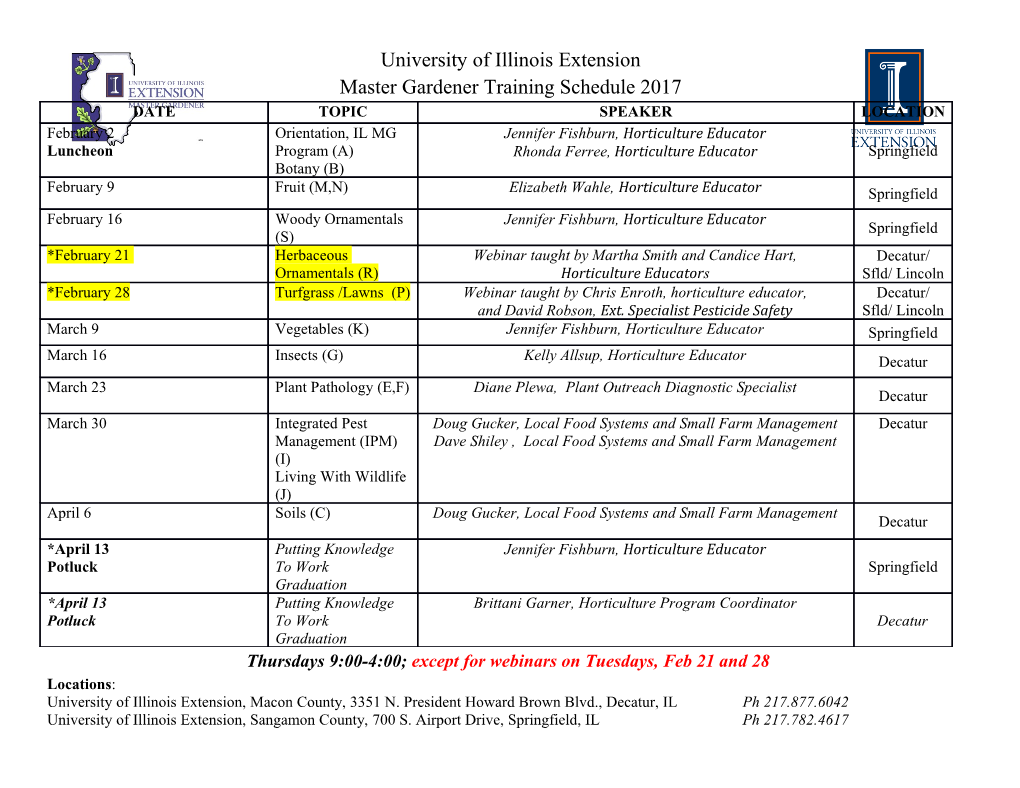
(IJACSA) International Journal of Advanced Computer Science and Applications, Vol. 12, No. 3, 2021 Smart Internet of Vehicles Architecture based on Deep Learning for Occlusion Detection Shaya A. Alshaya Department of Computer Science College of Science and Humanities at alGhat, Majmaah University, Majmaah, 11952, Saudi Arabia Abstract—In our days, the cyber world is developing due to avoid forged data based on interpretation mechanisms. Besides, the revolution of smart cities and machine learning technologies. deep learning methods reduce redundancy and provide high The internet of Things constitutes the essential background of robustness of the system by following the inspection's high cyber technology. As a case study, the Internet of Vehicles is one quality. of the leading applications which is developed quickly. Studies are focused on resolving issues related to real-time problems and Therefore, moving from a centralized approach to edge privacy leakage. Uploading data from the cloud during the data computing reduces the unnecessary jumps to the network and collection step is the origin of delay issues. This process decreases improves latency [5]. Privacy is enhanced by adopting a the level of privacy. The objective of the present paper is to Collaborative Learning (CL) technology. It manages data ensure a high level of privacy and accelerated data collection. through multiple decentralized edge devices without During this study, we propose an advanced Internet of Vehicle exchanging them. It follows a training method different from architecture to conduct the data collection step. An occlusion the traditional cloud data center. The trained data were not detection application based on a deep learning technique is collected directly from terminals [6]. The CL technology performed to evaluate the IoV architecture. Training data at ensures privacy by gathering models and their updates from Distributed Intelligent layer ensures not only the privacy of data each RSU. but also reduces the delay. All RSU shares the model of the cloud data center. The CL Keywords—Internet of vehicle; deep learning; collaborative performs the following steps: (1) the user provides data, (2) the technologies; cloud; edge computing local model trains the provided data, and (3) the trained model is uploaded to the cloud data center. I. INTRODUCTION However, the CL mechanism provides more privacy and The recent development of network technology gives birth reduces delay by decreasing the training time of the sharing to cyber intelligence technologies. The Internet of Vehicles model in the cloud. (IoV) is a subfield of the Internet of Things (IoT) that has evolved a new generation of network technology [1]. In light of this introduction, we can summarize contributions into three points: The IoV case analyzes data based on artificial intelligence (AI) and Machine Learning (ML) methods. This field is still 1) Propose an advanced architecture at the edge computing challenged by analyzing big data, privacy, and computational layer for the IoV. The proposed ensures intelligent data power limitation. collection. The IoV cooperates with the cloud platform to ensure 2) Propose a deep learning method for the preprocessing permanent services. Two issues are faced with the IoV step. The proposed reduces the delay. applications: (1) delay problem and (2) privacy violation [2]. 3) Deploy the CL technology between the local model We define an intermediate layer called edge computing to from the edge node and the cloud edge. manage the vehicle network layer and cloud layer to overcome The next section presents the literature review. The these problems. The edge computing layer is determined by the proposed IoV architecture is introduced in Section III. The data location information and the low latency. This is obtained preprocessing schemes designed according to a deep learning because edge nodes are constituted by the Road Side Unit method and collaborative learning are described in Section IV. (RSU) [3]. Results and analyzes are discussed in Section V. A conclusion The edge computing layer indeed solves the above is highlighted at the end of this article. problems, but other difficulties are bred as (1) increase in the II. LITERATURE REVIEW amount of data, (2) an increase in the amount of data types, (3) difficult to adapt existing data collection related to This section attempts to summarize the latest research embedded data, (4) need for intelligent methods to collect data, related to the Internet of Vehicles based on the following and (5) need for smart methods for the transmission [4]. directives: (1) The assessment of the quality of services, (2) occlusion detection. Deep learning methods provide a solution for the above difficulty. They ensure the simulation of the data analysis to 231 | P a g e www.ijacsa.thesai.org (IJACSA) International Journal of Advanced Computer Science and Applications, Vol. 12, No. 3, 2021 A. Quality of Service Assessment III. PROPOSED IOV ARCHITECTURE SCHEME The safety of the road and the crowd's reduction is We introduce the proposed IoV architecture for the enhanced according to the evaluation of the QoS [7]. Van der collection and transmission of data on an edge computing layer Lee et al., [8] propose a scheduling method to evaluate the during this section. This architecture is composed of four sub- vehicle network QoS. This method was named a Time layers, as described in Fig. 1. Synchronized Channel Hopping (TSCH). It introduces the interference diagram, which is dependent on internal Data collection layer: This layer is built based on nodes. interference and conflict. This attempt provides accurate RSUs collect data related to vehicles and roads as vehicle analyzes of the network performance. The ratio of packet location and traffic information [22]. These data are sent reception and latency compose the performance metrics. continuously to the distributed intelligence layer through edge devices. Zhang et al., [9] introduce an open access geometry-based efficient propagation model. This model is composed of a Distributed intelligence layer: This layer aims to ensure connected vehicle in the traffic network that analyzes the QoS preprocessing and data analysis received from the data according to the principal component, multidimensional collection layer. This intermediate layer between RSU and scaling, and variance. The QoS is carrying out for vehicle- centralized cloud computing provides a powerful step to infrastructure and vehicle-vehicle. increase storage capabilities, share communication resources, and improve computing. The distributed intelligence layer is B. Occlusion Detection in IoV Systems responsible for data transfer methods and the network's Avoid occlusion in traffic is the subject of many related evaluation [23]. works. Determine the density of the crowded in urban is still Data processing layer: In this layer, the collecting sensing challenged [10]. The process aims to detect vehicles and data are trained. Three functions are verified in this step: exploit the crowded density. This is ensured through (1) Detect the data quality, (2) Detect data similarity, and preprocessing, motion detection, feature extraction, and (3) Detect relevant data. This step decreases the amount of data classification steps [11]. There are many traditional and new transmitted to the cloud. Only the training results are sent to the techniques used for classification [12]. Deep learning is the cloud instead of direct transmission to the centralized cloud. kernel of these new approaches like CNN approaches. The data processing layer reduces delays related to In the traditional approaches, features are determined communications and increases the privacy level [24]. manually, and the classification step computes the similarity according to these predetermined features. For example, the authors in [24] applied HOG algorithm to detect humans. Hadjkacem et al. [13] used Gait-Appearance-based Multi- Scale Video Covariance (GAMS-ViCov) to detect a pedestrian. The estimation of a high-dense crowd through images is the main subject discussed in [14]. The author used Balanced Communication-Avoiding Support Vector Machine classifier for the detection. In [15], the authors are focused on the case of the detection of vehicles by applying Harr-like and Adaboost features. The limitation of representation is the main issue of the traditional approaches. In the deep learning approach, features are determined automatically according to the provided dataset. This approach offers a broad representation ability [16]. We focus on related works based on CNN with two-stage because it enriches higher performance compared to the one-stage approach [17][18]. The CNN-two-stage includes a region-based convolutional neural network [19]. Martinez et al., [19] perform CNN as a selective search. The purpose is to detect two thousand candidate regions through a fixed-sized input image. The obtained result in terms of performance is higher than the traditional approach but this method is time-consuming. The authors in [20] and [21] attempt to reduce the time consuming. They used the selective once (SPP-Net method) to remove it (Fast R-CNN method) as described in [21]. This led to announce the region network method instead of selective search. The region network ensures convolutional operation and sharing computation. These advancements provide a higher accuracy at minimum time-consuming. Fig. 1. Proposed IoV Architecture. 232 | P a g e www.ijacsa.thesai.org (IJACSA)
Details
-
File Typepdf
-
Upload Time-
-
Content LanguagesEnglish
-
Upload UserAnonymous/Not logged-in
-
File Pages6 Page
-
File Size-