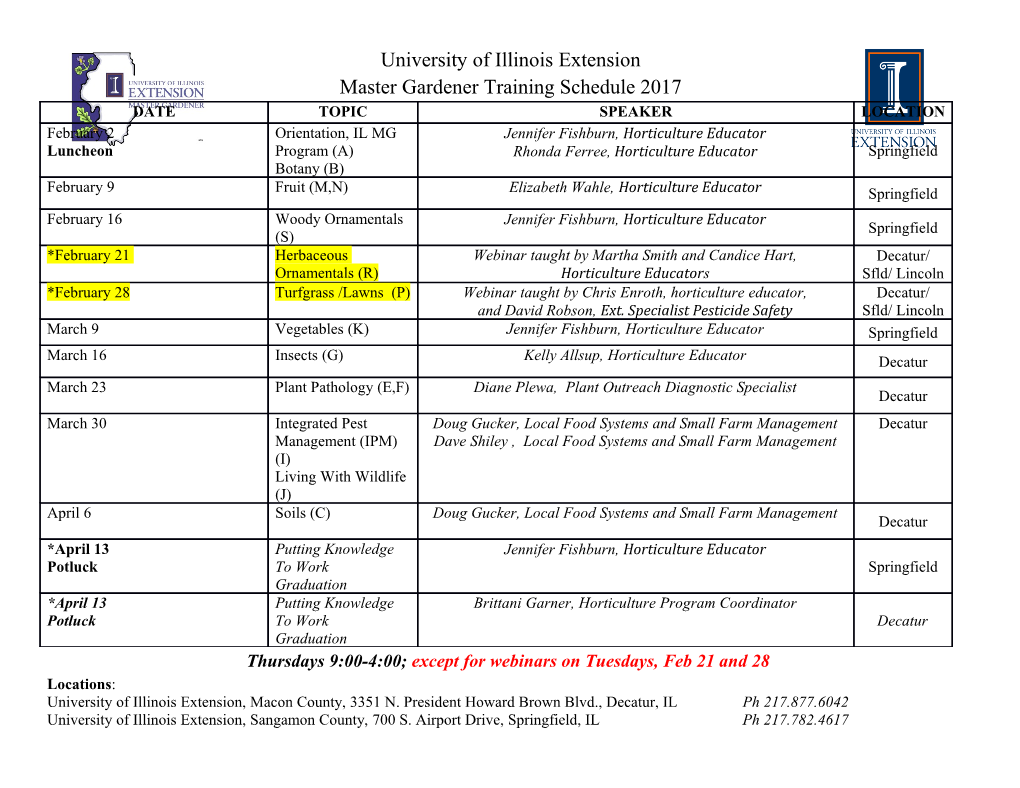
Modeling and Visualization of forest cover transition in protected areas Forests are complex ecosystems, requires efficient forestry management. This involves consideration of current and future management issues including the richness of living organisms in forests, the uniqueness of forest dependent people. Monitoring and mediating the negative consequences LULC (land use land cover) change will help in sustainable planning, decreasing environmental consequences such as climate change, biodiversity loss, land degradation, soil erosion and pollution of water, air. Modeling and visualization techniques have been widely applied to account and forecast changes in forests across the world. Modeling and visualization allows to quantify spatial and temporal variability in landscape at a finer scale, where similar studies on the ground would be logistically impossible (Amato et al., 2011). LULC modeling for forested landscape is often used for predicting trajectories of future, analyzing the fundamental socio-political, economic, cultural and biophysical forces acting. Modeling approach focuses on two disparate parts acting at a landscape i.e. processes, and spatial patterns. The two parts have included in dynamic models by presuppose homogeneity and immediate information transfer at various spatial extensions (Ramachandra et al., 2017). In general models are developed based on defensible empirical data, which bring findings back from abstraction to some real world understanding. The two basic modeling approaches are theoretical and simulation modeling. Theoretical models are built on mathematical principles and applying them to natural systems (Clark, 1991), which adds complexity in understanding pattern and process. Simulation models incorporate simple complexity, but reflect actual ecological accurately with better readability. Due to continuous improvement in technology, many models are developed for accounting patterns and processes effectively which connect biophysical and human related factors, explicit inclusion of human nature interaction mechanisms. The development of various models has considered biophysical and human interactions on land use at varied scales rather than land systems as a whole (Rounsevell et al., 2012). Numerous models have been advanced such as Markov cellular automata models (MCA), AHP (Analytical Hierarchical Processes), logistic regression models, ANN system (Artificial Neural Network), SWAM optimization (Ballestores et al., 2012; Bharath et al., 2016; Hyandye and Martz, 2017; Satty, 2008; Bharath et al., 2017; Citation: Ramachandra T.V, Setturu Bharath, Nimish Guptha, Bhargavi R Sharma 2017, Modeling and Visualization of Forest Cover Transition in Protected Areas, Sahyadri E-news, 2017 (58) : 3-45, http://wgbis.ces.iisc.ernet.in/biodiversity/sahyadri_enews/newsletter/Issue58/index.htm. Tayyebi et al., 2014; Yuan et al., 2009; Liu et al., 2013) for better understanding land use pattern and processes. Markov chain approach is a stochastic widely implemented to study the dynamics of land use change at different scales under the assumptions of probability of a system being in a certain state at certain time can be determined if its state at an earlier time is known (Halmy et al., 2015). Markov chain analysis does not assume statistical independence of data as required by logistic regression, which makes it suitable for the spatially dependent land use data to predict all multi directional land use changes (Overmars et al., 2003; Pontius and Malanson, 2005). The stationary transitions are assumed by the Markov models is one of the issues and not spatially explicit which makes it more suitable for short-term projections (Sinha and Kimar, 2013). This shortcoming of the technique can be overcome through the integration with other different dynamic and empirical models (Bharath et al., 2014). Integrated modeling approaches are considered more suitable for modeling land use change processes (Guan et al., 2011). Markov models can be integrated with cellular automata models (MCA) to model and predict land use change at different scales. Forest land use changes are most non-linear process, MCA considered as effective compare to other techniques. MCA model is simple and provides better understanding of how nonlinear process can aptly simulated by less complexity. MCA has the ability to predict any transition among any number of categories, as compared to many techniques. Many models transitions can be only a one way as from one category to one alternative category (Pontius and Malanson, 2005). Due to MCA’s advantages of multiple LU classes transition, that integrate the aspatial Markov with the spatial CA model outperformed regression based models in prediction, accuracy at multiple resolutions stands out as an effective modeling tool. The modeling of spatiotemporal changes across the protected areas are evaluated using MCA analysis. A cellular automaton has capable of modeling surprisingly complex behavior, defined as a large lattice of identical finite state automata (cells) (Von Neumann, 1966). Each state or move is defined as a triplet: < 퐼, 푆, 푊 > where I is the set of inputs, S is a set of states (both sets being finite), and W is the next-state function, defined on input-state pairs. The set of inputs is defined as ordered (or non-ordered) n- tuples of the states of a finite set of “neighboring” cells. In a two dimensional CA typically Citation: Ramachandra T.V, Setturu Bharath, Nimish Guptha, Bhargavi R Sharma 2017, Modeling and Visualization of Forest Cover Transition in Protected Areas, Sahyadri E-news, 2017 (58) : 3-45, http://wgbis.ces.iisc.ernet.in/biodiversity/sahyadri_enews/newsletter/Issue58/index.htm. neighborhood is considered as four or eight the adjacent cells in a square lattice based on typical rules as the Moore and von Neumann neighborhoods respectively. The Markov model is a theory based on the process of the formation of Markov random process systems for the prediction and optimal control theory method. Based on the Bayes conditional probability formula, the prediction of land use changes is calculated by the following equation: 푃(푁) = 푃(푁 − 1) ∗ 푃푖푗 (1) where, P(N) is state probability of any times, and P(N−1) is preliminary state probability. Transition area matrix can be obtained by, 푃11 푃12 푃13 푇푟푎푛푠푖푡푖표푛 푎푟푒푎 푀푎푡푟푖푥 푃 = [ ⋮ ⋮ ⋮ ] (2) 푃푁1 푃푁2 푃푁푁 th th where, Pij is the sum of areas from the i land use category to the j category during the years from start point to target simulation periods; and n is the number of land use types. The transition area matrix must meet the following conditions i. 0 ≤ Pij ≤1 푛 ii. ∑푖,푗=0 푃푖푗 = 1 Predictions of future LULC change of each protected areas (PA) using a MCA model emerged in three steps: 1) applying the Markov chain analysis to the 1973, 1991, and 2016 LULC maps for calculating transition matrices; 2) calculating transition potential maps of LULC; and 3) application of the CA model to the transition matrices and the transition potential maps to predict the spatial distribution of LULC. Markov process results in a transition probability matrix of land use change between two different dates, which is derived from observations. The transition probability matrix provides an estimate of the probability that each pixel of certain LULC class will be transformed to another class or remains in its class. The accuracy of each prediction is evaluated using current land use maps based on Kappa statistic. The Kappa-standard index of optimum point of the diagram as well as Kappa-location index were computed proves a significant Citation: Ramachandra T.V, Setturu Bharath, Nimish Guptha, Bhargavi R Sharma 2017, Modeling and Visualization of Forest Cover Transition in Protected Areas, Sahyadri E-news, 2017 (58) : 3-45, http://wgbis.ces.iisc.ernet.in/biodiversity/sahyadri_enews/newsletter/Issue58/index.htm. correlation between the simulated and the actual maps Table 1 shows the transition matrix of ADTR region based on land use maps of 1973-1992; 1992-2016. The transition probability and transition areas were computed for all protected areas to account category wise changes. Table 1: Transition probability matrix of ADTR based on 1973-1992. Sno Category 1 2 3 4 5 6 7 8 9 Ever green to 1 0.823 0.076 0.020 0.004 0.043 0.008 0.000 0.000 0.026 semi evergreen Deciduous 2 0.221 0.561 0.023 0.113 0.004 0.006 0.008 0.035 0.026 forest 3 Scrub_Grass 0.045 0.101 0.436 0.077 0.006 0.064 0.022 0.048 0.196 Forest 4 0.168 0.118 0.033 0.511 0.003 0.019 0.016 0.033 0.100 Plantations 5 Horticulture 0.058 0.139 0.055 0.045 0.562 0.048 0.018 0.045 0.025 6 Agriculture 0.024 0.040 0.050 0.090 0.196 0.37 0.018 0.097 0.116 7 Water 0.027 0.064 0.031 0.078 0.013 0.020 0.53 0.017 0.221 8 Built-up area 0.011 0.031 0.045 0.041 0.004 0.087 0.026 0.563 0.188 9 Open area 0.000 0.004 0.132 0.019 0.244 0.061 0.003 0.004 0.534 Table 2: Transition probability matrix of ADTR based on 1992-2016. Sno Category 1 2 3 4 5 6 7 8 9 Ever green to 1 0.867 0.008 0.013 0.000 0.096 0.008 0.000 0.000 0.009 semi evergreen Deciduous 2 0.007 0.714 0.087 0.000 0.073 0.014 0.000 0.004 0.102 forest 3 Scrub_Grass 0.000 0.163 0.540 0.096 0.110 0.003 0.000 0.001 0.088 Forest 4 0.089 0.019 0.099 0.400 0.180 0.033 0.001 0.013 0.166 Plantations Citation: Ramachandra T.V, Setturu Bharath, Nimish Guptha, Bhargavi R Sharma 2017, Modeling and Visualization of Forest Cover Transition in Protected Areas, Sahyadri E-news, 2017 (58) : 3-45, http://wgbis.ces.iisc.ernet.in/biodiversity/sahyadri_enews/newsletter/Issue58/index.htm.
Details
-
File Typepdf
-
Upload Time-
-
Content LanguagesEnglish
-
Upload UserAnonymous/Not logged-in
-
File Pages45 Page
-
File Size-