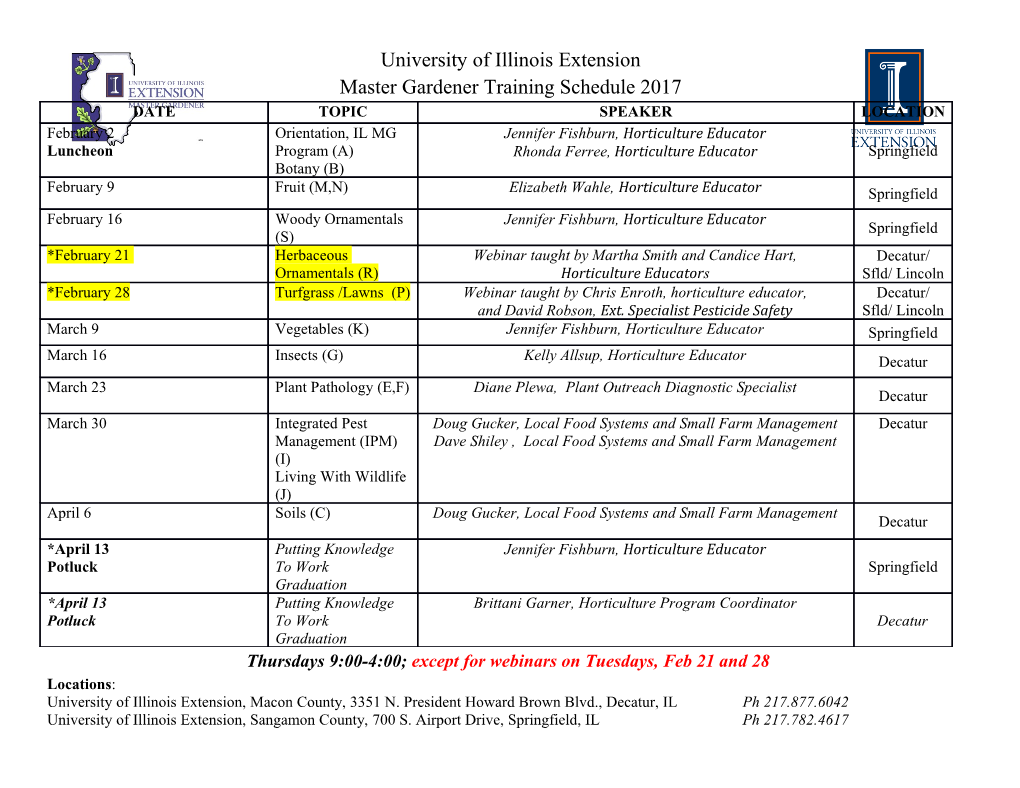
Machine Learning Platforms Learning Machine Machine Digital Catapult www.papis.io 101 Euston Road London NW1 2RA Learning 0300 1233 101 www.digicatapult.org.uk Platforms Digital Catapult Digital Using, extending and creating platforms to accelerate machine learning efforts and generate growth Research report 2019 Report contents Choosing machine learning platforms and filling the gaps 2 Introduction 48. 5 Machine learning deployment platforms 2 About machine learning platforms 48 Packaging models 4 Who is the report written for? 49 Case study: serving predictions 4 Outline of this report with Flask on Floyd 5 Scope of this report 50 Case study: A/B testing models in production with Amazon SageMaker 51 Serverless model serving platforms 8. 1 Pre-trained models as a service platforms 52 Example model management 9 Giving machine intelligence superpowers platform: Seldon Deploy to software via APIs 10 Platforms as a service to leverage pre-trained models 56. 6 Choosing machine learning platforms and filling the gaps 57 Evaluating predictions 14. 2 Vertical machine learning as a service (MLaaS) platforms 57 Preparing prediction APIs for usage in production 15 Case study: building a custom fraud detection model with the Sift API 58 Performance monitoring and model lifecycle management 16 MLaaS platforms for predictive customer relationship management 60 Example architecture of machine learning system components 17 Other example platforms 61 Finding the right type of platform to use 64 Platform selection criteria 20. 3 Semi-specialised machine learning as a service (MLaaS) platforms 65 Scaling to many use cases: building proprietary platforms 20 Case study: building a custom vision model with the Clarifai API 24 Example Vision and Language platforms 68 Conclusions 25 Behind the scenes: automated ML (‘AutoML’) and transfer learning 70 Footnotes 28 4. Machine learning development platforms 74 About 29 High-level platforms as a service 74 Digital Catapult/Machine Intelligence Garage 34 Self-hosted studios 74 PAPIs 38 Cloud machine learning integrated 74 Author development environments (IDEs) 43 Adding featurisation to machine learning pipelines Machine learning platforms 1 Introduction Machine learning (ML) platforms reduce ABOUT MACHINE LEARNING PLATFORMS the time and cost of integrating ML into Gartner defines ML platforms as “software products that data scientists use to help them develop and deploy their software applications, for organisations own data science and machine-learning solutions”1. For of all levels of ML maturity. ML platforms the purpose of this report this definition is extended to can be ready-made or custom-built. include products that can be used by software developers too. This report focuses on the integration of ML into This report provides a survey of ready-made software, for the purpose of creating machine intelligence, platforms, grouped into different types and rather than on building scientific knowledge from data. presented from starting with those that are ML platforms can come in different formats, as they can be based on open source or commercial software, and can aimed at general users, all the way to run on-premises or in the cloud. Some of these platforms platforms that enable machine learning are made available “as a service”, which Wikipedia2 defines experimentation and optimisation. It shows as “a category of cloud computing services3 that provides a how startups and larger organisations can platform4 allowing customers to develop, run, and manage applications without the complexity of building and benefit from utilising these platforms, and maintaining the infrastructure typically associated with what can be their limitations. developing and launching an app”. This model has the following advantages: This report can also inspire the design of – There are no set-up costs (which is ideal for startups custom-built platforms, which can serve and for pilot projects) two different purposes: – Users pay for the functionality they are interested in (not for hosting), according to usage 1. Internal usage to accelerate ML efforts – There’s nothing to install 2. Selling access to generate economic growth – Users do not need to worry about where the application program interface (API) and the models are running, for example, which processor to use (CPU/GPU), how to scale up or down as the volume of prediction requests evolves 2 Machine learning platforms Introduction Introduction “ We believe most people will begin their AI journeys using cloud services rather than creating and managing million-node neural networks in their own data centres. Even if you eventually outgrow a publicly available AI cloud service, your experience using it will help inform the design and scaling of your own AI infrastructure.” Andreessen Horowitz — AI Playbook5 Introduction Machine learning platforms 3 Introduction WHO IS THE REPORT WRITTEN FOR? OUTLINE OF THIS REPORT This paper has been written predominantly for users The different types of ML platforms are presented and potential users of ML platforms, including: in the order below: – Software developers and product managers, who – Pre-trained models as a service: platforms that give will be adding machine intelligence superpowers access to predictions from pre-trained models, aimed to their applications at product teams (including domain experts and software developers) – Data scientists, who will be shipping ML models in production, and extending toolkits to run ML experiments – Vertical ML as a service: platforms that allow the creation and deployment of custom predictive models for a specific – Data and ML engineers, extending these platforms type of business problem, aimed at product teams or creating new ones – Semi-specialised machine learning as a service (MLaaS): – Domain experts, exploring ideas of new ML use cases, platforms that allow the creation and deployment of assessing their feasibility, and building prototypical custom predictive models for a specific type of input solutions to business problems and task, aimed at product teams This report will also be of interest to managers, analysts, – ML development platforms: platforms that include an and consultants who have heard that machine learning experimentation component facilitating the creation can help improve products, software applications, and of predictive models, used by data scientists as well processes, who are trying to figure out the right way for as experts in the domain where ML is applied their organisation to make the most of ML, but who do not have the time to go through all the options. – ML deployment platforms: platforms that simplify the deployment of models created by data scientists, used by data engineers and data scientists This report ends with recommendations on how to choose the best platforms for individual use cases, and how to fill their gaps in the creation of production machine learning systems. 4 Machine learning platforms Introduction Introduction SCOPE OF THIS REPORT The number of vertical ML platforms is expected to VentureScanner segments US AI startups into 13 groups, continue to grow. David Kelnar of MMC Ventures remarks including Machine Learning Platforms, which had 357 that nine in ten AI startups in Europe are vertical7, and it is startups at the end of Q1 2019, and Computer Vision anticipated that many of them could benefit from offering Platforms, which had 234 startups in the same period. platforms for others to build on. Bradford Cross of Data Collective Venture Capital sees vertical AI startups as The objective of this report is not to provide an exhaustive providing fully-integrated solutions to business problems8, list of platforms, but to give a broad overview of the types which are powered by predictive models. However, the of products and services that are available. It provides authors of Prediction Machines9 argue that it could be examples of platforms with different characteristics, more advantageous in some cases to give access to the from which to extract general principles, ideas and models’ raw predictions, as illustrated with an example recommendations. The examples that are discussed in the medical sector. The vertical platforms that are here were chosen for illustration purposes, and this presented here provide both business solutions and report is not intended to provide a benchmark. It does, access to raw predictions. however, provide advice to create individual benchmarks and to help readers to find or create the best ML platform, This report is written to inspire organisations not only for their own needs or those of their customers. to use existing ML platforms, but to build on them and create their own vertical platforms, for their customers The number of ML platforms quoted above could be even to use and to build on. bigger, if vertical ML platforms are considered. Indeed, some of these were listed by VentureScanner in the Machine Learning Applications group, including Sift6, a startup that allows users to build custom fraud detection models and to access them in a production application via a prediction API. Introduction Machine learning platforms 5 Contents 8 Pre-trained models as a service platforms 9 Giving machine intelligence superpowers to software via APIs 10 Platforms as a service to leverage pre-trained models 6 Machine learning platforms Pre-trained models as a service platforms 1. Pre-trained models as a service platforms Pre-trained models as a service platforms Machine learning platforms 7 1. Pre-trained models as a service platforms This example relates to developers of a To add further context and examples: imagine developing software application that can be improved a customer support application for a global company. It wants to improve the app to reduce the average response with predictive features. This could be a time and increase customer satisfaction. When receiving a food delivery app, for instance (think new support ticket, the first step towards an answer is to UberEats), to which predicted delivery assign a representative based on the customer’s language. times could be added. Predictions would This step could be automated with a language detection model.
Details
-
File Typepdf
-
Upload Time-
-
Content LanguagesEnglish
-
Upload UserAnonymous/Not logged-in
-
File Pages78 Page
-
File Size-