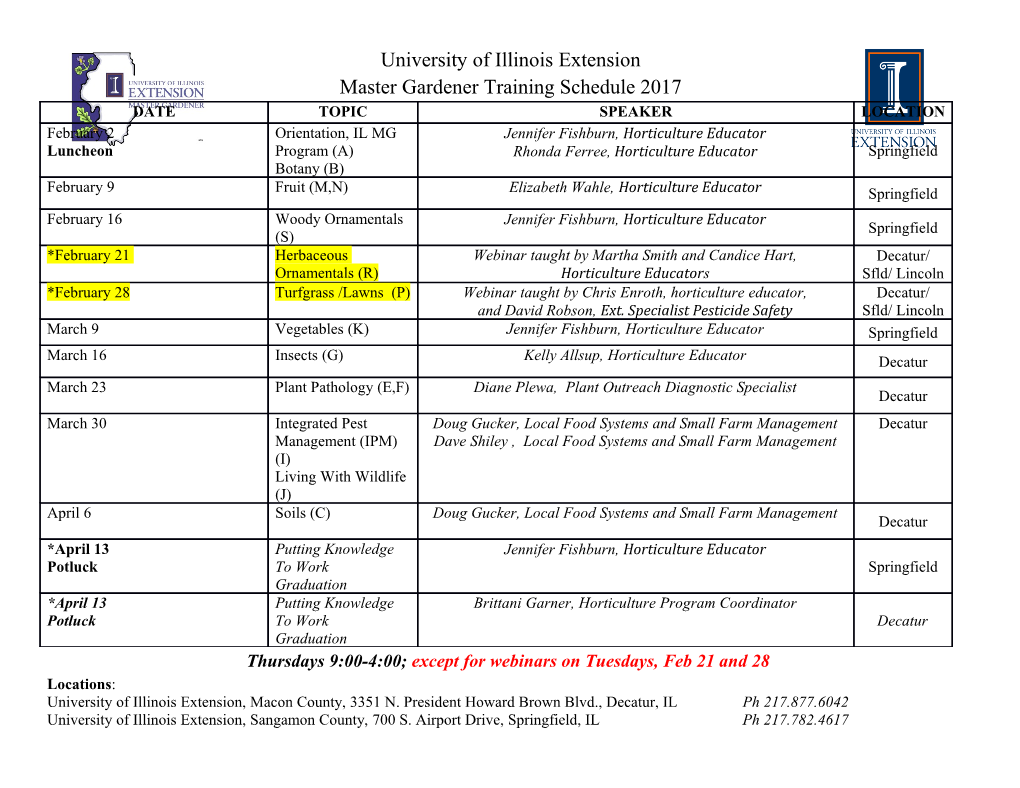
ULTIMATE TIC-TAC-TOE Scott Powell, Alex Merrill Professor: Professor Christman An algorithmic solver for Ultimate Tic-Tac-Toe May 2021 ABSTRACT Ultimate Tic-Tac-Toe is a deterministic game played by two players where each player’s turn has a direct effect on what options their opponent has. Each player’s viable moves are determined by their opponent on the previous turn, so players must decide whether the best move in the short term actually is the best move overall. Ultimate Tic-Tac-Toe relies entirely on strategy and decision-making. There are no random variables such as dice rolls to interfere with each player’s strategy. This is rela- tively rare in the field of board games, which often use chance to determine turns. Be- cause Ultimate Tic-Tac-Toe uses no random elements, it is a great choice for adversarial search algorithms. We may use the deterministic aspect of the game to our advantage by pruning the search trees to only contain moves that result in a good board state for the intelligent agent, and to only consider strong moves from the opponent. This speeds up the efficiency of the algorithm, allowing for an artificial intelligence capable of winning the game without spending extended periods of time evaluating each potential move. We create an intelligent agent capable of playing the game with strong moves using adversarial minimax search. We propose novel heuristics for evaluating the state of the game at any given point, and evaluate them against each other to determine the strongest heuristics. TABLE OF CONTENTS 1 Introduction1 1.1 Problem Statement............................2 1.2 Related Work...............................3 2 Methods6 2.1 Simple Heuristic: Greedy.........................6 2.2 New Heuristic...............................7 2.3 Alpha- Beta- Pruning and Depth Limit.................. 10 3 Results 12 4 Discussion 13 5 Conclusion 14 Bibliography 15 ii LIST OF FIGURES 1.1 Example of a game move. Players alternate placing marks, and each player’s mark determines which board their opponent will place a mark next. In this figure, One player has placed a circle in the top-left corner of the center board. The second player must then place their next mark on the top-left board (outlined with a square)...............3 1.2 Complete game of Ultimate Tic-Tac-Toe. O has three boards in a row, winning the game.............................4 1.3 There is no winning strategy that involves winning the bottom-left board for any player. All moves should be focused on winning one of the two other remaining boards, but previous heuristics would determine that winning the bottom-left board is still a good move............5 2.1 Each cell is assigned a unique number that can be used to specify a move. Each turn there is a range of valid moves, so if the player must move in the center board, they are prompted for a number between 36 and 44...................................7 iii CHAPTER 1 INTRODUCTION Ultimate Tic-Tac-Toe (UTTT) is a deterministic board game with perfect informa- tion. Each move requires the consideration of nine individual Tic-Tac-Toe boards. While traditional Tic-Tac-Toe (TTT) has been solved thoroughly such that the best moves are almost universally known, the ultimate variant adds enough variables that the game is more similar to popular games like Chess or Checkers than the single board version of TTT. Each player’s turn determines which board their opponent will place a mark on, adding additional levels of depth to decision making. Deterministic games are interesting to study because people have an innate curiosity for finding optimal moves in board games, and Artificial intelligence algorithms are ca- pable of processing millions of game scenarios to evaluate moves at a level humans can’t compete with. Additionally computers may be used as a tool by people looking to im- prove their gameplay. Players can select multiple difficulty levels to simulate opponents that provide the best amount of challenge. Many games, including Chess, Checkers, Gomoku, and Blokus, already have algorithms capable of finding optimal moves, but there is very little research on UTTT [4]. We propose a minimax algorithm that plays strong moves using a novel evaluation function. UTTT is an interesting problem for artificial intelligence because it is one of the few deterministic games with perfect information. This means that given the valid moves for a turn, one of the moves must result in a higher chance of winning compared to the others. It is exceedingly difficult for a human to determine the best move, as it depends on up to 80 subsequent moves. Hence, UTTT is a problem that humans are incapable of fully understanding, but a computer has no problem calculating the many potential board states that result from moves. There are other games that have similar rules (For example: Chess, Checkers, and Gomoku), but they have received substantially more 1 research. Thus, UTTT is a problem AI is well-equipped to solve, but there aren’t many intelligent agents capable of playing it. 1.1 Problem Statement The Ultimate Tic-Tac-Toe board is composed of nine regular Tic-Tac-Toe boards, ar- ranged in a 3x3 grid. This grid is stylized to look like one large Tic-Tac-Toe board, with each grid on the board filled with an empty TTT board. These boards are played simul- taneously. As in traditional TTT, player’s alternate selecting a cell to mark. However, in UTTT each mark also determines which of the nine TTT boards the opponent’s next move will be played in. If on a turn player 1 is permitted to place a mark in the center board in the 3x3 Tic-Tac-Toe grid, and they choose to place a mark in the top-left corner of that board, their opponent’s next move would need to be on the top-left board of the 3x3 grid (See Figure 1.1). Play continues in this fashion until a player has one a board on the 3x3 grid. There is some debate about how players handle winning a small board. For example, what happens when a player places a mark that would result in their opponent’s next move being in a board that has already been won? Early variants used a rule that would require the opponent to place marks in the already won board, to no effect [2], and if no moves are available the opponent may place a mark on any of the nine boards, in any (unfilled) position. Moves that may go on any board are known as wildcards. It has been proven that player 1 has a strategy to win the game in at most 43 moves if wildcards are only permitted if no valid move exists [2]. An alternative variation allows wildcards if a player’s next move is in an already won board [3]. To our knowledge there is no guaranteed winning solution in this variation. This makes it an ideal problem for an intelligent agent to solve. When one player wins three boards in a row, they win the game (See Figure 1.2). 2 Figure 1.1: Example of a game move. Players alternate placing marks, and each player’s mark determines which board their opponent will place a mark next. In this figure, One player has placed a circle in the top-left corner of the center board. The second player must then place their next mark on the top-left board (outlined with a square). We are focusing on Minimax Search Trees to create an intelligent agent capable of playing Ultimate Tic-Tac-Toe at a high level. We propose a new minimax evaluation function that is capable of beating human players. 1.2 Related Work To our knowledge, there is only one published paper on UTTT [2]. Other papers have either not been submitted for publication or have not been accepted into a journal [3]. The only published paper focuses on the older variant wither fewer wildcards. Addi- tionally, this paper focuses on an algorithmic solution to the game, forcing the opponent to play in useless squares. Essentially, it exploited a strategy where the first player could remove all agency from the second player and eventually win. The updated rules specif- 3 Figure 1.2: Complete game of Ultimate Tic-Tac-Toe. O has three boards in a row, winning the game. ically address the exploit used in the paper. Using the updated ruleset, the guaranteed winning solution is no long guaranteed. Other unpublished papers that focus on the new ruleset use artificial intelligence to create an intelligent agent that plays the game. As the game is an adversarial en- vironment, common approaches focus on minimax search as well, using a variety of heuristics [3][1]. Many of these heuristics place a strong emphasis on having as many ”won” boards as possible, meaning that oftentimes these heuristics will make greedy de- cisions that result in a win on a small board, even if winning the board does not further the goal of winning the game (winning a board is only useful if it prevents an opponent win or it can be used to make three in a row). Our goal is to focus on minimax search and create new heuristics that don’t greedily focus on winning as many boards as possible. We do this by calculating a score for a given board state, based on both the smaller board states and the overall state of the game across boards. A board that doesn’t block an opponent’s win and doesn’t help you win is given fewer points than a board that is critical for both player’s winning strategies.
Details
-
File Typepdf
-
Upload Time-
-
Content LanguagesEnglish
-
Upload UserAnonymous/Not logged-in
-
File Pages19 Page
-
File Size-