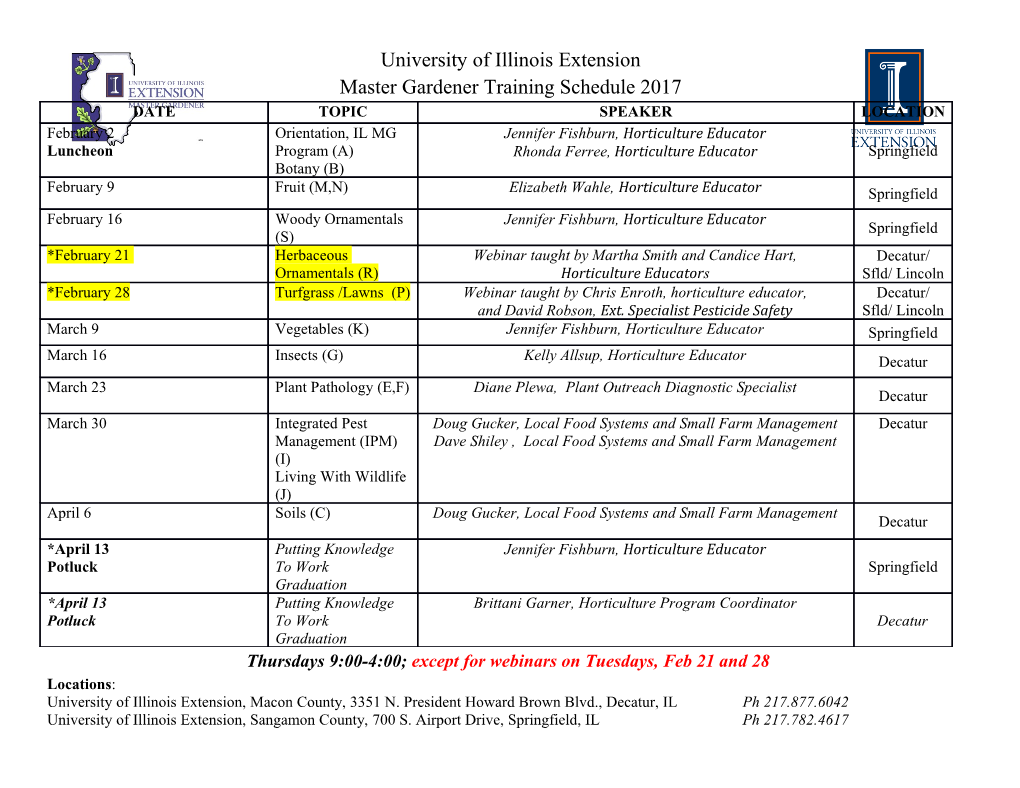
THE INDEX OF DISPERSION By EDGAR G. AVELINO B.Sc, The University of British Columbia, 1978 A THESIS SUBMITTED IN PARTIAL FULFILLMENT OF THE REQUIREMENTS FQR THE DEGREE OF MASTER OF SCIENCE in THE FACULTY OF GRADUATE STUDIES , Statistics Department The University of British Columbia We accept this thesis as conforming to the_ requifibd/t stan'cQrd' THE UNIVERSITY OF BRITISH COLUMBIA November 1934 © Edgar G. Avelino, 1984 In presenting this thesis in partial fulfilment of the requirements for an advanced degree at the University of British Columbia, I agree that the Library shall make it freely available for reference and study. I further agree that permission for extensive copying of this thesis for scholarly purposes may be granted by the head of my department or by his or her representatives. It is understood that copying or publication of this thesis for financial gain shall not be allowed without my written permission. Department of GTA7~/S7?&' The University of British Columbia 1956 Main Mall Vancouver, Canada V6T 1Y3 Date 'OCT- A3, /<?8y DE-6 (3/81) i i ABSTRACT The index of dispersion is a statistic commonly used to detect departures from randomness of count data. Under the hypothesis of randomness, the true distribution of this statistic is unknown. The accu• racy of large sample approximations is assessed by a Monte Carlo simulation. Further approximations by Pearson curves and infinite series expansions are in• vestigated. Finally, the powers of the individual tests based on the likelihood ratio, the index of dis• persion and Pearson's goodness-of-fit statistic are compared. i i i TABLE OF CONTENTS PAGE ABSTRACT ii ACKNOWLEDGEMENT ix 1. INTRODUCTION 1.1 History of the Index of Dispersion 1 1.2 Purpose of the Paper 4 2. LARGE SAMPLE APPROXIMATIONS 2.1 Joint Distribution of X & S2 6 2.2 The Asymptotic Distribution of I 7 2.3 Description of the Monte Carlo Simulation 9 2.4 The x2 Approximation 14 2.5 Discussion 15 3. PEARSON CURVES 3.1 The Theory of Pearson Curves 19 3.2 Two Examples 21 3.3 The First Four Moments of I 24 3.4 Discussion 29 iv PAGE 4. THE GRAM-CHARLIER SERIES OF TYPE A 4.1 The Theory of Gram-Charlier Expansions 37 4.2 Discussion 42 5. THE LIKELIHOOD RATIO AND GOODNESS-OF-FIT TESTS 5.1 The Likelihood Ratio Test 49 5.2 Pearson's Goodness-of-Fit Test 54 5.3 Power Computations 55 5.4 The Likelihood Ratio Test Revisited 65 6. GONGLUSIONS 74 REFERENCES .....76 APPENDIX Al.l The Conditional Distribution of a Poisson Sample Given the Total 79 A1.2 The First Four Moments of I 80 A1.3 The Type VI Pearson Curve 87 A1.4 A Limiting Case of the Negative Binomial 88 A2.1 Histograms of I 90 A2.2 Empirical Critical Values 106 A2.3 Pearson Curve Critical Values 107 A2.4 Gram-Charlier Critical Values 109 V TABLE OF CONTENTS LIST OF TABLES PAGE TABLE 1: Expected Number of I*'s 10 TABLE 2 (A-D): Normal Approximation 11 2 TABLE 3 (A-D): x Approximation 16 TABLE 4 (A-D): Pearson Curve Fit with Exact Moments 31 TABLE 5 (A-D): Pearson Curve Fit with Asymptotic Moments 33 TABLE 6 (A-D): Gram-Charlier Three-Moment Fit (Exact) 43 TABLE 7 (A-D): Gram-Charlier Four-Moment Fit (Exact) 45 TABLE 8: Asymptotic Power of the Index of Dispersion Test -. 57 TABLE 9-10 (A-D): Power of Tests Based on A,I and X2 60 TABLE 11 (A-D): Power of Tests Based on A and I (n=50).. 66 TABLE 12: Number of Times (n-l)S2 ^ nX 68 TABLE 13 (A-B): Power of Tests Based on A,I and X2 (n=10) 70 TABLE 14 (A-B): Power of Tests Based on A,I and X2 (n=20) 71 vi PAGE TABLE 15 (A-B): Power of Tests Based on A and I (n=50).. 72 2 TABLE Al: The Ratios of the Moments of I and x n_! 86 TABLE A2: Emperical Critical Values of I (Based on 15,000 Samples) 106 TABLE A3: Pearson Curve Critical Values (Exact) 107 TABLE A4: Pearson Curve Critical Values (Asymptotic) 108 TABLE A5: Gram-Charlier Critical Values (Three Exact Moments) 109 TABLE A6: Gram-Charlier Critical Values (Three Asymptotic Moments) 110 TABLE A7: Gram-Charlier Critical Values (Four Exact Moments) Ill TABLE A8: Gram-Charlier Critical Values (Four Asymptotic Moments) 112 vii TABLE OF CONTENTS LIST OF FIGURES PAGE FIG. A.l: Histogram of I (1000 Samples, n = 10, X = 3) 90 FIG. A.2: Histogram of I (1000 Samples, n = 10, X = 5) 91 FIG. A.3: Histogram of I (1000 Samples, n = 20, X = 3) 92 FIG. A.4: Histogram of I (1000 Samples, n = 20, X = 5) 93 FIG. A.5: Histogram of I (1000 Samples, n = 50, X = 3) 94 FIG. A.6: Histogram of I (1000 Samples, n = 50, X = 5) 95 FIG. A.7: Histogram of I (1000 Samples, n = 100, X = 3) 96 FIG. A.8: Histogram of I (1000 Samples, n = 100, X = 5) 97 FIG. A.9: Normal Probability Plot for I (1000 Samples, n = 10, X = 3) 9S v i i i PAGE FIG. A.10: Normal Probability Plot for I (1000 Samples, n = 10, x = 5) 9S FIG. A.11: Normal Probability Plot for I (1000 Samples, n = 20, x = 3) 100 FIG. A.12: Normal Probability 'Plot for I (1000 Samples, n = 20, x = 5) 101 FIG. A.13: Normal Probability Plot for I (1000 Samples, n = 50, X = 3) 102 FIG. A.14: Normal Probability Plot for I (1000 Samples, n = 50, X = 5) 103 FIG. Ail5: Normal Probability Plot for I (1000 Samples, n =.100, X = 3) 104 FIG. A.16: Normal Probability Plot for I (1000 Samples, n = 100, X = 5) 105 ix ACKNOWLEDGEMENT I wish to express my sincere appreciation to Prof. A. John Petkau who devoted precious time to the supervision of this thesis. Edgar G. Avelino 1 1. INTRODUCTION 1.1 HISTORY OF THE INDEX OF DISPERSION The index of dispersion is a test statistic often used to detect spatial pattern, a term ecologists use to describe non-randomness of plant populations. This is equivalent to testing that the growth of plants over an area is purely random, or equivalently that the number of plants in any given area has the Poisson distribution. Suppose then that we randomly partition some area by n disjoint equal-sized quadrats and make a count, x, of the number of plants in each quadrat. Under the hypothesis of randomness, Xj, ... , Xnwould have the Poisson distribution, P(X=x) = e'V/xl , for x > 0 and x = 0, 1, 2, ... , for which E(X) = Var(X) = X. For alternatives to complete randomness involving patches or clumping of plants, we would expect Var(X) > E(X), while for more regular spacing of plants, we would expect Var(X) < E(X) (see for example R.H. Green (1966)) . These properties lead quite naturally to considering the variance-to-mean ratio as a population index to measure spatial pattern. An estimator of the variance-to-mean ratio is the index of dispersion, defined as 1 , if I = 0 I = I s2/x if X > 0 n n where X = (1/n) F. X. and S2 = {l/(n-l)> z (X.-X)2, the i=l 1 i=l 1 2 unbiased estimators of E(X) and Var(X), respectively. (It is natural to define I to be 1 if X = 0 because under the null hypothesis, the variance-to-mean ratio equals 1.) Ever since G.E. Blackman (1935) used the Poisson model for counts of plants, the concept of randomness in a community of plants became a growing interest among ecologists. Although the index of dispersion was introduced by R.A. Fisher (Fisher, Thornton and MacKenzie, 1922), it was not until 1936 that it was first used by ecologists for the purpose of inference. A.R. Clapham (1936), using a x2 approximation for the distribution of the index of dispersion under the null hypothesis of randomness, found that among 44 plant species he studied, only four of these seemed to be distributed randomly, while over-dispersion (i.e. clumping) was clearly present for the remaining species. Student (1919) had already pointed out that the Poisson is not usually a good model for ecological data and in most cases, clumping occurs. This has been termed "contagious" by G. Polya (1930) and also by J. Neyman (1939). Ever since Clapham's paper, the use of the index of dispersion as a test of significance of departures from randomness has been extensive, not just for field data, but also in other areas (for example, blood counts, insect counts and larvae counts). Fisher et al (1922) showed that the distribution of the index of dispersion could be closely approximated by the x2 distribution with n-1 degrees of freedom. However, if the Poisson parameter, X» is small, or if the estimated expectation, X, is smal1, then the adequacy of the 3 x2 approximation becomes questionable. This is discussed by H.O. Lancaster (1952). Fisher (1950) and W.G. Cochran (1936) have pointed out that in this case, the test of randomness based on I should be done conditionally with given totals, £X.j. Since this sum is a sufficient statistic for the Poisson parameter X, conditioning on the total will yield a distribution independent of X. Hence, exact frequencies can be computed. The conditional moments of the index of dispersion are provided in Appendix A1.2.
Details
-
File Typepdf
-
Upload Time-
-
Content LanguagesEnglish
-
Upload UserAnonymous/Not logged-in
-
File Pages122 Page
-
File Size-