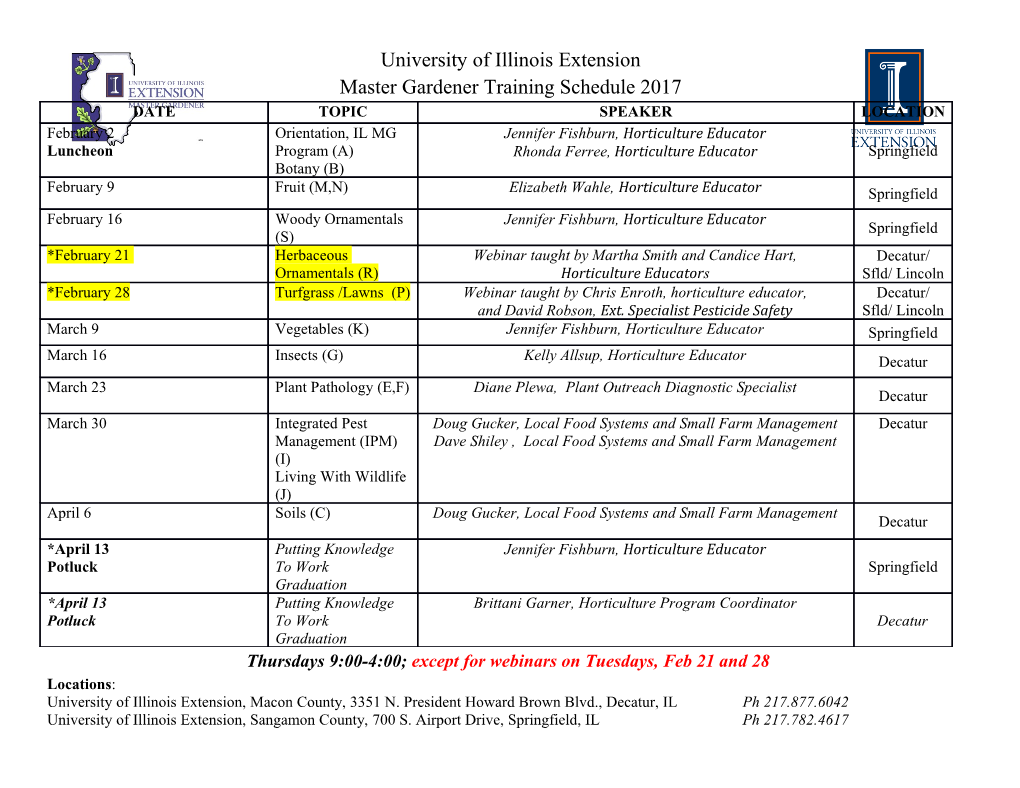
Resolving the Complexity of Some Fundamental Problems in Computational Social Choice A THESIS SUBMITTED FOR THE DEGREE OF Doctor of Philosophy IN THE Faculty of Engineering BY Palash Dey Computer Science and Automation Indian Institute of Science Bangalore { 560 012 (INDIA) August 2016 c Palash Dey August 2016 All rights reserved DEDICATED TO My teachers Acknowledgements I am grateful to my Ph.D. advisors Prof. Y. Narahari and Prof. Arnab Bhattacharyya for providing me a golden opportunity to work with them. I thank them for the all encompassing support they ushered upon me throughout my Ph.D. I convey my special thanks to Prof. Y. Narahari for all his academic and nonacademic supports which made my Ph.D. life very easy and enjoyable. I have throughly enjoyed working with Prof. Arnab Bhattacharyya. I convey my very special thanks to Prof. Neeldhara Misra for many excellent and successful research collaborations, useful discussions, and playing an all encompassing super-active role in my Ph.D. life. Prof. Neeldhara Misra was always my constant source of inspiration and she guided me throughout my Ph.D. as a supervisor, advisor, friend, and elder sister. I am indebted to Prof. Dilip P. Patil for teaching me how the practice of writing statements rigorously and precisely in mathematics automatically clarifies many doubts. I am extremely grateful to have a teacher like him at the very early stage of my stay in IISc who not only significantly improved my mathematical thinking process but also acted like a friend, philosopher, and guide throughout my stay in IISc. I throughly enjoyed all the courses that he taught during my stay in IISc and learned a lot from those courses. Specially his courses on linear algebra, Galois theory, commutative algebra have immensely influenced my thinking process. I thank Prof. Sunil Chandran for teaching me the art of proving results in graph theory in particular and theoretical computer science in general. I am grateful to Dr. Deeparnab Chakrabarty and Prof. Arnab Bhattacharyya for teaching me approximation algorithms and randomized algorithms, Prof. Manjunath Krishnapur for teaching me probability theory and martingales, Prof. Chandan Saha for teaching me complexity theory and algebraic geometry, Prof. Y. Narahari for teaching me game theory. I thank all the faculty members of Department of Computer Science and Automation (CSA) for all of their supports. I convey my special thank to all the staffs of CSA specially Ms. Suguna, Ms. Meenakshi, Ms. Kushael for their helping hands. I thank Prof. Ashish Goel for giving me an excellent opportunity to work with i Acknowledgements him and for hosting me in Stanford University for three months. I thank Dr. David Woodruff for collaborating with me on important problems. I feel myself extremely lucky to get such a wonderful and friendly lab members in the Game Theory lab. I want to thank all the members of the Game Theory lab with special mention to Dr. Rohith D. Vallam, Satyanath Bhat, Ganesh Ghalme, Shweta Jain, Swapnil Dhamal, Divya Padmanabhan, Dr. Pankaj Dayama, Praful Chandra, Shourya Roy, Tirumala Nathan, Debmalya Mandal, Arupratan Ray, and Arpita Biswas. I want to thank Dr. Minati De, Abhiruk Lahiri, Achintya Kundu, Aniket Basu Roy, Prabhu Chandran, Prasenjit Karmakar, R Kabaleeshwaran, Rohit Vaish, Sayantan Mukherjee, Srinivas Karthik, Suprovat Ghoshal, Vineet Nair for all the important discussions. I greatly acknowledge support from Ratul Ray and Uddipta Maity during my internship in Stanford University. I thank Google India for providing me fellowship during second and third years of my Ph.D. I acknowledge financial support from Ministry of Human Resource Development (MHRD) in India during my first year of Ph.D. Last but not the least, I express my gratitude to my parents, brother, wife, and all my friends for their love, blessing, encouragement, and support. ii Abstract In many real world situations, especially involving multiagent systems and artificial intel- ligence, participating agents often need to agree upon a common alternative even if they have differing preferences over the available alternatives. Voting is one of the tools of choice in these situations. Common and classic applications of voting in modern applications in- clude collaborative filtering and recommender systems, metasearch engines, coordination and planning among multiple automated agents etc. Agents in these applications usually have computational power at their disposal. This makes the study of computational aspects of voting crucial. This thesis is devoted to a study of computational complexity of several fundamental algorithmic and complexity-theoretic problems arising in the context of voting theory. The typical setting for our work is an “election”; an election consists of a set of voters or agents, a set of alternatives, and a voting rule. The vote of any agent can be thought of as a ranking (more precisely, a complete order) of the set of alternatives. A voting profile comprises a collection of votes of all the agents. Finally, a voting rule is a mapping that takes as input a voting profile and outputs an alternative, which is called the “winner” or “outcome” of the election. Our contributions in this thesis can be categorized into three parts and are described below. Part I: Preference Elicitation. In the first part of the thesis, we study the problem of elicit- ing the preferences of a set of voters by asking a small number of comparison queries (such as who a voter prefers between two given alternatives) for various interesting domains of preferences. We commence with considering the domain of single peaked preferences on trees in Chap- ter3. This domain is a significant generalization of the classical well studied domain of sin- gle peaked preferences. The domain of single peaked preferences and its generalizations are iii Abstract hugely popular among political and social scientists. We show tight dependencies between query complexity of preference elicitation and various parameters of the single peaked tree, for example, number of leaves, diameter, path width, maximum degree of a node etc. We next consider preference elicitation for the domain of single crossing preference pro- files in Chapter4. This domain has also been studied extensively by political scientists, social choice theorists, and computer scientists. We establish that the query complexity of pref- erence elicitation in this domain crucially depends on how the votes are accessed and on whether or not any single crossing ordering is a priori known. Part II: Winner Determination. In the second part of the thesis, we undertake a study of the computational complexity of several important problems related to determining winner of an election. We begin with a study of the following problem: Given an election, predict the winner of the election under some fixed voting rule by sampling as few votes as possible. We establish optimal or almost optimal bounds on the number of votes that one needs to sample for many commonly used voting rules when the margin of victory is at least "n (n is the number of voters and " is a parameter). We next study efficient sampling based algorithms for estimating the margin of victory of a given election for many common voting rules. The margin of victory of an election is a useful measure that captures the robustness of an election outcome. The above two works are presented in Chapter5. In Chapter6, we design an optimal algorithm for determining the plurality winner of an election when the votes are arriving one-by-one in a streaming fashion. This resolves an intriguing question on finding heavy hitters in a stream of items, that has remained open for more than 35 years in the data stream literature. We also provide near optimal algorithms for determining the winner of a stream of votes for other popular voting rules, for example, veto, Borda, maximin etc. Voters’ preferences are often partial orders instead of complete orders. This is known as the incomplete information setting in computational social choice theory. In an incomplete information setting, an extension of the winner determination problem which has been stud- ied extensively is the problem of determining possible winners. We study the kernelization complexity (under the complexity-theoretic framework of parameterized complexity) of the possible winner problem in Chapter7. We show that there do not exist kernels of size that is polynomial in the number of alternatives for this problem for commonly used voting rules iv Abstract under a plausible complexity theoretic assumption. However, we also show that the problem of coalitional manipulation which is an important special case of the possible winner prob- lem admits a kernel whose size is polynomially bounded in the number of alternatives for common voting rules. Part III: Election Control. In the final part of the thesis, we study the computational com- plexity of various interesting aspects of strategic behavior in voting. First, we consider the impact of partial information in the context of strategic manipu- lation in Chapter8. We show that lack of complete information makes the computational problem of manipulation intractable for many commonly used voting rules. In Chapter9, we initiate the study of the computational problem of detecting possible instances of election manipulation. We show that detecting manipulation may be computa- tionally easy under certain scenarios even when manipulation is intractable. The computational problem of bribery is an extensively studied problem in computational social choice theory. We study computational complexity of bribery when the briber is “fru- gal” in nature. We show for many common voting rules that the bribery problem remains intractable even when the briber’s behavior is restricted to be frugal, thereby strengthening the intractability results from the literature. This forms the subject of Chapter 10. v Publications based on this Thesis 1. Palash Dey, Neeldhara Misra, and Yadati Narahari. “Kernelization Complexity of Possible Winner and Coalitional Manipulation Problems in Voting”.
Details
-
File Typepdf
-
Upload Time-
-
Content LanguagesEnglish
-
Upload UserAnonymous/Not logged-in
-
File Pages306 Page
-
File Size-