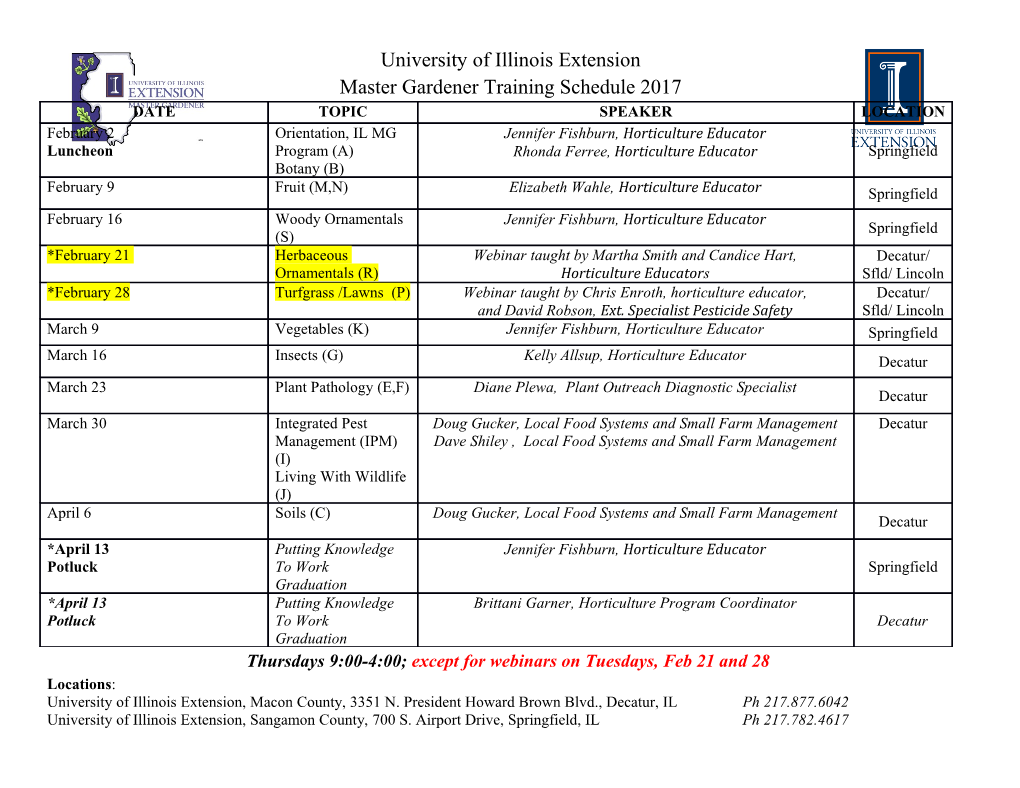
Prediction of Properties of Organic Compounds – Empirical Methods and Management of Property Data Den Naturwissenschaftlichen Fakultäten der Friedrich-Alexander-Universität Erlangen-Nürnberg zur Erlangung des Doktorgrades vorgelegt von Thomas Kleinöder aus Marburg/Lahn Als Dissertation genehmigt von den Naturwissenschaftlichen Fakultäten der Universität Erlangen-Nürnberg Tag der mündlichen Prüfung: 12.12.2005 Vorsitzender der Promotionskommission: Prof. Dr. D.-P. Häder Erstberichterstatter: Prof. Dr. J. Gasteiger Zweitberichterstatter: Prof. Dr. T. Clark Meinem Doktorvater Herrn Prof. Dr. Johann Gasteiger danke ich für die vielfältige Unterstützung und die wertvollen Anregungenen, ohne die diese Arbeit nicht möglich gewesen wäre. Mein besonderer Dank gilt weiterhin: Dr. Lothar Terfloth für die vielfältigen Diskussionen zu allen Bereichen der in dieser Arbeit behandelten Themen und die stete Hilfsbereitschaft, Dr. Achim Herwig, Jörg Marusczyk und den anderen Entwicklern von MOSES für die gute Zusammenarbeit und die manchmal kontroversen aber immer produktiven Diskussionen, den Administratoren der verschiedenen Betriebssystem-Plattformen und des Netzwer- kes für die Bereitstellung und Aufrechterhaltung einer stabilen Arbeitsumgebung, Dr. Nico van Eikema Hommes und Prof. Dr. Tim Clark für die hilfreichen Diskussio- nen zu Fragen der Berechnung quanten-chemischer Atomladungen, Christoph Schlenker für die hilfreichen Diskussionen und Anregungen zu allen Be- reichen der Software-Entwicklung, Angela Döbler, Ulrike Scholz und Karin Holzke für die Unterstützung bei allen admi- nistrativen Aufgaben, allen nicht genannten Kollegen des Arbeitskreises für die freundliche Aufnahme, viele fruchtbare Diskussionen und die positive und produktive Arbeitsatmosphäre. Mein weiterer Dank gilt dem Bundesministerium für Bildung und Forschung für die finan- zielle Unterstützung im Rahmen der Forschungsprojekte „Suche und Optimierung von Leit- strukturen (SOL)” und „Bioinformatics for the Functional Analysis of Mammalian Genomes (BFAM)”. Insbesondere danke ich meiner Frau, Diana Kleinöder, die mir immer Unterstützung und Verständnis beim Erstellen dieser Arbeit entgegengebracht hat. Für Diana, Karla und meine Eltern . Contents 1 Introduction 1 2 Fundamentals and Methods 5 2.1 Empirical Approaches to the Prediction of Properties . 5 2.1.1 Linear Free–Energy Relationships (LFER) . 5 2.1.2 Quantitative Structure–Property Relationships (QSPR) . 7 2.2 Structure Descriptors . 9 2.3 Multivariate Data Analysis . 12 2.3.1 Multivariate Data . 12 2.3.2 Feature Selection and Transformation . 13 2.4 Learning Methods . 14 2.4.1 Unsupervised Learning . 15 2.4.2 Supervised Learning . 15 2.5 Model Building . 19 2.6 Parametrization . 21 2.7 Charge Calculation by Partial Equalization of Electronegativity . 23 2.7.1 The Concept of Electronegativity and its Equalization . 24 2.7.2 Partial Equalization of Orbital Electronegativity (PEOE) . 27 2.7.3 Partial Equalization of π-Electronegativity (PEPE) . 32 2.8 Software Development . 35 2.8.1 Programming Paradigms . 35 2.8.2 Design Techniques . 40 2.8.3 Techniques for Implementation and Maintenance . 44 3 MOSES 45 i ii CONTENTS 3.1 Introduction . 45 3.2 From Script-based to Integrated Workflows . 47 3.3 Overcoming Limitations of Existing In-house Libraries . 50 3.4 Representation of Chemical Structures . 51 3.4.1 Connection Tables . 53 3.4.2 RAMSES – A Structure Representation Based on σ/π Separation . 54 3.4.3 Extension to Hypervalent Atom Types . 57 3.4.4 Perception of Aromaticity based on RAMSES . 60 3.5 Architecture and Implementation . 64 3.6 Management of Properties . 69 3.6.1 Analysis . 70 3.6.2 Design and Implementation . 71 3.7 Current Status of the Calculator Sublibrary . 81 3.8 Applications . 82 3.8.1 MOSES::WORKFLOWMANAGER . 82 3.8.2 Polarizabilities Through a Zero Order Additivity Scheme . 84 4 Quantification of Atomic Partial Charges 89 4.1 Introduction . 89 4.2 Development of a Combined PEOE/HMO Calculation . 91 4.2.1 Substitution of PEPE by a Modified Hückel MO Treatment . 91 4.2.1.1 Hückel MO Theory . 92 4.2.1.2 Modified HMO: Accounting for the Inductive Effect . 98 4.2.1.3 Hyperconjugation . 101 4.2.2 Datasets . 102 4.2.3 The Problem of Parametrization . 106 4.2.4 Observable Properties . 107 4.2.5 Charges from Quantum Mechanical Calculations . 111 4.2.5.1 Methods . 112 4.2.5.2 Requirements for a Reference Charge Calculation Scheme 115 4.2.6 Analysis and Comparison of QM Charges . 116 4.2.6.1 Rates of Failures for QM Charge Calculation Methods . 116 4.2.6.2 Direct Comparison of Charges from QM Calculations . 117 CONTENTS iii 4.2.6.3 Dipole Moments from QM Charges . 119 4.2.6.4 Distribution of Charges for Various Atom Types . 121 4.2.6.5 Relationship to Electronegativity . 129 4.2.6.6 Shortcomings of the Merz-Kollman Scheme . 132 4.2.6.7 Conclusions of the Analysis . 133 4.2.7 Summary . 135 4.3 Workflow and Procedure for the Parametrization . 136 4.4 Calibration with Molecular Dipole Moments . 138 4.4.1 PEOE . 140 4.4.2 Modified HMO . 144 4.5 Calibration with DFT/NPA Charges . 148 4.5.1 General Considerations . 148 4.5.2 Modified PEOE . 149 4.5.2.1 Revised Electronegativity Parameters . 150 4.5.2.2 Negative Hyperconjugation . 153 4.5.3 Modified HMO . 159 4.5.4 Results of the Parametrization . 166 4.6 Evaluation . 177 4.6.1 C-1s ESCA Shifts . 177 4.6.2 π-Charges . 178 4.7 Summary . 181 5 Summary 183 6 Zusammenfassung 185 A Supplementary Material 189 A.1 To Section 4 . 189 B Datasets 195 B.1 To Section 3 . 195 B.2 To Section 4 . 202 Bibliography 217 . Table of Symbols and Abbreviations Abbreviations and Acronyms QSPR Quantitative Structure–Property Relationships QSAR Quantitative Structure–Activity Relationships LFER Linear Free–Energy Relationships PEOE Partial Equalization of Orbital Electronegativities MPEOE Modified Partial Equalization of Orbital Electronegativities PEPE Partial Equalization of Pi-Electronegativities HMO Hückel Molecular Orbital (theory) MHMO Modified Hückel Molecular Orbital (method) PEOE/MHMO(µ) combined PEOE/MHMO procedure fitted to molecular dipole moments MPEOE/MHMO(NPA) combined MPEOE/MHMO procedure fitted to DFT/NPA charges DFT Density Functional Theory NPA Natural Population Analysis MPA Mulliken Population Analysis AIM Atoms In Molecules (method) MK-ESP Merz-Kollman-Electrostatic-Potential (derived charges) RICOS Representation of Inorganic, Coordinative, and Organic Structures MOSES Molecular Structure Encoding System RAMSES Representation Architecture for Molecular Structures by Electron Systems CORINA Coordinates CACTVS Chemical Algorithms Construction, Threading, and Verification System UML Unified Modeling Language OOP Object Oriented Programming SP Structured Programming GP Generic Programming SA Simulated Annealing GA Genetic Algorithm Physicochemical Quantities and Units property symbol unit mean molecular polarizabilities α¯ [Å3] atomic partial charges q [e] electronegativities χ [eV] dipole moments µ [debyes] ([D]) ESCA shifts – [eV] Chapter 1 Introduction Organic chemistry deals with understanding the mechanisms of organic reactions and with planning and performing the synthesis of organic compounds. The chemical structure of a compound is under consideration, its construction from simple structural building blocks by a synthesis strategy or its modification through the application of given reaction types. Tightly related to the chemical structure of a compound are its properties. As has been stated by George S. HAMMOND "the most fundamental and lasting objective of synthesis is not production of new compounds, but production of properties" (Norris Award Lecture, 1968). Thus, even though we deal with the chemical structure as a necessary abstract representa- tion, the fundamental physicochemical properties of a compound are of central concern and determine its behavior in chemical, biochemical or environmental processes. Having a compound or a set of compounds at hand, the property of interest can be mea- sured experimentally if an appropriate analytical method is available. However, experimental measurements are often tedious and time-consuming and may fail. With some fortune, an experimental value might be found in one of the available data bases (e.g., [1, 2]). Yet, one is likely to fail in finding the requested property considering the number of compounds available and the number of entries in a typical data base. For in- stance, the octanol-water partition coefficient is the property having the most entries (13250) in the PHYSPROP data base [2]. Compared with the number of compounds currently reg- istered in the CAS data base [3], about 26 million, it becomes obvious that the chance of finding an experimental value of a property for a given compound is quite low. The demand for computational methods for the prediction of properties of compounds is therefore evident. 1 Chapter 1 The basic approach to the problem of predicting properties can be written in a very simple form that states that a molecular property P can be expressed as a function of the molecular structure C P = f(C) (1.1) The function f(C) may have a very simple form, as is the case for the calculation of the molecular mass from the relative atomic masses. In most cases, however, f(C) will become more complicated, for instance, when it comes to describe the structure by quantum mechan- ical means and the property of interest may be derived from the wavefunction; for example the dipole moment may be obtained by applying the dipole moment operator. If we can describe the system of interest by first principles based on the theory of quan- tum mechanics no other information is required and if the level of theory is sufficient we can calculate the property of interest ab initio with good accuracy [4]. This approach to predicting properties is called deductive learning. It is only limited by the ability to handle the underlying mathematics in order to solve the Schrödinger equation and to find a way to derive the property of interest from the wavefunction. Even though the computational power of current hardware has increased dramatically over the last decades, ab initio calculations on a high level of accuracy are still restricted to medium-sized molecules and small datasets.
Details
-
File Typepdf
-
Upload Time-
-
Content LanguagesEnglish
-
Upload UserAnonymous/Not logged-in
-
File Pages247 Page
-
File Size-