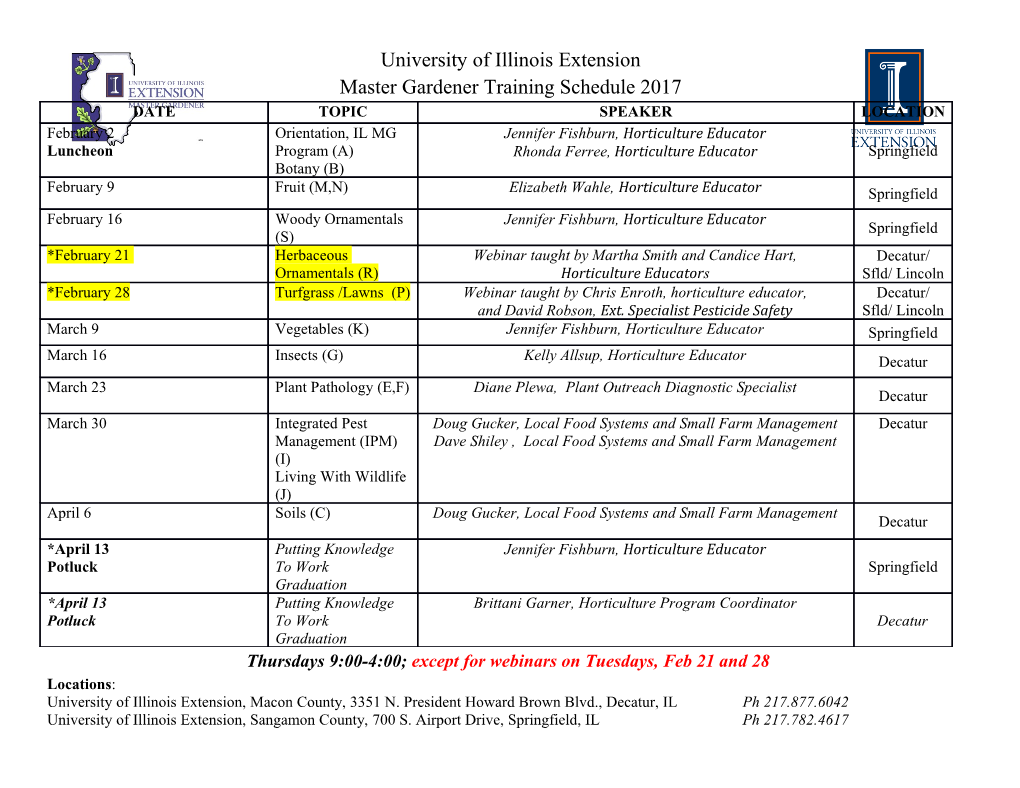
Julia Language Documentation Release 0.4.6 Jeff Bezanson, Stefan Karpinski, Viral Shah, Alan Edelman, et al. October 24, 2016 Contents I The Julia Manual1 1 Introduction 3 2 Getting Started 5 3 Variables 9 4 Integers and Floating-Point Numbers 13 5 Mathematical Operations and Elementary Functions 25 6 Complex and Rational Numbers 35 7 Strings 41 8 Functions 55 9 Control Flow 65 10 Scope of Variables 81 11 Types 89 12 Methods 109 13 Constructors 119 14 Conversion and Promotion 129 15 Interfaces 135 16 Modules 141 17 Documentation 149 18 Metaprogramming 157 19 Multi-dimensional Arrays 173 20 Linear algebra 185 i 21 Networking and Streams 189 22 Parallel Computing 195 23 Date and DateTime 209 24 Interacting With Julia 217 25 Running External Programs 221 26 Calling C and Fortran Code 227 27 Embedding Julia 243 28 Packages 251 29 Package Development 259 30 Profiling 271 31 Memory allocation analysis 277 32 Performance Tips 279 33 Workflow Tips 299 34 Style Guide 301 35 Frequently Asked Questions 309 36 Noteworthy Differences from other Languages 321 37 Unicode Input 329 II The Julia Standard Library 331 38 Essentials 333 39 Collections and Data Structures 349 40 Mathematics 367 41 Numbers 389 42 Strings 397 43 Arrays 405 44 Tasks and Parallel Computing 419 45 Linear Algebra 427 46 Constants 453 47 Filesystem 455 48 I/O and Network 459 ii 49 Punctuation 471 50 Sorting and Related Functions 473 51 Package Manager Functions 479 52 Dates and Time 483 53 Unit and Functional Testing 491 54 C Interface 495 55 LLVM Interface 499 56 C Standard Library 501 57 Dynamic Linker 503 58 Profiling 505 Bibliography 507 iii iv Part I The Julia Manual 1 CHAPTER 1 Introduction Scientific computing has traditionally required the highest performance, yet domain experts have largely moved to slower dynamic languages for daily work. We believe there are many good reasons to prefer dynamic languages for these applications, and we do not expect their use to diminish. Fortunately, modern language design and compiler techniques make it possible to mostly eliminate the performance trade-off and provide a single environment productive enough for prototyping and efficient enough for deploying performance-intensive applications. The Julia programming language fills this role: it is a flexible dynamic language, appropriate for scientific and numerical computing, with performance comparable to traditional statically-typed languages. Because Julia’s compiler is different from the interpreters used for languages like Python or R, you may find that Julia’s performance is unintuitive at first. If you find that something is slow, we highly recommend reading through the Performance Tips (page 279) section before trying anything else. Once you understand how Julia works, it’s easy to write code that’s nearly as fast as C. Julia features optional typing, multiple dispatch, and good performance, achieved using type inference and just-in- time (JIT) compilation, implemented using LLVM. It is multi-paradigm, combining features of imperative, functional, and object-oriented programming. Julia provides ease and expressiveness for high-level numerical computing, in the same way as languages such as R, MATLAB, and Python, but also supports general programming. To achieve this, Julia builds upon the lineage of mathematical programming languages, but also borrows much from popular dynamic languages, including Lisp, Perl, Python, Lua, and Ruby. The most significant departures of Julia from typical dynamic languages are: • The core language imposes very little; the standard library is written in Julia itself, including primitive operations like integer arithmetic • A rich language of types for constructing and describing objects, that can also optionally be used to make type declarations • The ability to define function behavior across many combinations of argument types via multiple dispatch • Automatic generation of efficient, specialized code for different argument types • Good performance, approaching that of statically-compiled languages like C Although one sometimes speaks of dynamic languages as being “typeless”, they are definitely not: every object, whether primitive or user-defined, has a type. The lack of type declarations in most dynamic languages, however, means that one cannot instruct the compiler about the types of values, and often cannot explicitly talk about types at all. In static languages, on the other hand, while one can — and usually must — annotate types for the compiler, types exist only at compile time and cannot be manipulated or expressed at run time. In Julia, types are themselves run-time objects, and can also be used to convey information to the compiler. While the casual programmer need not explicitly use types or multiple dispatch, they are the core unifying features of Julia: functions are defined on different combinations of argument types, and applied by dispatching to the most specific matching definition. This model is a good fit for mathematical programming, where it is unnatural for the first 3 Julia Language Documentation, Release 0.4.6 argument to “own” an operation as in traditional object-oriented dispatch. Operators are just functions with special notation — to extend addition to new user-defined data types, you define new methods for the + function. Existing code then seamlessly applies to the new data types. Partly because of run-time type inference (augmented by optional type annotations), and partly because of a strong focus on performance from the inception of the project, Julia’s computational efficiency exceeds that of other dynamic languages, and even rivals that of statically-compiled languages. For large scale numerical problems, speed always has been, continues to be, and probably always will be crucial: the amount of data being processed has easily kept pace with Moore’s Law over the past decades. Julia aims to create an unprecedented combination of ease-of-use, power, and efficiency in a single language. In addition to the above, some advantages of Julia over comparable systems include: • Free and open source (MIT licensed) • User-defined types are as fast and compact as built-ins • No need to vectorize code for performance; devectorized code is fast • Designed for parallelism and distributed computation • Lightweight “green” threading (coroutines) • Unobtrusive yet powerful type system • Elegant and extensible conversions and promotions for numeric and other types • Efficient support for Unicode, including but not limited to UTF-8 • Call C functions directly (no wrappers or special APIs needed) • Powerful shell-like capabilities for managing other processes • Lisp-like macros and other metaprogramming facilities 4 Chapter 1. Introduction CHAPTER 2 Getting Started Julia installation is straightforward, whether using precompiled binaries or compiling from source. Download and install Julia by following the instructions at http://julialang.org/downloads/. The easiest way to learn and experiment with Julia is by starting an interactive session (also known as a read-eval-print loop or “repl”) by double-clicking the Julia executable or running julia from the command line: $ julia _ _ _ _(_)_ | A fresh approach to technical computing (_) | (_) (_) | Documentation: http://docs.julialang.org _ _ _| |_ __ _ | Type "?help" for help. | | | | | | |/ _` | | | | |_| | | | (_| | | Version 0.4.3 (2016-01-12 21:37 UTC) _/ |\__'_|_|_|\__'_| | |__/ | x86_64-apple-darwin13.1.0 julia> 1 + 2 3 julia> ans 3 To exit the interactive session, type ^D — the control key together with the d key or type quit(). When run in interactive mode, julia displays a banner and prompts the user for input. Once the user has entered a complete expression, such as 1 + 2, and hits enter, the interactive session evaluates the expression and shows its value. If an expression is entered into an interactive session with a trailing semicolon, its value is not shown. The variable ans is bound to the value of the last evaluated expression whether it is shown or not. The ans variable is only bound in interactive sessions, not when Julia code is run in other ways. To evaluate expressions written in a source file file.jl, write include("file.jl"). To run code in a file non-interactively, you can give it as the first argument to the julia command: $ julia script.jl arg1 arg2... As the example implies, the following command-line arguments to julia are taken as command-line arguments to the program script.jl, passed in the global constant ARGS. ARGS is also set when script code is given using the -e option on the command line (see the julia help output below). For example, to just print the arguments given to a script, you could do this: $ julia -e 'for x in ARGS; println(x); end' foo bar foo bar 5 Julia Language Documentation, Release 0.4.6 Or you could put that code into a script and run it: $ echo 'for x in ARGS; println(x); end' > script.jl $ julia script.jl foo bar foo bar The -- delimiter can be used to separate command-line args to the scriptfile from args to Julia: $ julia--color=yes-O-- foo.jl arg1 arg2.. Julia can be started in parallel mode with either the -p or the --machinefile options. -p n will launch an additional n worker processes, while --machinefile file will launch a worker for each line in file file. The machines defined in file must be accessible via a passwordless ssh login, with Julia installed at the same location as the current host. Each machine definition takes the form [count*][user@]host[:port] [bind_addr[:port]] . user defaults to current user, port to the standard ssh port. count is the number of workers to spawn on the node, and defaults to 1. The optional bind-to bind_addr[:port] specifies the ip-address and port that other workers should use to connect to this worker.
Details
-
File Typepdf
-
Upload Time-
-
Content LanguagesEnglish
-
Upload UserAnonymous/Not logged-in
-
File Pages531 Page
-
File Size-