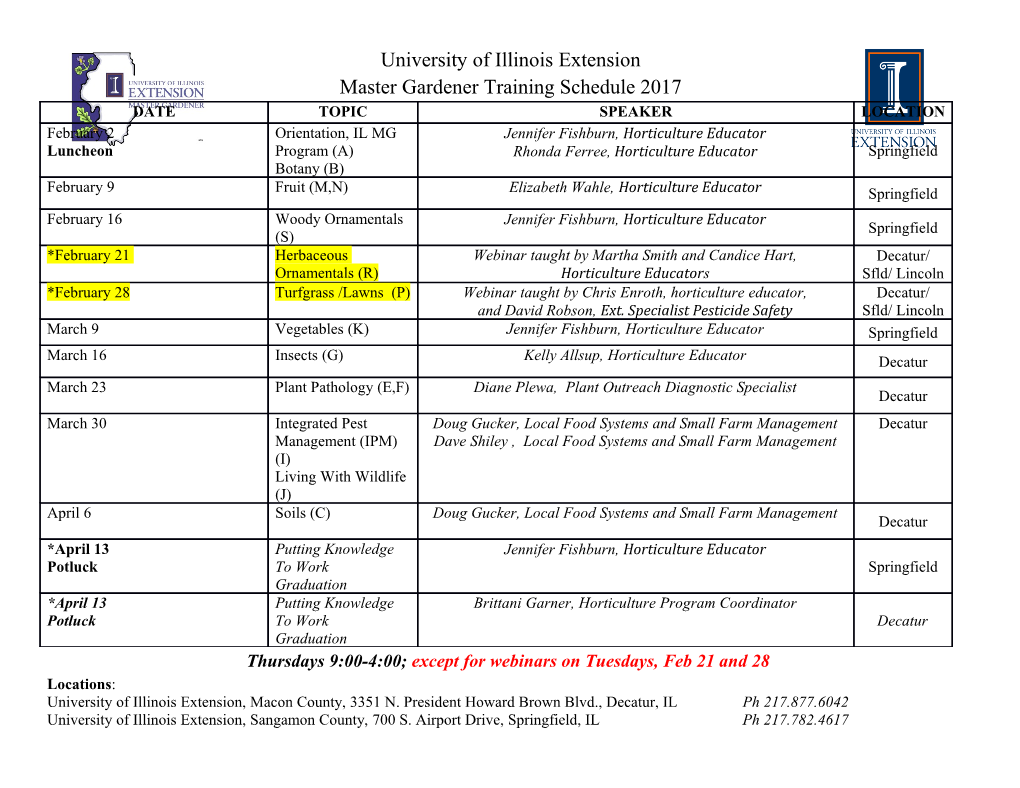
Identifying Optimal Technical and Tactical Performance Characteristics in Australian Football by Christopher Martin Young B Ex Sp Sci (Hons) Submitted in fulfilment of the requirements for the degree of Doctor of Philosophy Deakin University May, 2019 Acknowledgements I have had a truly enjoyable doctoral experience. The challenges associated with completing a PhD, although significant, seemed to diminish with the support I had during this time. Every PhD has its highs and lows and you can only hope that at the end of the day, when all is said and done, that the highs outweigh the lows. I can certainly say that my experience heavily favoured the highs. I would like to thank those people who provided support throughout this journey. To my fellow PhD colleagues who I regularly shared conversations, complaining about the challenges of PhD life, many lunches, coffees, beers and dinners, particularly to Daniel for the many valuable conversations we had about our respective projects, and the friendship we have had over the past decade as we have followed very similar journeys, thank you. To my supervisors thank you for the guidance and support during this time. Jacquie, as an early co-supervisor your involvement in many meetings during the planning stage and revision of manuscripts was greatly appreciated. Wei, your input during key stages was invaluable, and without your support, many methodological aspects of the analysis would have been poorer for it. Paul, your expertise and wealth of knowledge has made numerous valuable contributions to this Thesis, I have greatly appreciated the many conversations we have had and for challenging me at times to reach certain goals. Finally, Dan as my primary supervisor you have gone well beyond the requirements of your position, being available at any time to provide advice and support. I have greatly appreciated the professional and personal friendship we have developed, the many conversations that began as ‘work’, but ended up on something completely unrelated, such as our joint appreciation for good coffee or the ski fields. Without the support you have given me during this time, I undoubtedly would have had a considerably more difficult and less enjoyable candidature, so I would just like to say thank you. To my mum and dad (Diana and Martin), thank you for putting up with me throughout this journey. You have always been incredible sources of support, cooking many meals, making many coffees. I truly appreciate the many things you have done for me during this time. Finally, to my wife Row, thank you for the support you have given me. You have always been an inspiration, always encouraging me to reach my goals. I would never have been able to embark on this journey without your unwavering support. I look forward to the many years ahead. iv Publications and Conference Presentations Studies one, two and three have been published or accepted for publication. Full-text versions are available in the appendices. The details of these are as follows: Published: Study one - Young, C., Luo, W., Gastin, P., Tran, J. and Dwyer, D., 2019. Modelling Match Outcome in Australian Football: Improved accuracy with large databases. International Journal of Computer Science in Sport, 18(1), pp.80-92. (In-press, see Appendix B) Study two - Young, C.M., Luo, W., Gastin, P., Tran, J. and Dwyer, D.B., 2019. The relationship between match performance indicators and outcome in Australian Football. Journal of science and medicine in sport, 22(4), pp.467-471. (see Appendix C). Study three - Young, C.M., Luo, W., Gastin, P., Lai, J. and Dwyer, D.B., 2019. Understanding effective tactics in Australian football using network analysis. International Journal of Performance Analysis in Sport, 19(3), pp.331-341. DOI: 10.1080/24748668.2019.1605562 (see Appendix D) Under review: Study four - Young, C.M., Luo, W., Gastin, P. and Dwyer, D.B., 2019. Understanding the relative contribution of technical and tactical performance to match outcome in Australian Football. April 2019, under review. Conference papers: Christopher Young, Wei Luo, Paul Gastin, Jacqueline Tran, Dan Dwyer (2018). Data mining with Performance Indicators in Australian Rules Football - Methodological Considerations. Exercise and Sport Science Australia: Research to Practice, Brisbane, Australia. 27-29 March. Christopher M Young, Wei Luo, Paul Gastin, Jacqueline Tran and Daniel B Dwyer (2018). Modelling of Match Performance Indicators in Australian Football. World Congress of Performance Analysis of Sport XII, Opatija, Croatia. 19-23 September Christopher M. Young, Wei Luo, Paul Gastin, Jerry Lai & Daniel B. Dwyer (2019) Understanding effective tactics in Australian football using network analysis. Conference Poster. European College of Sport Science (ECSS). Prague, Czech Republic. 3-6 July i Thesis Summary Introduction In recent years, there has been a large growth in the application of ‘data mining’ approaches to analyse sports data. This has occurred, in part due to developments in technology that have increased the collection of data. Consequently, there are improved opportunities to analyse these large datasets to uncover patterns and gain novel insights about performance. This Thesis has analysed, through four experimental Studies, the largest dataset of Australian Football League (AFL) match statistics (by the number of seasons and performance indicators) within the current literature. In doing so, the technical and tactical characteristics of successful and less successful teams have been established. This Thesis was motivated by a need and an opportunity to comprehensively explore and identify the optimal technical and tactical characteristics of Australian Football (AF) through the application of appropriate data analysis methods. Through achieving this, coaches are able to inform their decisions by an evidence-base, reducing the burden placed on key decision-makers. Methods Ninety-one team match aggregate performance indicators (PIs), from 3,145 AFL matches (16 seasons- 2001 to 2016) were analysed in Studies one and two. The dataset analysed in Study three was in the form of an event log with every match event timestamped. This included 1,516 AFL matches (8 seasons) and 932,808 player interactions. A combination of the datasets used in Studies one and two, and the derived Social Network Analysis (SNA) measures from Study three were analysed in Study four. Segmented regression analysis and a Change-point analysis were used in Study one to identify eras across the 16 AFL seasons; and applied an ensemble feature selection method to rank PIs by their importance to match outcomes (Win- Loss and Score margin). The top 45 ranked PIs identified for each era in Study one were used to develop decision tree and Generalised Linear Models (GLMs) in Study two. Social Network Analysis was used to identify the relationship between measures of teamwork (i.e. the interactions between players) and match outcome in Study three. Study four incorporated the methods implemented in Studies one, two (feature selection and identified eras) and three (SNA measures), creating combined models, including technical and tactical datasets. ii Results Eras exist in the 16 years (2001–2016) of AF data, but establishing their location is difficult. In general, the relative form of PIs are more important than their absolute form. The use of a wider range of technical PIs in modelling revealed new PIs that are more important than other more established PIs. The use of a longer duration database allows for the creation of models with the highest published classification accuracies for match outcome. It is possible to characterise the tactical performance of AF teams by analysing their passing network in a match. Tactical PIs identified as important in relation to match outcome in AF include: Transitivity, Average path length and Edge count. Tactical PIs derived from SNA have not been used in this way previously. The combination of technical and tactical PIs did not substantially improve model performance and there are several reasons for this (e.g. large PI database already has very high model accuracy, Bayes error-rate/ceiling effect). Conclusions When analysing a longitudinal dataset, several methods of detecting eras should be used to improve the likelihood of identifying the true underlying reality. An ensemble method of feature selection provides stable and reliable results and should be utilised in future research. The most valuable PIs included several that have not been previously identified as important. Further, feature selection identified that the relative form of PIs were more valuable than the absolute form. The decision tree models provide key PIs (KPIs) and the values of these KPIs can be benchmarked and targeted by coaches. The accuracy of these models is as high and higher than in previous reports, which underscores the value of conducting an analysis using a larger database with a wider range of PIs. Australian Football teams have relatively stable but different teamwork characteristics and there are differences in measures of teamwork between teams that win and lose AF matches. These findings demonstrate that SNA can be used to assess teamwork in AF. The combination of technical and tactical PIs in models that explain match outcome did not perform significantly better than previous technical PI models. The optimal technical and tactical characteristics of AF teams have been identified from the findings in this Thesis. iii Table of Contents List of Figures ______________________________________________________
Details
-
File Typepdf
-
Upload Time-
-
Content LanguagesEnglish
-
Upload UserAnonymous/Not logged-in
-
File Pages172 Page
-
File Size-