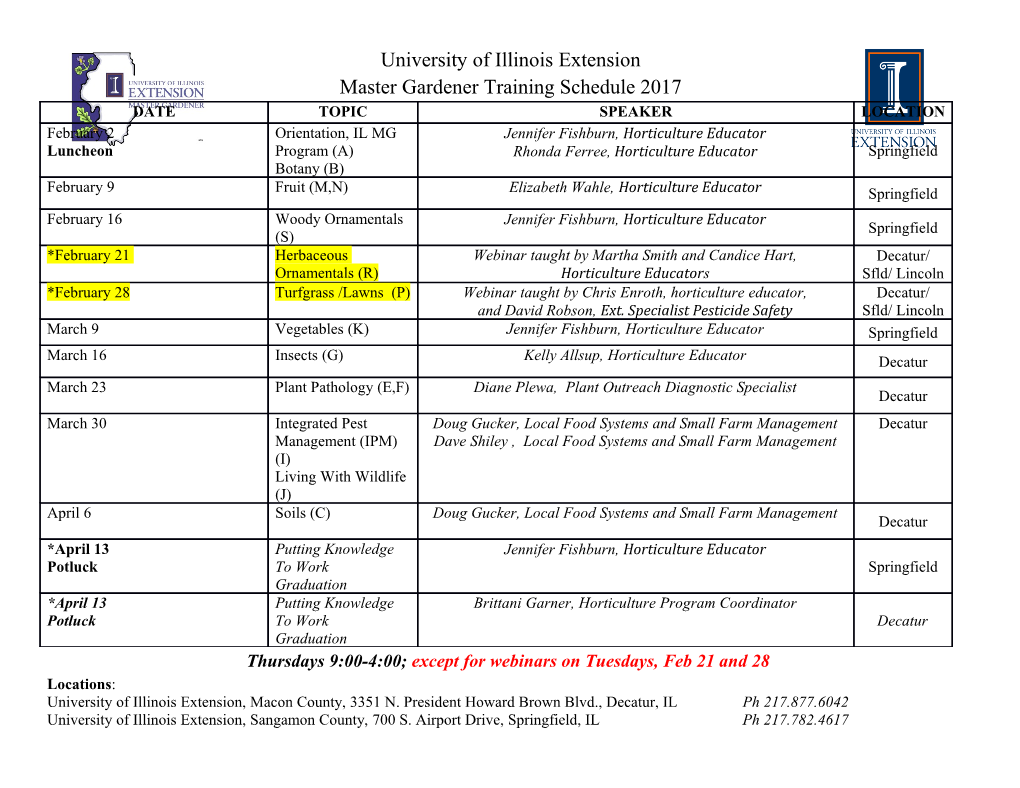
Comparative analysis of urban microbiomes using machine learning algorithms DIPLOMARBEIT ZUR ERLANGUNG DES AKADEMISCHEN GRADES MASTER OF SCIENCE IN ENGINEERING DER FACHHOCHSCHULE FH CAMPUS WIEN MASTER-STUDIENGANG BIOINFORMATIK Vorgelegt von: René Lenz Personenkennzeichen: c1810542004 FH-Hauptbetreuer*in: FH-Prof.in Mag.a Dr.in Alexandra Graf Abgabetermin: 19.11.2020 FH Campus Wien University of Applied Sciences/Fachbereich Bioengineering Erklärung: Ich erkläre, dass die vorliegende Diplomarbeit von mir selbst verfasst wurde und ich keine anderen als die angeführten Behelfe verwendet bzw. mich auch sonst keiner unerlaubter Hilfe bedient habe. Ich versichere, dass ich dieses Diplomarbeitsthema bisher weder im In- noch im Ausland (einer Beurteilerin/einem Beurteiler zur Begutach- tung) in irgendeiner Form als Prüfungsarbeit vorgelegt habe. Weiters versichere ich, dass die von mir eingereichten Exemplare (ausgedruckt und elektronisch) identisch sind. 19.11.2020 Datum Unterschrift FH Campus Wien University of Applied Sciences/Fachbereich Bioengineering Abstract Die Metagenomforschung ist in der Lage, Einblicke in die mikrobielle Zu- sammensetzung von Proben zu gewinnen. Mit Hilfe von Machine Learning Methoden ist es bis zu einem gewissen Grad möglich, unbekannte Proben ihrer Herkunft zuzuordnen. Verbindet man diese beiden Ansätze ergeben sich auch neue Felder für die Forensik. In dieser Arbeit wird untersucht, ob man Proben die in öffentlichen Verkehrsmitteln verteilt über den Globus ge- sammelt wurden, wieder den einzelnen Städten zugeordnet werden können und ob sich Schlüsselspezies herauskristalisieren. Die Whole Genome Sequencing Daten werden taxonomisch zugeordnet und die Häufigkeit der gefundenen Spezies ermittelt. Die Daten stammen aus 2016 und 2017. 4 Städte von 2016 und 9 Städte von 2017 werden analysiert. Es werden Vorhersagemodelle mit Random Forests, K-nearest Neighbors und Support Vector Machines erstellt. Das genaueste Modell hat jeweils der Random Forest geliefert, > 98% 2016, > 80% 2017. Die Arbeit zeigt, dass die Genauigkeit der Vorhersagemodelle erwartungs- gemäß mit der Anzahl an Städten sinkt. Die Randon Forest Modellen haben Spezies hervorgehoben, die das Potenzial haben, näher betrachtet zu wer- den. Die Analyse von Mystery Samples hat letzten Endes dann nicht so gut funktioniert, wie erwartet. Hier gibt es auf jeden Fall noch viel Potenzial zur Verbesserung. Metagenomic research is capable of creating insights into the microbial composition of samples. With the help of machine learning methods it is, to a certain degree, possible to assign unknown samples to their origin. Com- bining these two approaches also opens up new fields for forensics. In this thesis it is investigated whether samples collected in public transport sys- tems distributed around the globe can be assigned to their individual cities of origin and whether key species can be identified. The Whole Genome Sequencing data are taxonomically assigned and the abundance of the species found is determined. The data are derived from 2016 and 2017. 4 cities from 2016 and 9 cities from 2017 are analysed. Predictive models using random forests, K-nearest Neighbors and Support Vector Machines are developed. The most accurate model was provided by the random forest, > 98% in 2016, > 80% in 2017. The work shows that, as expected, the accuracy of the prediction models decreases with the number of cities. However, the Randon Forest models have identified a number of species that have the potential to be studied more thoroughly.In the end, the analysis of Mystery Samples did not work as well as expected. In any case, there is still much potential for improvement. 1 FH Campus Wien University of Applied Sciences/Fachbereich Bioengineering Acknowledgements Als erstes möchte ich meinen Betreuerinnen Alexandra und Theresa danken, für ihre fachliche Unterstützung über den gesamten Zeitraum der Masterarbeit und auch schon während des Studiums. Vielen Dank für das angenehme produktive Arbeitsklima! Der nächste Dank gebührt meinen Eltern Helene und Daniel, sowie ihren Lebenspartnern Gerd und Ulli, die die Hoffnung nie aufgegeben haben, dass aus mir irgendwann noch was wird. Ich möchte mich auch herzlich bei Jennifer bedanken. Du hast dich wirklich hervorragend um mein leibliches und seelisches Wohl gesorgt. Du bist mir immer mit Rat und Tat zur Seite gestanden! Vielen Dank dafür! Vielen Dank Eva und Alex, für die als „Think Tanks“ getarnten „Drink Tanks“. Etliche gute Ansätze wurden hier geboren und vernichtet. „Your code is wrong on so many levels that I barely know where to start.“ - Danke Perffizienz! 2 FH Campus Wien University of Applied Sciences/Fachbereich Bioengineering Contents 1 Introduction5 1.1 Machine Learning . .5 1.1.1 Learning Methods . .6 1.1.2 PCA - Principal Component Analysis . .6 1.1.3 Hierarchical Clustering . .7 1.1.4 RF - Random Forest . .8 1.1.5 KNN - k-Nearest neighbors . .8 1.1.6 Support Vector Machines . .8 1.2 Metagenomics . .9 1.3 Background . 10 1.3.1 MetaSub . 12 1.3.2 CAMDA . 14 2 Aim of this Work 16 3 Materials and Methods 17 3.1 Data origin . 17 3.2 Data availability . 19 3.3 Workflow . 19 3.3.1 From WGS data to species abundance . 19 3.3.2 Ecological assignment . 20 3.3.3 Abundance analysis and Machine learning . 21 3.4 Software . 24 3.5 Hardware . 24 4 Results 25 4.1 CSD17 . 25 4.1.1 PCA analysis . 26 4.1.2 Hierarchical Clustering . 28 4.1.3 Random Forest analysis . 29 4.1.4 K-nearest neighbors analysis . 34 4.1.5 Support Vector Machines analysis . 35 4.1.6 Accuracy comparison . 36 4.2 CSD16 . 38 4.2.1 PCA analysis . 40 4.2.2 Hierarchical Clustering . 41 4.2.3 Random Forest analysis . 42 4.2.4 K-nearest neighbors analysis . 47 4.2.5 Support Vector Machines analysis . 48 4.2.6 Accuracy comparison . 49 4.3 Matching species of Ilorin and New York (CSD16 vs. CSD17) . 51 4.4 Mystery samples . 52 5 Discussion 54 6 Glossary 58 3 FH Campus Wien University of Applied Sciences/Fachbereich Bioengineering List of Figures 1 General workflow . 21 2 CSD17 Species per data set . 26 3 CSD17 PCA example . 27 4 CSD17 Hierarchical Clustering . 28 5 CSD17 RF example 1 . 29 6 CSD17 RF example 2 . 30 7 CSD17 variable importance plot . 32 8 CSD17 KNN plots . 34 9 CSD17 SVM plots . 35 10 CSD16 Species per data set . 39 11 CSD16 PCA example . 40 12 CSD16 Hierarchical Clustering . 41 13 CSD16 RF example 1 . 42 14 CSD16 RF example 2 . 43 15 CSD16 variable importance plot . 44 16 CSD16 KNN plots . 47 17 CSD16 SVM plots . 48 List of Tables 1 Cities participating at the CSDs . 18 2 Mystery sample translation . 19 3 Data partitioning . 22 4 Used R software . 24 5 CSD17 explained variance . 27 6 CSD17 RF error table . 31 7 CSD17 most important variables . 33 8 CSD17 Parameter optimization . 36 9 CSD17 model accuracy incl. train sets . 37 10 CSD17 model accuracy incl. trest sets . 38 11 CSD16 explained variance . 41 12 CSD16 RF error table . 44 13 CSD16 most important variables . 46 14 CSD16 Parameter optimization . 49 15 CSD16 model accuracy incl. train sets . 50 16 CSD16 model accuracy incl. test sets . 51 17 No. of matching species of CSD17 vs. CSD16 . 51 18 Matching species of CSD17 vs. CSD16 . 52 19 Mystery samples right prediction . 52 20 Evaluation of the mystery samples . 53 4 FH Campus Wien University of Applied Sciences/Fachbereich Bioengineering 1 Introduction 1.1 Machine Learning Machine learning has actually been an important part of data analysis from the be- ginning of the computer age. In the 50s and 60s of the last century, when computers appeared on a larger scale, algorithms were developed or known approaches were further developed (Kononenko, 2001). One of the cornerstones was Baysian statistics (Bayes’ Theorem, 18th century). Further discoveries were the method of least squares (Legendre, 1805), Markov chains (Hayes, 2013) and the Turing machine (Turing, 1950). The idea behind machine learning is to make computers work in a similar way to the human brain. Nowadays so called Neural Networks (NN) imitate the way information is processed by neurons by passing the data from an input layer (of neurons) on to hidden layers and to the output layer (Lancashire et al., 2009). There is always training data, from which it is tried to recognize certain rules, processes and patterns. Conclusions are then drawn from these findings, the computer learns similar to humans. Tom M. Mitchell, a pioneer in the field of machine learning described this approach as follows: "A computer program is said to learn from experience E with respect to some class of tasks T and performance measure P if its performance at tasks in T, as measured by P, improves with experience E." There are three important methods of learning, namely "supervised learning", "unsu- pervised learning" and "semisupervised learning". In fact, depending on the charac- teristics, there are even further distinctions, but these can be roughly divided into the above categories. Information that can be obtained from the models includes clus- tering, classification, regression, detection of anomalies or recommendation engines1. Cluster analysis is based on unsupervised learning and groups the data according to their similarity. Methods are Hierarchical Clustering (HC), centroid based cluster- ing, distribution-based clustering or density clustering. If the task is to assign data to different clusters this is called classification2. Applied methods are decision trees, Random Forest (RF), Support Vector Machines (SVM), K-nearest neighbors (KNN), logistic regression just to name a few. To relate a dependent variable to one or more independent variables is called regression. Regression analysis can be divided in lin- ear and non linear regression. One must be careful when interpreting the results, as a regression makes relations visible, but does not establish causality (Freedman, 2009). 1https://www.innoarchitech.com/blog/machine-learning-an-in-depth-non-technical-guide, accessed: 08.11.2020 2https://www.stat.berkeley.edu/ s133/Class1a.html, accessed: 08.11.2020 5 FH Campus Wien University of Applied Sciences/Fachbereich Bioengineering Recommendation engines are often used in the marketing industry to analyse user habits and thus offer customised advertising.
Details
-
File Typepdf
-
Upload Time-
-
Content LanguagesEnglish
-
Upload UserAnonymous/Not logged-in
-
File Pages66 Page
-
File Size-