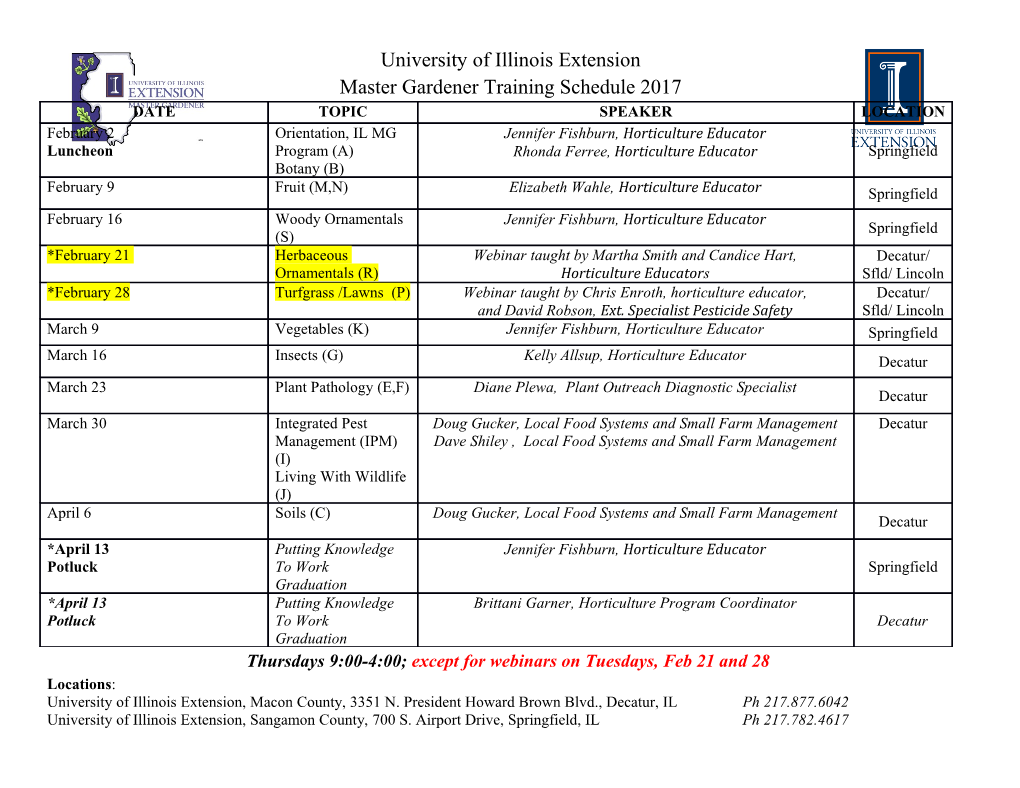
Evolution of Test Cricket in Last Six Decades A Univariate Time Series Analysis Mayank Nagpal Sumit Mishra 1 Introduction We intend to analyse the structural changes in the average annual run-rate, i.e., how many runs are scored in each over, a measure of how much bat dominates the ball or how aggressively teams bat. Test cricket is the traditional format of the game. It is considered to be a snail-form of the game when we compare it with newer versions of the game, viz, ODI and T20 .A test cricket match lasting 5 days is apparently a lot less exciting for some than an ODI which lasts for eight hours or a T20 match which is matter of two-three hours. The general view is that with the advent of new technology, pitches that are more batsmen-friendly and craving for result in each game, the average run rate seems to have increased. Some analysts attribute this change to the emergence of newer formats and other innovations in the game. The question we are trying to answer is about whether these factors like those mentioned below had any significant impact on the game. The events whose effect we like to capture are: • Advent of One Day International(ODI):With dying popularity of test cricket matches during 1960s,a tournament called Gillette Cup was played in 1963 in England. The cup had sixty- five overs a side matches. This tourney was a knockout one and it became quite popular and laid foundation for a sleeker format of the game known as ODI-fifty overs a side game. The advent of ODI in 1971 is considered to be a turning point in the history of the game; • Kerry Packer circus: Kerry Packer was an Australian media baron who formed a rebel league of cricket known as World Series Cricket in 1977 which changed the nature of the game being played forever; • In 2003, a new and vibrant Twenty 20 format was introduced.The format of Twenty20 closely resembles a form of the game that has been popular in English amateur cricket since 1960.It is expected to increase interest in the game by pulling more people towards cricket as a whole. In recent times with high scores being chased easily in all formats of the game, it is being said that this is due to the effect of T20 games on other formats of the game. We plan to model and empirically test for this effect. We apply time series analysis to answer the question.We perform a similar analysis for two other series i.e. the proportion of matches drawn in a year and the variance of the run rate for a year. 2 Data The annual data for the paper is downloaded from the ESPN Cricinfo website. We use annual data for average run rate, variance of run rates for the year and the proportion of matches drawn for every year from 1946. Although we had data from the year 1877, we use only the post World War-II data as very few matches were played before this period leaving us with only 63 data points. 1 As there are factors other than what we plan to model affecting the runs per over series such as the weather conditions, type of teams playing, condition on the pitch, etc., an innings by innings study of the series would not have been feasible. To nullify the effect we converted the data into an annual series by taking the average for an entire year. As matches are played throughout the year and in all weather conditions, we hope to nullify the effects of these factors by averaging out the data. Also, there are other factors such as the changing nature of the pitches and the kind of equipment used which might affect the average runs per over in a year. We plan to perform this time series analysis for test matches as well as for one day internationals and study whether different events have affected the game and how cricket has changed over the period of 60 years. Table-I Summary Statistics Run Rate Variance Proportion Of Matches Drawn Mean 2.78 0.66 38.23 Median 2.77 0.49 36.11 Standard Error 0.04 0.12 1.79 Standard Deviation 0.33 1 14.2 Sample Variance 0.11 1 201.7 Kurtosis -0.47 52.97 -0.33 Skewness 0.1 7.02 0.54 Range 1.44 7.98 63.89 Minimum 2.04 0.19 11.11 Maximum 3.48 8.17 75 Sum 174.86 41.71 2408.77 Data Points 63 63 63 3 Analysis of Run-Rate Series The time plot suggests that the average annual run rate remained lower than the average till 1976 after which it hovered around the average peaking rarely until 2001. There seems to be a positive trend in the series, i.e. the value seems to increase with time. In post 2001 period the average run rate has remained pretty high(consistently above 3).It seems that there is a structural change in the run-rate in late 1950s and mid-1960s,late 1970s and then in 2000. We will apply proper analytical tests for this hypothesis to ascertain whether structural break exists. 2 Time Plot of Run Rate ACF&PACF Plot for Run Rate Series There appears to be a clear dependence on past values looking at the ACF and PACF of the series. Also, looking at the time plot there appears to be a dependence on time for the run rate series. The series appears to be non-stationary as there is a slow decay in the value of the ACFs.To test for a stationary trend in the series,we regress run rate on time and get the following result. 3 Dependent Variable:Run Rate Independent Variable Estimate Standard Error p-value Intercept* 2.281589 0.042254 2e-16 Time* 0.015438 0.001148 2e-16 The regression confirms our suspicion that there is a trend in the run-rate series.We would be testing, using the structural break test, whether the introduction of the new formats made any significant difference in the rise. The positive coefficient on time indicates a positive trend in the series. We remove this trend by detrending the data and get the new series which looks like what we have plotted in the figure below. ACF and PACF of this detrended series are also stacked along with the given time plot. Looking at the plots of the ACF are PACF we can predict the models for the de-trended run rate series to be either AR (1), AR(4) or ARMA(2,1). To find the best fitting model we calculate the AIC, SBC for various order ARMA models and test if the residuals for these models are white noise. This test is essential for our analysis as any model which does not have white noise error terms is said to be a misspecified model. The results show that the model with lowest values of SBC and AIC is AR(1). Dependent Variable:Detrended Run Rate[AR(1)] Independent Variable Estimate Standard Error Intercept 0.0031 0.0370 AR (1) [t−1∗] 0.5442 0.1042 To test for GARCH (Generalised Auto Regressive Conditional Heteroscedasticity) effects for the run-rate series we use the residuals and residual squared series of the model and plot these errors along with their ACFs and PACFs. 4 The ACF and PACF plots of the residual squared term do not point to any ARCH/GARCH effects in the series. Moreover, when we ran the test for SBC and AIC values (Given in the Ap- pendix), ARMA (0,0) turned out to be the DGP that represents the residual squared series. Thus, the estimated model for the run-rate series is as given below: Xt = 2.28 + 0.015t + φ(L)t + ωt (1) where φ(L) = 1 − 0.54L (2) and ωt ∼ N(0, 0.019) (3) Xt = 2.28 + 0.015t + 0.54t−1 + t (4) The equation implies that the run rate for a year depends on the previous years innovations, i.e. if for some reason the run rate for some year is higher than expected or higher than the normal run rate due to some innovations in the game, it would be high for the next year as well. Thus, if any innovation (for instance new and improved bats) introduced in the game causes an increase in the rate of runs scored,there will be persistence. To answer our question that whether structural break exists, we need to test the hypothesis that there is no structural break at the specified points in the series. We perform the Chow test to look for structural breaks in the three series. For the first series we calculate the F statistic and plot it for all values. We perform the test separately for the regression of Run Rate on time and for the AR(1) model of the de-trended series. The red lines represent the critical values for a 95% confidence interval. It can be seen that the F statistic is greater than the critical value for time 10 to 16 i.e. from the years 1956 to 1962, reaching a peak at time =12, i.e. 1958. The plot suggests that the Chow test accepts (does not reject) our hypothesis of no structural breaks in the seasons 1970-71, 1977-78 and 2003-04.For the detrended run-rate series, the F-statistics values sit perfectly below the red line indicating absence of any structural break in the data.
Details
-
File Typepdf
-
Upload Time-
-
Content LanguagesEnglish
-
Upload UserAnonymous/Not logged-in
-
File Pages21 Page
-
File Size-