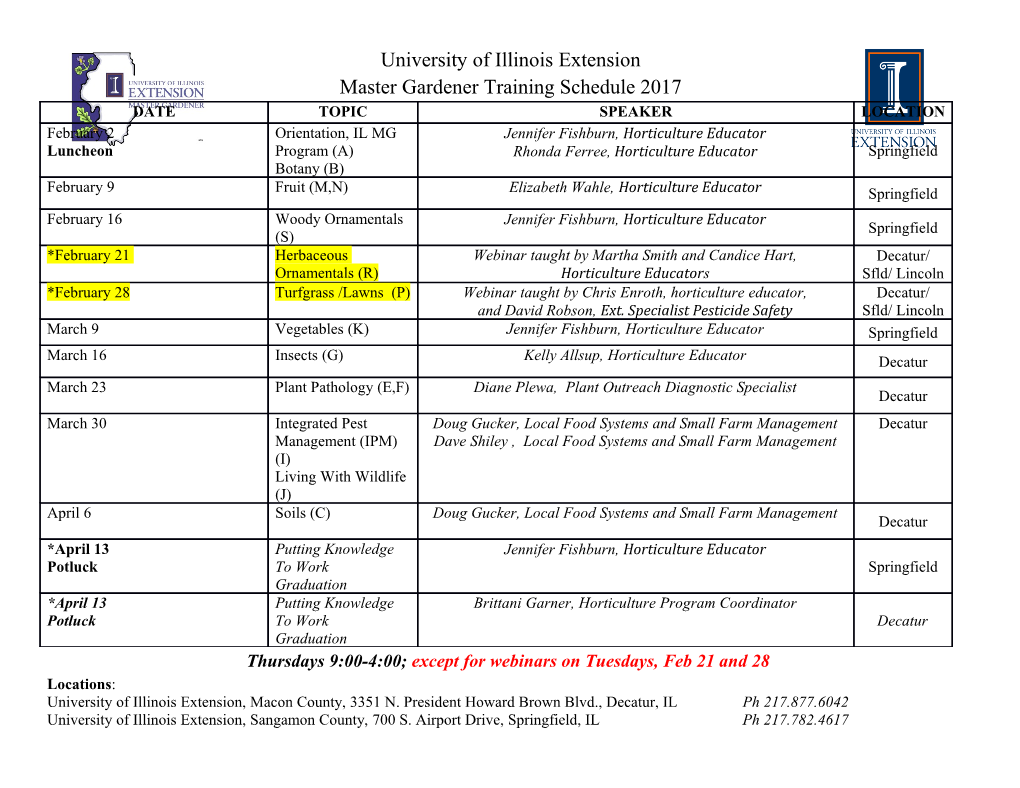
' $ Stochastic Methods in Electrostatics: Applications to Biological and Physical Science Michael Mascagni a Department of Computer Science and School of Computational Science Florida State University, Tallahassee, FL 32306 USA E-mail: [email protected] URL: http://www.cs.fsu.edu/»mascagni Research supported by ARO, DoD, DOE, NATO, and NSF aWith help from Drs. James Given, Chi-Ok Hwang, and Nikolai Simonov & % ' $ Outline of the Talk First-Passage Algorithms ² Walk on Spheres (WOS) ² Greens Function First Passage (GFFP) ² Simulation-Tabulation (S-T) ² Walk on Subdomains (biochemistry) ² Walk on the Boundary Applications ² Materials Science ² Biochemistry Last-Passage Algorithms Conclusions and Future Work & % Prof. Michael Mascagni: Stochastic Electrostatics Slide 1 of 56 ' $ Stochastic Methods for Partial Di®erential Equations (PDEs) Examples for Solving Elliptic PDEs (Path Integrals) ² Exterior Laplace problems and electrostatics ² Electrical capacitance ² Charge density Advantages of Stochastic Algorithms (Curse of Dimensionality) ² Can avoid complex discrete objects ² Can deal with complicated geometries/interfaces ² Can often cope with singular solutions & % Prof. Michael Mascagni: Stochastic Electrostatics Slide 2 of 56 ' $ Brownian Motion and the Di®usion/Laplace Equations Cauchy problem for the di®usion equation: 1 u = ¢u (1) t 2 u(x; 0) = f(x) (2) in 1-D: Z 1 u(x; t) = !(x ¡ y; t)f(y)dy (3) ¡1 where 2 1 ¡ (x¡y) !(x ¡ y; t) = p e 2t (4) 2¼t & % Prof. Michael Mascagni: Stochastic Electrostatics Slide 3 of 56 ' $ Brownian Motion and the Di®usion/Laplace Equations x u(x; t) = Ex[f(X (t))] (5) ² Xx(t): a Brownian motion which has !(x ¡ y; t) as the transition probability of going from x to y in time t ² Ex[:]: an expectation w.r.t. Brownian motion Z 1 x Ex[f(X (t))] = !(x ¡ y; t)f(y)dy (6) ¡1 & % Prof. Michael Mascagni: Stochastic Electrostatics Slide 4 of 56 ' $ The First Passage (FP) Probability is the Green's Function A related elliptic boundary value problem (Dirichlet problem): ¢u(x) = 0; x 2 ­ u(x) = f(x); x 2 @­ (7) ² Distribution of z is uniform on the sphere ² Mean of the values of u(z) over the sphere is u(x) ² u(x) has mean-value property and harmonic ² Also, u(x) satis¯es the boundary condition x u(x) = Ex[f(X (t@­))] (8) & % Prof. Michael Mascagni: Stochastic Electrostatics Slide 5 of 56 ' $ The First Passage (FP) Probability is the Green's Function Ω @ ª Ü Ü X ´Øµ X ´Ø µ ª z @ first−passage location x; starting point & % Prof. Michael Mascagni: Stochastic Electrostatics Slide 6 of 56 ' $ The First Passage (FP) Probability is the Green's Function (Cont.) Reinterpreting as an average of the boundary values Z u(x) = p(x; y)f(y)dy (9) @­ Another representation in terms of an integral over the boundary Z @g(x; y) u(x) = f(y)dy (10) @­ @n g(x; y) { Green's function of the Dirichlet problem in ­ @g(x; y) =) p(x; y) = (11) @n & % Prof. Michael Mascagni: Stochastic Electrostatics Slide 7 of 56 ' $ `Walk on Spheres' (WOS) and Green's Function First Passage (GFFP) Algorithms ² Green's function is known =) direct simulation of exit points and computation of the solution through averaging boundary values ² Green's function is unknown =) simulation of exit points from standard subdomains of ­, e.g. spheres =) Markov chain of `Walk on Spheres' (or GFFP algorithm) fx0 = x; x1;:::g xi ! @­ and hits "-shell is N = O(ln(")) steps xN simulates exit point from ­ with O(") accuracy & % Prof. Michael Mascagni: Stochastic Electrostatics Slide 8 of 56 ' $ `Walk on Spheres' (WOS) and Green's Function First Passage (GFFP) Algorithms WOS: O Shell thickness ε & % Prof. Michael Mascagni: Stochastic Electrostatics Slide 9 of 56 ' $ Timing of the 'Walk on Spheres' Algorithm 3500 3000 2500 2000 running time (secs) 1500 1000 logarithmic regression 500 10−8 10−7 10−6 10−5 10−4 10−3 10−2 10−1 100 ε & % Prof. Michael Mascagni: Stochastic Electrostatics Slide 10 of 56 ' $ Various Laplacian Green's Functions: GFFP O O O (a) Putting back (b) Void space (c)Intersecting & % Prof. Michael Mascagni: Stochastic Electrostatics Slide 11 of 56 ' $ Geometry for Permeability Computations & % Prof. Michael Mascagni: Stochastic Electrostatics Slide 12 of 56 ' $ The Simulation-Tabulation (S-T) Method for Generalization ² Green's function for the non-intersected surface of a sphere located on the surface of a reflecting sphere Absorbing Sphere Ω 2 r 2 Ω 1 O2 r 1 Reflecting Sphere O1 & % Prof. Michael Mascagni: Stochastic Electrostatics Slide 13 of 56 ' $ Example: Solc-Stockmayer Model without Potential Reactive patch θ R R0 & % Prof. Michael Mascagni: Stochastic Electrostatics Slide 14 of 56 ' $ Another S-T Application: Mean Trapping Rate In a domain of nonoverlapping spherical traps : δ O & % Prof. Michael Mascagni: Stochastic Electrostatics Slide 15 of 56 ' $ Biological Electrostatics: Motivation Electrostatics are Extremely Important in Quantitative Biochemistry: ² Ligand binding ² Protein-protein interactions ² Protein-nucleic acid interactions ² Prediction from primary structure information In Vivo Electrostatics Must Include the Solvent ² Explicit solvent model: individual water/ions computed, often with Molecular Dynamics ² Implicit solvent models: continuum model of water and dissolved ions used, Poisson-Boltzmann equation & % Prof. Michael Mascagni: Stochastic Electrostatics Slide 16 of 56 ' $ Molecular Electrostatics Problem Implicit solvent model ² Poisson equation for the electrostatic potential, Á: ¡r²(x)rÁ(x) = 4¼½(x) ; x 2 R3 dielectric permittivity, ², and charge density, ½, are position-dependent 3 ² molecule ­ { a compact cavity in R with low ² = ²i ² surrounded by solvent with larger ² = ²e ² point charges, qm, at xm inside molecule ² Boltzmann distribution of mobile ions in solvent & % Prof. Michael Mascagni: Stochastic Electrostatics Slide 17 of 56 ' $ Molecular Electrostatics Problem (Cont.) Explicit geometric models for solute molecule ² van der Waals surface: SM (m) (m) union of intersecting spheres (atoms): ­ = m=1 B(x ; r ) (m) point charges { at their centers, xm = x ² contact and reentrant surface, ¡: @­ smoothed by the probe molecule of the solute rolling on it ² ion-accessible surface @­0: 0 SM (m) (m) ­ = m=1 B(x ; r + rion); ion-exclusion layer between @­0 and ¡ & % Prof. Michael Mascagni: Stochastic Electrostatics Slide 18 of 56 ' $ Molecular Electrostatics Problem (Cont.) Mathematical model (one-surface geometry) ² Poisson equation for the electrostatic potential, Á, inside a molecule XM ¡²i¢Ái(x) = 4¼qm±(x ¡ xm) ; x 2 ­ m=1 ² linearized Poisson-Boltzmann equation outside, x 2 R3 n ­: 2 ¢Áe(x) ¡ · Áe(x) = 0 ; ² Continuity condition on the boundary @Á @Á Á = Á ; ² i = ² e ; y 2 ¡ ´ @­ i e i @n(y) e @n(y) & % Prof. Michael Mascagni: Stochastic Electrostatics Slide 19 of 56 ' $ Electrostatic Potential, Field and Energy (Linear Problem) ² Point values of the potential: Á(x) = Á(0)(x) + g(x) Here, singular part of Á: XM q 1 g(x) = m ² jx ¡ x j m=1 i m ² Free electrostatic energy of a molecule = linear combination of point values of the regular part of the electrostatic potential Á(0): 1 XM E = Á(0)(x )q ; 2 m m m=1 ² Point values of the electrostatic ¯eld: rÁ(x) & % Prof. Michael Mascagni: Stochastic Electrostatics Slide 20 of 56 ' $ Monte Carlo Estimates for Point Potential Values Two di®erent approaches to constructing Monte Carlo algorithms 1. Probabilistic representation for the solution 2. Classical potential theory First approach Laplace equation for the regular part of the potential inside ­ ¢Á(0) = 0 Probabilistic representation (0) (0) ¤ Á (x) = Ex[Á (x )] x¤ { exit point from ­ of Brownian motion starting at x & % Prof. Michael Mascagni: Stochastic Electrostatics Slide 21 of 56 ' $ `Walk on Spheres' Algorithm x { center of a sphere ) exit points are distributed isotropically. Ball B(x; R) lies entirely in ­. Strong Markov property of Brownian motion ) probabilistic representation holds valid for exit points. Hence follows `random walk on spheres' algorithm for general domains with regular boundary: xk = xk¡1 + d(xk¡1) £ !k ; k = 1; 2;:::: Here d(xk¡1) { distance from xk¡1 to the boundary f!kg { sequence of independent unit isotropic vectors xk is exit point from the ball, B(xk¡1; d(xk¡1)), for Brownian motion starting at xk¡1 & % Prof. Michael Mascagni: Stochastic Electrostatics Slide 22 of 56 ' $ Green's Function First Passage Simulation Other domains with known Green's function (G) () one-step simulation of exit points distributed on the boundary in accordance with @G=@n For general domains: E±cient way to simulate x¤ { combination of `walk in subdomains' approach and `walk on spheres' algorithm The whole domain, ­, is represented as a union of intersecting subdomains: [M ­ = ­m m=1 Simulate exit point separately in every ­m & % Prof. Michael Mascagni: Stochastic Electrostatics Slide 23 of 56 ' $ Green's Function First Passage Simulation (cont.) x0 = x; x1; : : : ; xN { Markov chain, every xi+1 is exit point from the corresponding subdomain for Brownian motion starting at xi i i For spherical subdomains, B(xm;Rm), exit points are distributed in accordance with the Poisson's kernel i i i i 2 i jx ¡ xmj jx ¡ xmj ¡ Rm i i 3 4¼Rm jx ¡ yj x¤ = xN is exit point of Brownian motion from ­ Schwartz lemma ) Markov chain fxig converges to x¤ geometrically & % Prof. Michael Mascagni: Stochastic Electrostatics Slide 24 of 56 ' $ `Walk on Spheres' and `Walk in Subdomains' Algorithms Figure 1: Walk in subdomains example. & % Prof. Michael Mascagni: Stochastic Electrostatics Slide 25 of 56 ' $ Monte Carlo Estimate for Point Potential Value On every step Á(0)(xi) = E[Á(0)(xi+1)jxi] Hence Á(0)(x) = EÁ(0)(x¤) ´ E[Á(x¤) ¡ g(x¤)] Values of the electrostatic potential on the boundary, Á(x¤), are not known. We can use their Monte Carlo estimates instead & % Prof. Michael Mascagni: Stochastic Electrostatics Slide 26 of 56 ' $ Monte Carlo Estimate for Boundary Potential Values ² First approach Discretization and randomization of the boundary condition (y 2 ¡, n = n(y) { normal vector); 2 Á(y) = piÁ(y ¡ hn) + peÁ(y + hn) + O(h ) =) ¤ 0 ¤ 2 Á(x1) = E(Á(x2jx1) + O(h ) 0 ¤ x2 = x1 ¡ hn with probability pi (reenter molecule) 0 ¤ x2 = x1 + hn with probability pe = 1 ¡ pi (exit to solvent) ²i pi = ²i + ²e & % Prof.
Details
-
File Typepdf
-
Upload Time-
-
Content LanguagesEnglish
-
Upload UserAnonymous/Not logged-in
-
File Pages57 Page
-
File Size-