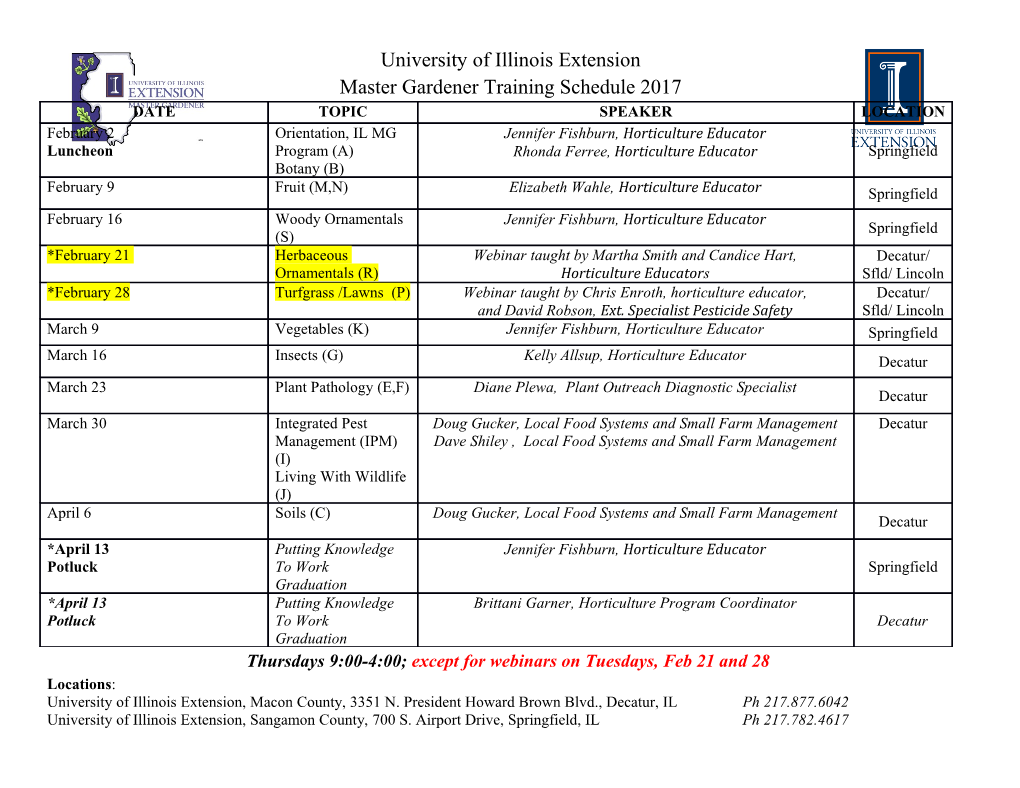
Surveys, simulation and single-cell assays relate function and phylogeny in a lake ecosystem The MIT Faculty has made this article openly available. Please share how this access benefits you. Your story matters. Citation Preheim, Sarah P. et al. “Surveys, Simulation and Single-Cell Assays Relate Function and Phylogeny in a Lake Ecosystem.” Nature Microbiology 1.9 (2016): 16130. As Published http://dx.doi.org/10.1038/nmicrobiol.2016.130 Publisher Nature Publishing Group Version Author's final manuscript Citable link http://hdl.handle.net/1721.1/108409 Terms of Use Article is made available in accordance with the publisher's policy and may be subject to US copyright law. Please refer to the publisher's site for terms of use. 1 Title: 2 Surveys, simulation, and single-cell assays relate function and phylogeny in a lake ecosystem 3 4 Authors: 5 Sarah P. Preheima,b†, Scott W. Olesena†, Sarah J. Spencera, Arne Maternac, Charuleka 6 Varadharajand, Matthew Blackburne, Jonathan Friedmanf, Jorge Rodríguezg, Harold Hemondh 7 and Eric J. Alma,* 8 9 a Department of Biological Engineering, Massachusetts Institute of Technology, Cambridge, MA 10 02139 11 b Department of Geography and Environmental Engineering, Johns Hopkins University, 12 Baltimore, MD 21218 13 c Qiagen Corp., Aarhus, Denmark 14 d Lawrence Berkeley National Laboratory, Berkeley, CA 94720 15 e École Polytechnique Fédérale de Lausanne, Lausanne, Switzerland 16 f Department of Physics, Massachusetts Institute of Technology, Cambridge, MA 02139 17 g Institute Centre for Water and Environment (iWater), Masdar Institute of Science and 18 Technology, Abu Dhabi, UAE 19 h Department of Civil and Environmental Engineering, Massachusetts Institute of Technology, 20 Cambridge, MA 02139 21 22 † These authors contributed equally to this work. 23 24 * To whom correspondence should be addressed 25 26 27 Summary paragraph: 28 Much remains unknown about what drives microbial community structure and diversity. 29 Highly structured environments might offer clues. For example, it may be possible to 30 identify metabolically similar species as groups of organisms that correlate spatially with 31 the geochemical processes they carry out. Here, we use a 16S ribosomal RNA gene survey 32 in a lake that has chemical gradients across its depth to identify groups of spatially correlated but 33 phylogenetically diverse organisms. Some groups had distributions across depth that aligned 34 with the distributions of metabolic processes predicted by a biogeochemical model, suggesting 35 these groups performed biogeochemical functions. A single-cell genetic assay showed, however, 36 that the groups associated with one biogeochemical process, sulfate reduction, contained only a 37 few organisms that have the genes required to reduce sulfate. These results raise the possibility 38 that some of these spatially-correlated groups are consortia of phylogenetically diverse and 39 metabolically different microbes that cooperate to carry out geochemical functions. 40 41 Keywords: ecology / 16S / function / gradient / phylogeny / lake / co-occurrence 42 Introduction 43 Explaining the vast diversity of microbes found in many ecosystems 1,2 is a challenge for 44 microbial ecology. Environments with chemical or other abiotic gradients like temperature have 45 been a key resource for studying microbial ecology. For example, studies in Winogradsky 46 columns 3, microbial mats 4, mine drainage sites 5, hydrothermal vents 6, and dimictic lakes 7 47 have provided insight about the relationships between environmental parameters, microbial 48 diversity, and ecosystem functions. Microbial surveys with spatial scales comparable to those of 49 the ecosystem gradients can identify groups of spatially-correlated organisms and relate the 50 distribution of those organisms to the environmental gradients. 51 52 There are challenges to interpreting the relationship between organisms in spatially-correlated 53 groups and environmental information. First, the relationship between an organism’s spatial 54 distribution and environmental parameters can be complicated. For example, a naïve expectation 55 might be that sulfate-reducing organisms are abundant where sulfate concentrations are highest. 56 In fact, the distribution of sulfate-reducing organisms also depends on the distribution of more 57 favorable electron acceptors and the transport of sulfur compounds around the ecosystem. Even 58 more subtly, bacterial populations may be capable of performing multiple metabolisms, and they 59 can even be simply inactive. Thus, there is a need to develop techniques that provide quantitative 60 expectations about factors that shape organismal distributions given observed environmental 61 information. 62 63 A second challenge is that there are multiple experimental methods that can verify the 64 relationships between function and phylogeny, but most of these methods are in vitro or perturb 65 the environment 8. A method that relates phylogeny and function without perturbing the natural 66 ecosystem would clarify the in situ functional relationships between organisms in a spatially- 67 correlated group. Deep metagenomic sequencing along with differential genome binning 68 techniques can produce draft genomes from complex communities 9 but is expensive and cannot 69 target specific functions. 70 71 A third challenge to studying spatially-correlated organisms in ecosystems with gradients is 72 relating the groups’ diversities to their environmental functions, especially if these organisms are 73 unrelated. Organisms in these groups could use similar resources, as it is know that many traits 74 are widespread in the tree of life 10, or could have recently exchange genes through horizontal 75 gene transfer 11. Unrelated organisms with similar distributions could also be found together 76 because they are part of multispecies, symbiotic associations 12. The challenge lies in 77 differentiating between these or other possibilities. 78 79 In this paper, we investigate spatially-correlated organisms in an ecosystem with gradients. First, 80 we conducted a microbial survey of a dimictic lake. Second, we constructed a quantitative, 81 dynamic biogeochemical model that shows how bacteria can drive the creation of chemical 82 gradients. Third, we show that there are many groups of spatially-correlated organisms in this 83 lake and relate those groups to the biogeochemical model. Finally, we used a single-cell assay to 84 investigate the functional capabilities of the groups of spatially-correlated bacteria related to one 85 modeled process, sulfate reduction. We show that, taken together, these results raise the 86 possibility that these spatially-correlated groups are multispecies, symbiotic associations of 87 microbes, that is, consortia 13. 88 89 Results 90 Community structure is influenced by geochemistry. We performed our study in Upper 91 Mystic Lake, a dimictic, eutrophic freshwater lake outside Boston, MA because this lake is 92 seasonally stratified and supports complex microbial communities that catalyze well- 93 characterized biogeochemical cycles 14-19. The seasonal stratification means that the deepest 94 three-quarters of this lake is anoxic, supporting fewer predators that can complicate microbial 95 distribution patterns, and that the deeper parts of the lake are relatively isolated from external 96 inputs. 97 98 To characterize microbial diversity, we conducted amplicon-based bacterial surveys (16S rRNA 99 gene library from DNA samples) along a vertical transect in the lake, collecting samples at 100 approximately each meter of depth. We grouped 16S rRNA gene sequences into operational 101 taxonomic units (OTUs) using the ecologically-informed distribution-based clustering algorithm, 102 which merges sequences from related organisms that have similar spatial distributions 20. We 103 also measured major geochemical parameters (temperature, specific conductivity, dissolved 104 oxygen, nitrate, iron and sulfate; Fig. S1-S3). 105 106 The 16S rRNA gene survey showed that biogeochemistry had a major influence on the bacterial 107 community structure (Fig. 1). Transitions in community structure lined up with the lake’s major 108 geochemical features: the thermocline, oxycline, and nitrocline (Fig. 1b). Cyanobacteria were 109 most abundant near the surface (Fig. 1a). Bacteroidetes, Actinobacteria, and Proteobacteria were 110 abundant across depths. δ-Proteobacteria, which include most of the known sulfate-reducing 111 bacteria, were abundant only below the nitrocline where more favorable terminal electron 112 acceptors were exhausted. The differences in geochemistry and bacterial community structure 113 across depths suggest that organisms exploiting similar resources should have correlated 114 distributions across depths. 115 116 Multiple groups of spatially-correlated OTUs in the lake. To identify groups of spatially- 117 correlated organisms, we used hierarchical clustering to group the 536 most abundant OTUs into 118 49 groups based on the similarity of the OTUs’ distributions across depths. We call these groups 119 operational ecological units (OEUs) because they are groups of organisms that we expect have 120 functional or ecological relationships (thus “ecological”) but were defined in a purely statistical 121 way (thus “operational” 21). 122 123 Most OEUs contained OTUs from multiple phyla. OEUs ranged in size from 2 to 33 OTUs and 124 contained 1 to 10 phyla. The number of phyla in each OEU (0.34 additional phyla per OTU 125 beyond the
Details
-
File Typepdf
-
Upload Time-
-
Content LanguagesEnglish
-
Upload UserAnonymous/Not logged-in
-
File Pages46 Page
-
File Size-