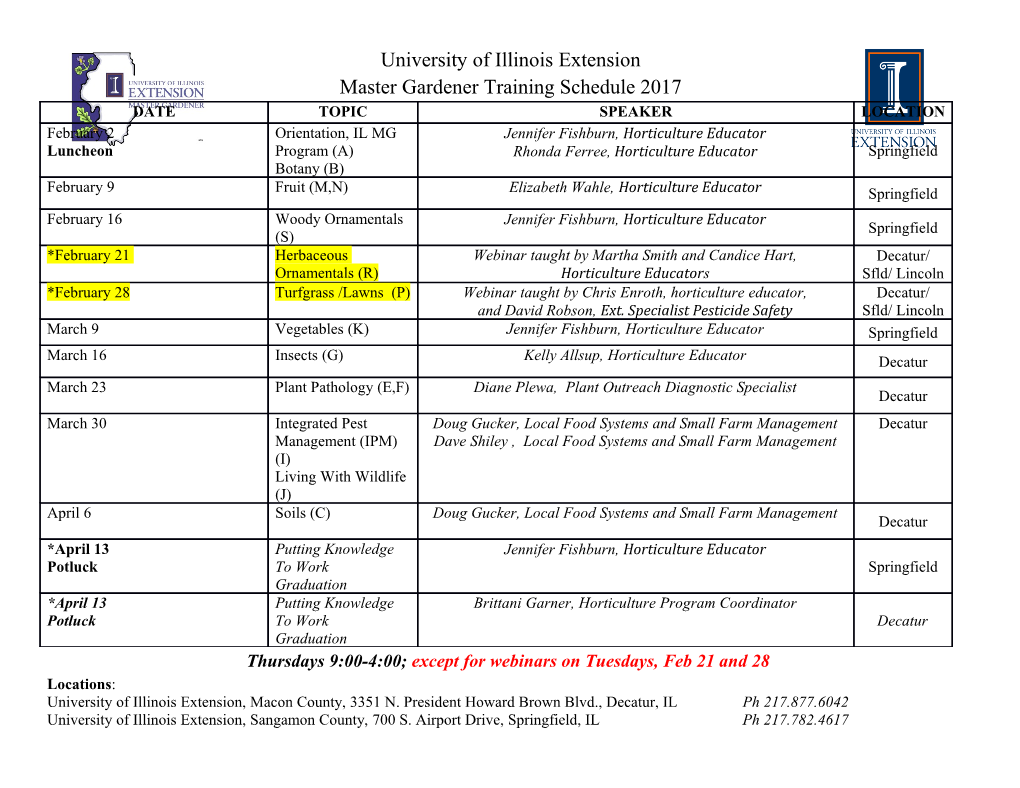
agronomy Review Technologies for Forecasting Tree Fruit Load and Harvest Timing—From Ground, Sky and Time Nicholas Todd Anderson 1,* , Kerry Brian Walsh 1 and Dvoralai Wulfsohn 2 1 Institute for Future Farming Systems, Central Queensland University, Rockhampton 4701, Australia; [email protected] 2 Geco Enterprises Ltd., San Vicente de Tagua, Tagua 2970000, Chile; [email protected] * Correspondence: [email protected] Abstract: The management and marketing of fruit requires data on expected numbers, size, quality and timing. Current practice estimates orchard fruit load based on the qualitative assessment of fruit number per tree and historical orchard yield, or manually counting a subsample of trees. This review considers technological aids assisting these estimates, in terms of: (i) improving sampling strategies by the number of units to be counted and their selection; (ii) machine vision for the direct measurement of fruit number and size on the canopy; (iii) aerial or satellite imagery for the acquisition of information on tree structural parameters and spectral indices, with the indirect assessment of fruit load; (iv) models extrapolating historical yield data with knowledge of tree management and climate parameters, and (v) technologies relevant to the estimation of harvest timing such as heat units and the proximal sensing of fruit maturity attributes. Machine vision is currently dominating research outputs on fruit load estimation, while the improvement of sampling strategies has potential for a widespread impact. Techniques based on tree parameters and modeling offer scalability, but tree crops are complicated (perennialism). The use of machine vision for flowering estimates, fruit Citation: Anderson, N.T.; Walsh, sizing, external quality evaluation is also considered. The potential synergies between technologies K.B.; Wulfsohn, D. Technologies for are highlighted. Forecasting Tree Fruit Load and Harvest Timing—From Ground, Sky Keywords: yield; estimation; machine vision; remote sensing; correlative; models; fruit; tree; review and Time. Agronomy 2021, 11, 1409. https://doi.org/10.3390/ agronomy11071409 1. Introduction Academic Editor: Xiangjun Zou 1.1. The Need for Fruit Load Forecast This review considers advances in methods for the yield forecast of tree fruit. Such Received: 27 May 2021 forecasts can be made at a tree, orchard, whole farm or regional level, depending on Accepted: 8 July 2021 Published: 14 July 2021 the management task. At a farm level, forecasts can inform grower decisions around in-season agronomic practices such as thinning, postharvest requirements such as harvest Publisher’s Note: MDPI stays neutral labor, transportation, storage, and requirements for consumables such as trays and inserts. with regard to jurisdictional claims in Improved monitoring also offers great potential for the accumulation of reliable data across published maps and institutional affil- time. An experienced agronomist will be able to utilize such a resource, combining this iations. information with knowledge of fruit and tree physiology to provide current season advice and to advise on management practices to optimize orchard development. Forecasts are also critical to the value chain to inform marketing strategy and prac- tice. For example, a typical advertising campaign is planned over four weeks ahead of harvest. In general, the longer or more involved the value chain, the more demand for Copyright: © 2021 by the authors. Licensee MDPI, Basel, Switzerland. accurate forecasts. ± This article is an open access article A general target for yield forecast is for an estimate within 5% to 10% of the actual distributed under the terms and yield, with greater accuracy required for industries tied to processing industries or to other conditions of the Creative Commons markets of set size, e.g., export markets. For example, winemakers desire errors of no more Attribution (CC BY) license (https:// than 2–3% on forecast production, while a tree fruit producer supplying multiple and easily creativecommons.org/licenses/by/ accessed domestic markets might accept a 10–20% forecast error, depending on production 4.0/). volumes, or operate without a forecast. Agronomy 2021, 11, 1409. https://doi.org/10.3390/agronomy11071409 https://www.mdpi.com/journal/agronomy Agronomy 2021, 11, x FOR PEER REVIEW 2 of 38 Agronomy 2021, 11, 1409 2 of 37 and easily accessed domestic markets might accept a 10–20% forecast error, depending on production volumes, or operate without a forecast. Yield forecast has multiple components, including the at-harvest fruit number, size and Yieldquality, forecast and harvest has multiple timing. components, Fruit number including is the primary the at-harvest determinant fruit of number, yield, while size andfruit quality, size and and quality harvest are timing. key attributes Fruit number in terms is of the consumer primary determinantacceptability, of and yield, therefore, while fruitachievable size and pricing. quality In-field are key measurements attributes in terms allow of for consumer the forward acceptability, estimation and of the therefore, propor- achievabletions of fruit pricing. class In-fielddistributions measurements on these attributes allow for theat harvest, forward and estimation thus for of market the propor- plan- tionsning. of The fruit timing class distributionsof fruit maturation on these is attributesdetermined at harvest,by the timing and thus of flowering for market and planning. subse- The timing of fruit maturation is determined by the timing of flowering and subsequent quent growth conditions. The combination of harvest timing and fruit load information growth conditions. The combination of harvest timing and fruit load information can be can be used to estimate a weekly harvest schedule. Advances in techniques for the fore- used to estimate a weekly harvest schedule. Advances in techniques for the forecasting of casting of each of these components of yield are presented in the current review. each of these components of yield are presented in the current review. 1.2.1.2. LiteratureLiterature BaseBase InIn thisthis document, document, thethe termterm ‘orchard’‘orchard’ is is used used to to refer refer to to a a management management block block of of fruit fruit trees,trees, i.e., i.e., of of a a common common genotype, genotype, planting planting date, date, irrigation irrigation scheduling, scheduling, management management history, his- harvesttory, harvest unit, etc. unit, A ‘typical’etc. A ‘typical’ orchard orchard size is around size is 1000 around plants 1000 in2 plants ha, but in this 2 ha, is dependent but this is ondependent commodity on andcommodity management and management practice. practice. AA recentrecent review review of of the the use use ofof machinemachine learninglearning forfor cropcrop yieldyield predictionprediction reported reported 567 567 relevantrelevant studies; studies; however, however, this this work work primarily primarily refers refers to to annual annual crops crops [ 1[1].]. For For the the narrower narrower topictopic of of tree tree fruit fruit yield yield forecast, forecast, the the scientific scientific publication publication rate rate has has risen risen steeply steeply from from a a low low basebase inin thethe last last decade decade [ 2[2]] (Figure (Figure1 ).1). FigureFigure 1. 1.Annual Annual scientific scientific paper paper publication publication rate rate as as indexed indexed by by Scopus Scopus records records (doa (doa 18/4/2021) 18/4/2021) forfor thethe keywords keywords ‘tree ‘tree AND AND fruit fruit AND AND yield yield AND AND estimation’. estimation’. PublicationsPublications onon treetree fruitfruit yieldyield estimationestimationhave havefocused focusedon onthe the major major crops crops of of apple apple andand citrus, citrus, and and the the tropical tropical crop, crop, mango mango (68% (68% of records), of records), with with researchers researchers primarily primarily located lo- incated China, in China, the USA the and USA Australia and Australia (Table1 (Table). The majority1). The majority of work of has work addressed has addressed the use ofthe machineuse of machine vision using vision RGB using cameras RGB cameras (65% of records),(65% of records), but attention but attention has also beenhas also given been to thegiven correlation to the correlation of fruit load of fruit to tree load structural to tree structural parameters, parameters, as measured as measured proximally proximally (in field) or(in remotely field) or (by remotely an Unmanned (by an Unmanned Aerial Vehicle, Aerial UAV, Vehicle, or satellite), UAV, and or satellite), to the use and of time to the series use andof time climate series data. and Work climate on data. the use Work of stereology on the use to of streamline stereology manual to streamline sampling manual methods sam- waspling dominated methods bywas the dominated work of Wulfsohn by the work and of co-workers. Wulfsohn and However, co-workers. to our However, knowledge, to theour onlyknowledge, reviews the on treeonly fruit reviews crop on load tree estimation fruit crop published load estimation in the lastpublished decade in have the beenlast dec- on theade use have of been deep on learning the use for of treedeep fruit learning detection for tree using fruit machine detection vision using [3 ]machine rather than vision fruit [3] loadrather estimation than fruit per load se. estimation per se. Agronomy 2021, 11, 1409 3 of 37 Table 1. Literature base as indexed
Details
-
File Typepdf
-
Upload Time-
-
Content LanguagesEnglish
-
Upload UserAnonymous/Not logged-in
-
File Pages37 Page
-
File Size-