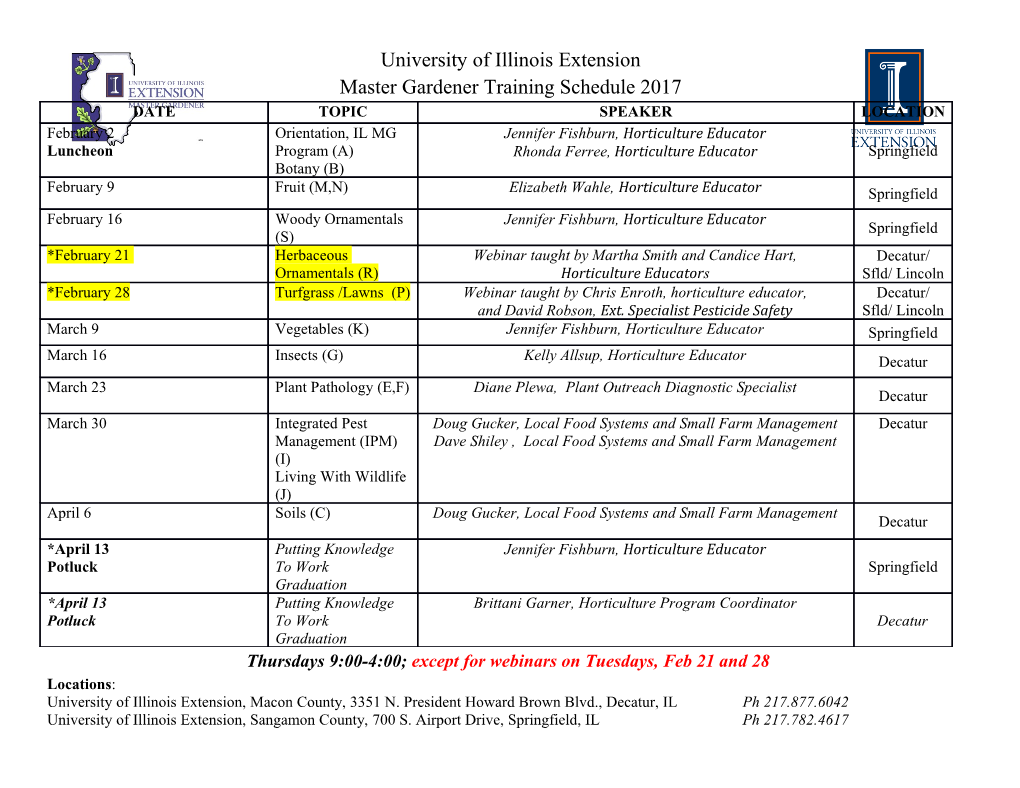
Dynamic Toll Prediction Using Historical Data on Toll Roads: Case Study of the I-66 Inner Beltway Sara Zahedian*, Amir Nohekhan, Kaveh Farokhi Sadabadi Center for Advanced Transportation Technology, University of Maryland, United States * Corresponding author email: [email protected] Abstract Providing the users of a dynamic tolling system with predictions of tolling prices and the travel time difference between the toll road and the alternative routes enables them to make their travel decisions before starting their trip. This study aims to provide accurate predictions of tolling price through training and testing random forest, multilayer perceptron, and long short-term memory models and compare them with the current situation that the best prediction is extending the current toll to the next timesteps. The prediction time horizon includes five 6-minute time intervals ahead of the present time. The prediction performance of models over the testing set reveals that while all the models were significantly better than the base model, the random forest outperforms all models. For instance, while in the trained models, the mean absolute error range is from $1.5 to $2.5 for the next six minutes to the next 30 minutes, respectively, the same measure in the base model is in the range of $2.5 to $6. The prediction of travel time difference along the toll road and its alternative route with the shortest travel time revealed that the multilayer perceptron performs marginally better than the base model. However, due to a relatively stable travel time difference, the current travel time difference is an acceptable prediction for the next 30 minutes prediction horizon. Keywords: Dynamic tolling, Toll prediction, Machine learning, High-occupancy toll lanes, Travel time 1. Introduction This study aims to train and investigate various machine learning models to predict the toll price based on the historical data for facilities with dynamic tolling. Moreover, it examines the travel time difference between the toll facility and the alternative route with the shortest travel time for different prediction horizons to build a complete picture of the system's performance under the dynamic tolling scheme. The implementation of dynamic tolling systems is an efficient manner for reducing traffic congestion (Zhang et al., 2008; Yin and Lou, 2009). In a dynamic tolling system, the price that users should pay to use the toll facility or enter a congested region is determined based on the travel demand at each particular time interval. In theory, the users alter their trip start time, mode choice, route choice, or other decisions based on the tolling amount (Button and Verhoef, 1998; Yang and Huang, 2005). Therefore, the effectiveness of a dynamic tolling system not only relies on an optimal tolling scheme (Zhu and Ukkusuri, 2014) but also on providing an accurate prediction of the tolling price and the time which can be saved using the toll facility to the users in advance (Mahmassani and Jayakrishnan, 1991; Noland, 1997; Knorr et al., 2014; Qi et al., 2020). This study contributes to the literature in predicting the toll price and travel time difference between the toll facility and the best alternative route for different timesteps without any knowledge of the operator's tolling algorithm. Therefore, the introduced models predict the amount of toll by extracting the interaction pattern between users and the operator. Such prediction is beneficial to users by reducing the uncertainty of their generalized travel costs. Moreover, such interactive communication between the users and the operator can minimize public opposition in implementing toll facilities (Odeck and Kjerkreit, 2010; Gu et al., 2018). Besides, it provides useful information to the system operator because unlike the actual toll set based on the existing algorithms, the predicted toll is estimated based on the historical data. Therefore, the operator can use it to gain insight into the users’ interactions with the system in practice. This study uses three well-known machine learning models, namely, Random Forest (RF), Multilayer Perceptron (MLP), and Long short-term memory (LSTM), to address the toll prediction problem. These models are trained using the historical data of the I-66 interstate inside the Capital Beltway, which is one of the most debating tolling facilities in the United States. This route is a High Occupancy Toll (HOT) facility during the rush hours in peak traffic direction. The dynamic toll fare is set every 6-minute to maintain a speed of 55 miles per hour along the corridor. This study uses the data of travel time, traffic flow, and the amount of toll over approximately 18 months and trains models that, given the data of the time t, predict the amount of toll for the next five 6-minute time intervals (i.e., t+6, t+12, …, t+30 minutes). The introduced models' performance is compared against the existing condition in which the best toll estimation available to the users is the current toll amount provided by the operator online1. Additionally, this study analyzes the travel time difference between the toll road and its alternative routes during the same prediction horizon to investigate the system performance and its predictability from the users' reaction perspective. This paper is organized as follows. Section 2 reviews the related studies of the problem in transportation literature; then, we provide an overview of the study area in section 3. Section 4 explains the data sources used in this study and describe them for the study area. Section 5 provides more details about the methodology we used to address the toll prediction problem; whose numerical results are presented in Section 6. The last section summarizes the study framework and concludes the findings. 2. Literature Review The ever-increasing demand for transportation in urban areas has long been a significant source for the transportation network's reduced service level. Transportation network pricing is a well-known strategy (Lindsey and Verhoef, 2001) and among the most successful travel demand management schemes that aim to use the existing infrastructure more efficiently by internalizing the external costs of congestion (Zhang et al., 2008; Yin and Lou, 2009). In addition to reducing congestion, another advantage of a pricing scheme is generating revenue for transportation authorities, which can be leveraged in improving the transportation supply and specifically the public transportation (Szeto and Lo, 2008; Zhu and Ukkusari, 2015). However, an optimal tolling strategy needs to conform to the users' time-dependent travel demand profile, which mandates a dynamic tolling scheme (Liu et al., 2009). Road pricing strategies can be categorized based on various characteristics, such as the tolling scheme (de Palma and Lindsey, 2011). There are two primary road pricing schemes of cordon pricing and link-based pricing. In cordon pricing, vehicles entering a specified urban area should pay a cost, while in link-based pricing, vehicles traversing a road (or specific lanes of a road) should pay a toll. While cordon pricing is more prevalent in Europe and Asia, link- based pricing is more implemented in the US (Lou et al., 2011). Another approach to categorize road pricing is based on the time differentiation of the toll. Flat, time of day, responsive and anticipatory tolls are examples of such categorization (de Palma and Lindsey, 2011). Responsive and anticipatory dynamic tolling have entered the state of 1 https://vai66tolls.com/ the practice more recently (Yin and Lou, 2009). The examples of such tolling are mostly limited to HOT facilities to maintain free-flow speed. The operator of the HOT lanes in a dynamic tolling strategy regulates the toll price to manage the single- occupancy vehicles (SOV) users of the road, thereby maintaining a desirable level-of-service (LOS) on the facility (Gardner et al., 2013). The SOV users decide whether to pay the toll and reduce travel time or use the regular facilities, typically experiencing a higher travel time. Therefore, the tolling price results from an interaction between the users and the system's operator, which in theory can improve the performance of both HOT and General-purpose (GP) lanes (Zhang et al., 2013). There are various techniques to implement dynamic tolling given the intelligent transportation systems (ITS) advancements in recent years. For a comprehensive up to date literature review of the studies in this area, readers may refer to Saharan et al. (2020). While there are a handful of studies on the theoretical approaches to take for dynamic tolling at the design level, the number of researches on addressing the problems at an operational level is limited. The few studies in this area are mostly focused on descriptive analysis of the traffic condition in the locations with dynamic tolling on the HOT lanes such as I-394 MnPASS (Goodall and Smith, 2010) and I-15 San Diego (Borrell-Rovira and Supernak, 2011). However, to the best of the authors' knowledge, no study in the literature addresses the toll prediction problem. Therefore, this study contributes to the literature in predicting the toll price and travel time difference between the toll facility and the best alternative route for different prediction horizons. There are several aspects of transportation operation and planning that have benefited from predicting the travel behavior and traffic flow characteristics (Sekula et al., 2018; Baee et al., 2019; Ahmed et al., 2020; Taghipour et al., 2020; Zahedian et al., 2020). Similarly, dynamic toll price prediction is beneficial to the users and operator since this variable impacts the travelers' behavior and, subsequently, the operator's response. From a user's viewpoint, this prediction is advantageous in estimating a more accurate cost for her trip, directly impacting the route choice, departure time, and mode choice. From the operator's perspective, providing toll prediction to the users can flatten the corridor's traffic volume profile, thereby reducing traffic congestion.
Details
-
File Typepdf
-
Upload Time-
-
Content LanguagesEnglish
-
Upload UserAnonymous/Not logged-in
-
File Pages13 Page
-
File Size-