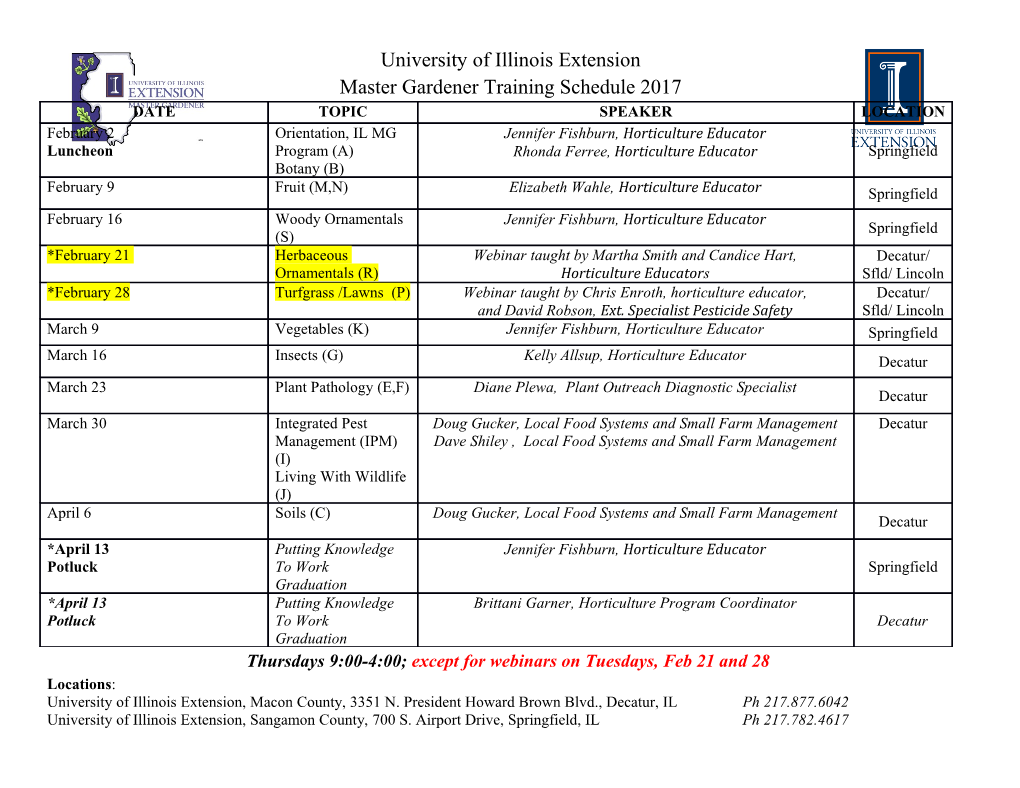
Learning Algorithms, and current developments – Banking at the Crossroads Essays on Machine learning / Applied Data Analytics, Asset Encumbrance / Bail-in, Sustainability and Resource Availability Dissertation zur Erlangung des akademischen Grades Doctor rerum politicarum an der Fakultät Wirtschaftswissenschaften Technische Universität Dortmund Dipl. Wi-Ing. Joachim Erhardt London, December 2017 Acknowledgments This PhD thesis was supported by Landesbank Baden-Württemberg. I am grateful to Professor Dr. Peter N. Posch for his ongoing encouragement and valued advice. I would like to express thanks to my team, particularly to Dr. Rainer Dürr, Tarek Hard (PhD) and David West for their support and modelling work over the years. I am thankful for having had the opportunity to supervise the master theses of Mark Wolters, Johannes Luebbers, Bernd Schumacher and Patrick Kosa. Last but not least, I would like to thank my wife and especially our daughter for their mostly unwavering patience. ii Contents Contents ................................................................................................................................... iii 1. Introduction ........................................................................................................................ 1 2. Efficiency Impact through Data Analytics ......................................................................... 4 2.1. Introduction ................................................................................................................. 4 2.2. Machine Learning – Elements of Choice .................................................................... 5 2.3. Application – Market Trends .................................................................................... 27 2.4. Application – Institutional Customer Behaviour ...................................................... 62 2.5. Summary and Conclusion - the use of Learning Algorithms in Finance .................. 76 3. Bail-In and Asset Encumbrance: Implications for Banks’ Asset Liability Management . 81 3.1. Introduction ............................................................................................................... 81 3.2. Data Description ........................................................................................................ 83 3.3. Simulation Setup ....................................................................................................... 90 3.4. Effect of Asset Encumbrance on Costs ..................................................................... 94 3.5. Conclusion ............................................................................................................... 100 4. Sustainability, Green Bonds and Ethics ......................................................................... 101 4.1. Introduction ............................................................................................................. 101 4.2. Business Case for Investing under ESG – Economic Return.................................. 103 4.3. Conclusions on ESG - and Social Return................................................................ 107 4.4. Green Bond Solution ............................................................................................... 110 5. The Impact of Commodity Finance on Resource Availability ....................................... 113 5.1. Introduction ............................................................................................................. 113 5.2. Empirical Analysis .................................................................................................. 114 5.3. Conclusion ............................................................................................................... 117 6. Concluding Summary ..................................................................................................... 119 7. Literature ........................................................................................................................ 124 8. Appendix ........................................................................................................................ 8-1 8.1. Appendix on Elliott Wave Theory .......................................................................... 8-1 8.2. Appendix on Trend Prediction ................................................................................ 8-7 8.3. Appendix on Institutional Customer Behaviour.................................................... 8-40 iii List of Tables Table 1: Classic Feature set ..................................................................................................... 32 Table 2: The Landmark Feature set ......................................................................................... 34 Table 3: List of Residuals and ‘Landmark light’ Features ...................................................... 44 Table 4: Confusion Matrix – NKY, Multi-asset with Granger Causality and Classic Features. .................................................................................................................................................. 45 Table 5: Prediction statistics – Overview across models for NKY, DAX, and SPX Index. .... 47 Table 6: Confusion Matrix – UKX, Multi-asset with Granger Causality and ‘Residuals & Landmark light’ Features. ........................................................................................................ 50 Table 7: Confusion Matrix – DAX, Multi-asset with Granger Causality and ‘Residuals & Landmark light’ Features. ........................................................................................................ 50 Table 8: Confusion Matrix – SPX, Single-asset with Landmark Features. ............................. 52 Table 9: Confusion Matrix – DAX with XGBoost, Multi-asset with Granger Causality and Residuals. ................................................................................................................................. 54 Table 10: Confusion Matrix – DAX with Random Forest, Multi-asset with Granger Causality and Residuals. .......................................................................................................................... 54 Table 11: Comparison of Prediction Statistics according to Customer Activity Ranking. ..... 72 Table 12: Funding composition of the bank sample. ............................................................... 85 Table 13: Simple average over-collateralisation levels for covered bonds. ............................ 86 Table 14: The level of asset encumbrance (AE) and deposits. ................................................ 87 Table 15: Approximated funding curves based on simple Z spreads. ..................................... 89 Table 16: Shown are the percentage share of covered bonds (Covered) based on a 2% asset loos scenario............................................................................................................................. 95 Table 17: Shown are the percentage share of covered bonds (Covered) based on a 10% asset loss scenario. ............................................................................................................................ 97 Table 18: Shown are the percentage share of covered bonds (Covered) based on a 20% asset loss scenario. ............................................................................................................................ 98 Table 19: Fixed Effects Regression of Import Changes, worldwide ..................................... 116 Table 20: Fixed Effects Regression of Import Changes, European Union ............................ 117 Table 21: Calculation of reversal prices based on Fibonacci multiples................................. 8-1 Table 22: Assigned probabilities for alternative multiples, ................................................... 8-1 Table 23: Order of preference for wave patterns in ‘up- and down-trends’. ......................... 8-3 Table 24: EW-Features used as modelling set ....................................................................... 8-5 iv Table 25 (cont.): EW-Features used as modelling set ........................................................... 8-6 Table 26: List of Leading Markets – out of 300 assets - based on Granger Causality test: .. 8-7 Table 27: Confusion Matrix NKY - Single time series with ‘Classic Features’. ................ 8-14 Table 28: Confusion Matrix NKY – Single time series with ‘Landmarks’. ........................ 8-15 Table 29: Confusion Matrix NKY – Single time series with ‘EW Features’. ..................... 8-16 Table 30a, 30b: Confusion Matrix & Performance Statistics NKY – Multi-asset with Granger and ‘Landmarks’. ................................................................................................................. 8-16 Table 31a, 31b: Confusion Matrix & Performance Statistics NKY – Multi-asset with Granger and ‘EW-Features’. .............................................................................................................. 8-17 Table 32a, 32b: Confusion Matrix & Performance Statistics NKY – Multi-asset with Granger and ‘Residuals’..................................................................................................................... 8-18 Table 33a, 33b: Confusion Matrix & Performance Statistics NKY – Multi-asset without Granger and ‘Residuals’. ..................................................................................................... 8-19 Table 34a, 34b: Confusion Matrix & Performance
Details
-
File Typepdf
-
Upload Time-
-
Content LanguagesEnglish
-
Upload UserAnonymous/Not logged-in
-
File Pages188 Page
-
File Size-