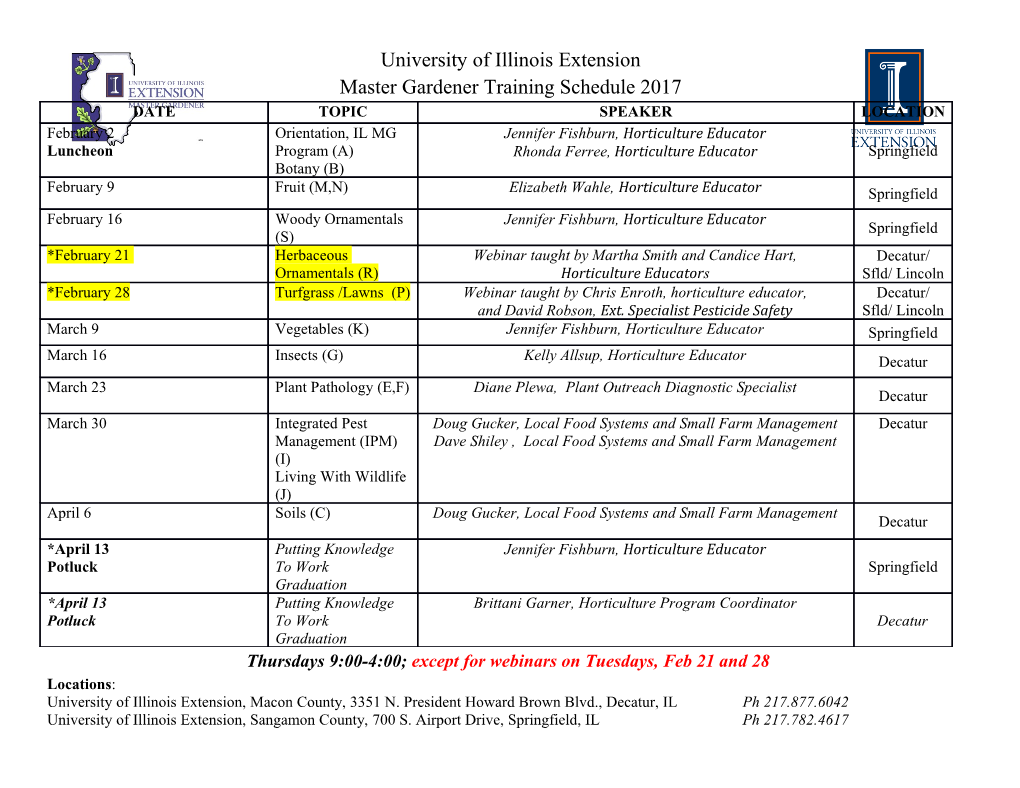
Available online at www.sciencedirect.com ScienceDirect Working memory in children’s math learning and its disruption in dyscalculia Vinod Menon Working memory (WM) plays an essential role in children’s WM components at different stages of mathematical skill mathematical learning. WM influences both the early acquisition. Deficits in WM in children with dyscalculia foundational phases of number knowledge acquisition and contribute to weaknesses in the representation of quanti- subsequent maturation of problem solving skills. The role of ty information, as well as the ability to manipulate this individual WM components in mathematical cognition depends information during numerical problem solving [7 ]. Con- not only on problem complexity but also on individual vergent findings from neuroimaging studies provide fun- differences in mathematical abilities. Furthermore, the damental insights into the link between WM and contributions of individual WM components change mathematical cognition, and the mechanisms by which dynamically over development with visuospatial processes poor WM contributes to dyscalculia. A common neural playing an increasingly important role in learning and enhancing locus of deficits in visuospatial quantity representations mathematical proficiency. Convergent findings from and visuospatial WM likely contributes to both numerical neuroimaging studies are now providing fundamental insights magnitude judgment and arithmetic problem solving into the link between WM and mathematical cognition, and the deficits in children with dyscalculia. mechanisms by which poor WM contributes to learning disabilities. Evidence to date suggests that visuospatial WM is Working memory in children’s mathematical a specific source of vulnerability in children with mathematical cognition and learning learning disabilities and needs to be considered as a key The particular emphasis on WM in developmental stud- component in cognitive, neurobiological, and developmental ies has its origins in children’s immature problem solving models of typical and atypical mathematical skill acquisition. abilities, which require them to break down numerical Address problems into more basic components. The use of such Stanford University, Stanford, CA, United States strategies necessitates greater reliance on WM systems for problem solving in children. For example, children Corresponding author: Menon, Vinod ([email protected]) rely more on counting strategies during simple arithmetic problem solving and need to access multiple WM com- Current Opinion in Behavioral Sciences 2016, 10:125–132 ponents including short-term storage and rule-based manipulation and updating of the contents of stored This review comes from a themed issue on Neuroscience of education information [8]. With increased proficiency and a switch to fact retrieval strategies there is less demand and need Edited by De´ nes Szu¨ cs, Fumiko Hoeft and John DE Gabrieli for WM resources [9,10]. The link between WM and For a complete overview see the Issue and the Editorial children’s mathematical cognition and learning has large- Available online 7th June 2016 ly been based on Baddeley and Hitch’s influential mul- doi:10.1016/j.cobeha.2016.05.014 ticomponent model [11,12]. Briefly, this model includes a 2352-1546/# 2016 Elsevier Ltd. All rights reserved. central executive component, responsible for high-level control, monitoring, and task switching, along with two subordinate, modality-dependent components, impor- tant for short-term storage of verbal and visuospatial information, respectively [11]. Crucially, all three com- ponents of WM can be distinguished from an early age Introduction [13]. Many aspects of children’s academic skill acquisition require access to working memory (WM) resources Developmental studies using the Baddeley and Hitch [1–3]. In no academic domain is this truer than in math- model have predominantly reported a strong link ematical cognition where problem solving abilities de- between the central executive and visuospatial WM pend on the capacity to efficiently manipulate quantity components and math abilities [9,14–17] (Simmons representations in WM [4 ,5]. Over three decades of et al., 2012). The effects of phonological WM have gen- behavioral research have established that numerical prob- erally been much weaker, and are typically more evident lem solving tasks place strong demands on the active during very early stages (ages 4–5), when phonological maintenance and manipulation of task-relevant informa- representations for numbers are still weak and word- tion in WM [5,6]. Cross-sectional and longitudinal studies based problem solving places greater demands on reading are providing new insights into the role of individual comprehension. In a detailed cognitive analysis of the www.sciencedirect.com Current Opinion in Behavioral Sciences 2016, 10:125–132 126 Neuroscience of education factors that contribute to mathematical abilities, Szucs Longitudinal studies have expanded on these findings and colleagues found strong relations between visuospa- and shown that the central executive component predicts tial WM measures, but not phonological WM measures, performance on single-digit addition tasks in grades 1 to and mathematical abilities in a large well-characterized 3 as well as faster transitions from simple (e.g., counting) group of 9 year-old children [18]. to sophisticated (e.g., decomposition) solution strategies Figure 1 (a) Numerical L R (b) Arithmetic (c) Working Memory (d) Visuospatial Current Opinion in Behavioral Sciences Common patterns of fronto-parietal network activations elicited by numerical, arithmetic, working memory and visuospatial processing tasks. Results from meta-analysis conducted using Neurosynth (www.neurosynth.org) with the corresponding search terms. Current Opinion in Behavioral Sciences 2016, 10:125–132 www.sciencedirect.com Working memory in children’s math learning Menon 127 [16]. Similarly, in a large sample of 673 children, Lee and abilities in third graders [17]. Similarly, Li and Geary Bull found that WM updating capacity in kindergarten reported individual differences in the growth rate of visuo- predicted growth rate of math abilities (numerical opera- spatial WM during childhood. Notably, they found that tions) in subsequent grades [19]. these differences became increasingly important for learn- ing over time [20]. Nuerk and colleagues examined longi- It is important to note that the role of individual WM tudinal changes associated with multiplication fact retrieval components depends not only on task complexity but also [21 ]. They found that multiplication task performance was on children’s developmental stage. The changing role of correlated with verbal WM in third graders but with visuo- WM components can be detected even in a 1-year time- spatial WM in grade four. Taken together, these patterns of window between ages 8 and 9. Meyer and colleagues found relationships suggest that the contributions of individual that while the central executive and phonological compo- WM processes change dynamically over development with nents of WM predicted mathematical abilities in second visuospatial WM processes playing an increasingly impor- graders, it was the visuospatial component that predicted tant role in enhancing mathematical proficiency. Figure 2 SMG IPS SMG CE PL VS Overlap of VS and CE Overlap of PL and CE L SMG L IPS Z = 34 Y = –43 Z = 54 Y = –47 CE r = .334 CE r = .417 1 VS r = .353 1 PL r = .374 ) ) β β 0 0 Activity ( –1 Activity ( –1 –2 –2 51015 2025 30 5 15 25 35 Working Memory Score Working Memory Score Current Opinion in Behavioral Sciences Functional dissociations and overlap between brain areas associated with each of the three components of working memory in 7 to 9-year-old children (N = 74). The neural correlates of the central executive (CE), phonological (PL) and visuo-spatial (VS) components of working memory were examined by contrasting brain responses to two different types of addition problems that differed in complexity. Overlap between the CE and VS components was observed only in left supramarginal gyrus (SMG); overlap between CE and PL components was observed only in the left intra-parietal sulcus (IPS); no overlap was observed between VS and PL components. Negative correlation between activity and PL ability is not depicted. No overlap for VS and PL (magenta) was observed. Bottom panel: coronal slices depict regions of interest selected as overlap in correlations of activity and individual working memory components. Scatter plots are based on functional clusters identified using whole-brain regression analysis, and are provided for the purpose of visualization. L, left. Source: [23 ]. www.sciencedirect.com Current Opinion in Behavioral Sciences 2016, 10:125–132 128 Neuroscience of education Figure 3 (a) (a) TD R AIC L IPS R IPS L MFG L MFG R MFG R MFG R Visual L IFG Cortex Y = 32 L IPS Z = 10 Precuneus R MFG R Fusiform Gyrus Cingulate Gyrus Cerebellum Cerebellum Y = –44 X = 0 Y = –56 X = 40 (b) DD L Postcentral Gyrus Positive Correlations 2 4 Negative Correlations 2 4 TD > DD (b) (a) Prefrontal L IFG R MFG 4 4 ∗∗ 3 r = –.65 3 ∗ 2 r = –.23 2 r = –.29 r = –.60 1 1 T Score 0 T Score 0 –1 –1 –2 –2 50 60 70 80 90 100 110 120 50 60 70 80 90 100 110 120 X = –42 Block Recall Z = 36 Block Recall (b) Parietal L IPS R SMG 4 4 3 ∗ 3 ∗ 2 r = .55 r = –.50 r = –.21 2 r = –.15 1 1 T Score 0 T Score 0 –1 –1 –2 –2 50 60 70 80 90 100 110 120 50 60 70 80 90 100 110 120 Block Recall Z = 20 Z = 36 Block Recall DD 2 4 TD
Details
-
File Typepdf
-
Upload Time-
-
Content LanguagesEnglish
-
Upload UserAnonymous/Not logged-in
-
File Pages8 Page
-
File Size-