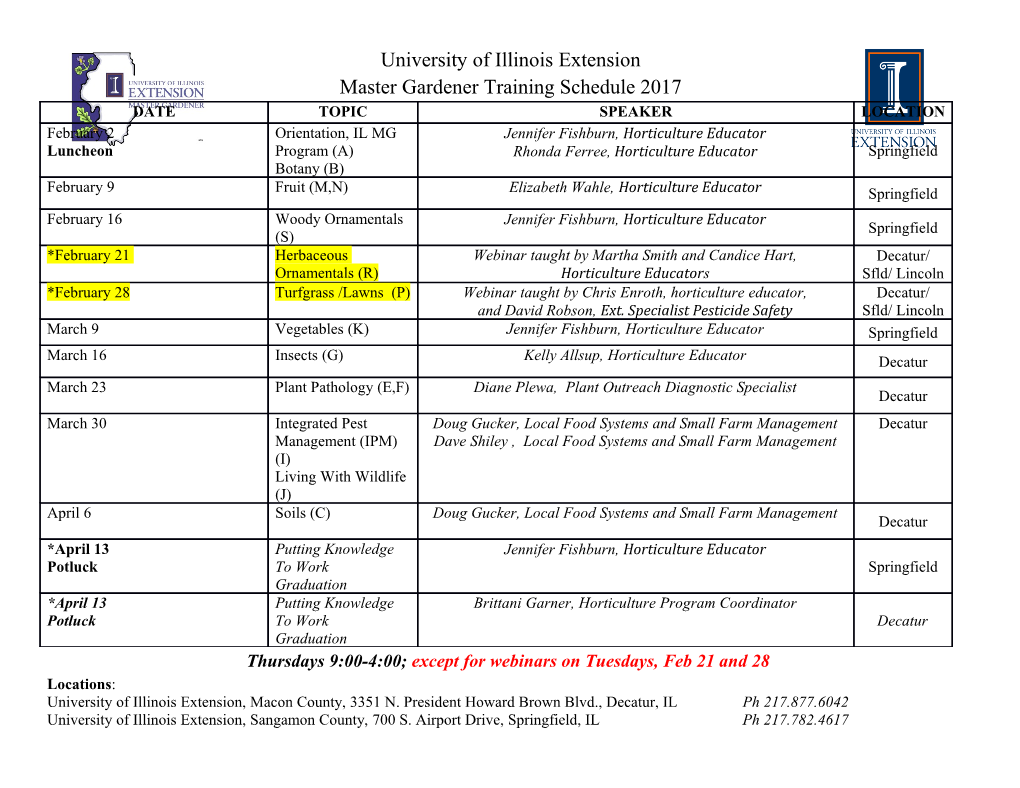
Hierarchical models { motivation James-Stein inference ² Suppose X » N(θ; 1) { X is admissible (not dominated) for estimating θ with squared error loss ² Now Xi » N(θi; 1); i = 1; : : : ; r { X = (X1;:::;Xr) is admissible if r = 1; 2 but not r ¸ 3 { for r ¸ 3 r ¡ 2 ±i = (1 ¡ P 2 )Xi i Xi yields better estimates { known as James-Stein estimation 57 Hierarchical models { motivation James-Stein inference (cont'd) ² Bayes view: Xi » N(θi; 1) and θi » N(0; a) { posterior distn: θijXi » N 1 { posterior mean is (1 ¡ a+1 )Xi { need to estimate a; one natural approach yields James-Stein ² Summary { estimation results depend on loss function { squared-error loss do well on avg but maybe poor for one component { powerful lesson about combining related problems to get improved inferences 58 Hierarchical Models Suppose we have data Yij j = 1;:::;J i = 1; : : : ; nj such that Yij i = 1; : : : ; nj are independent given θj with distribution p(Y jθj). e.g. |scores{z } for |students{z } in |classrooms{z } It might be reasonable Y (i) (j) to expect θj's to be \similar" (but not necessarily identical). Therefore, we may perhaps try to estimate population distribution of θj's. This is achieved in a natural way if we use a prior distribution in which the θj's are viewed as a sample from a common population distribution. 59 Hierarchical Models ² Key: The observed data, yij , with units indexed by i within groups indexed by j, can be used to estimate aspects of the population distribution of the θj's even though the values of θj are not themselves observed. ² How? It is natural to model such a problem hierarchically { observable outcomes modeled conditionally on parameters θ { θ given a probabilistic speci¯cation in terms of other parameters, Á, known as hyperparameters. 60 Hierarchical Models ² Nonhierarchical models are usually inappropiate for hierarchical data. { a single θ (i.e. θj ´ θ 8j) may be inadequate to ¯t a combined data set. { separate unrelated θj are likely to \over¯t" data. { information about one θj can be obtained from others' data. ² Hierarchical model uses many parameters but population distribution induces enough structure to avoid over¯tting. 61 Setting up hierarchical models Exchangeability Recall: A set of random variables (θ1; : : : ; θk) is exchangeable if the joint distribution is invariant to permutations of the indexes (1; : : : ; k). The indexes contain no information about the values of the random variables. ² hierarchical models often use exchangeable models for the prior distribution of model parameters ² iid random variables are one example ² seemingly non-exchangeable r.v.'s may become exchangeable if we condition on all available information (e.g., regression analysis) 62 Setting up hierarchical models Exchangeable models ² Basic form of exchangeable model { θ = (θ1; : : : ; θk) are independent conditional on additional parameters Á (known as hyperparameters) Yk p(θjÁ) = p(θjjÁ) j=1 { Á referred to as hyperparameter(s) with hyperprior distn p(Á) R { implies p(θ) = p(θjÁ)p(Á)dÁ { work with joint posterior distribution, p(θ; Ájy) ² One objection to exchangeable model is that we may have other information, say (Xj). In that case may take YJ p(θ1; : : : ; θJ jX1;:::;XJ ) = p(θijÁ; Xi) i=1 63 Setting up hierarchical models ² Model is speci¯ed in nested stages { sampling distribution p(yjθ) (¯rst level of hierarchy) { prior (or population) distribution for θ: p(θjÁ) (second level of hierarchy) { prior distribution for Á (hyperprior): p(Á) { Note: more levels are possible { hyperprior at highest level is often di®use but improper priors must be checked carefully to avoid improper posterior distributions. 64 Setting up hierarchical models ² Inference { Joint distn: p(y; θ; Á) = p(yjθ; Á)p(θjÁ)p(Á) = p(yjθ)p(θjÁ)p(Á) { Posterior distribution p(θ; Ájy) / p(Á)p(θjÁ)p(yjθ) = p(θjy; Á)p(Ájy) ¤ often p(θjÁ) is conjugate for p(yjθ) ¤ if we know (or ¯x) Á: p(θjy; Á) follows from conjugacy ¤ then need inference for Á: p(Ájy) 65 Computational approaches for hierarchical models ² Marginal model Z p(yjÁ) = p(yjθ)p(θjÁ)dθ do inference only for Á (e.g. marginal maximum likelihood) ² Empirical Bayes p(θjy; Á^) / p(yjθ)p(θjÁ^) do inference for θ ² Hierarchical Bayes (a.k.a. full Bayes) p(θ; Ájy) / p(yjθ)p(θjÁ)p(Á) inference for θ and Á 66 Hierarchical models and random e®ects Animal breeding example Consider the following mixed linear model commonly used in animal breeding studies Y = X¯ + Zu + e X = design matrix for ¯xed e®ects Z = design matrix for random e®ects ¯ = ¯xed e®ects parameters u = random e®ects parameters 2 e = individual variation » N(0; σe I) 2 2 Y j¯; u; σe » N(X¯ + Zu; σe I) 2 2 ujσa » N(0; σaA) (can also think of ¯ as random with p(¯) / 1) 67 Hierarchical models and random e®ects Animal breeding example ² Marginal model (after integrating out u) 2 2 2 0 2 Y j¯; σa; σe » N(X¯; σaZAZ + σe I) ² Note: the separation of parameters into θ and Á is somewhat ambiguous here: 2 { model speci¯cation suggests Á = fσag and θ = f¯; u; σeg 2 2 { marginal model suggests Á = f¯; σa; σe g and θ = fug 68 Hierarchical models and random e®ects Animal breeding example ² Empirical Bayes (known as REML/BLUP) 2 2 We can estimate σa, σe by marginal 2 2 (restricted?) maximum likelihood (^σa,σ ^e ). Then 2 2 2 2 p(u; ¯jy; σ^a; σ^e ) / p(yj¯; u; σ^e )p(ujσ^a) (a joint normal distn) ² Hierarchical Bayes 2 2 2 2 2 2 p(¯; σa; σe ; ¹jy) / p(yj¯; u; σe )P (ujσa)p(¯; σa; σe ) 69 Computation with hierarchical models ² Two cases { conjugate case (p(θjÁ) conjugate prior for p(yjθ)) ¤ approach described below { non-conjugate case ¤ requires more advanced computing ¤ problem-speci¯c implementations ² Computational strategy for conjugate case { write p(θ; Ájy) = p(Ájy)p(θjÁ; y) { identify conditional posterior density of θ given Á, p(θjÁ; y) (easy for conjugate models) { obtain marginal posterior distribution of Á, p(Ájy) { simulate from p(Ájy) and then p(θjÁ; y) 70 Computation with hierarchical models The marginal posterior distribution p(Ájy) ² Approaches for obtaining p(Ájy) R { integration p(Ájy) = p(θ; Ájy)dθ { algebra - for a convenient value of θ p(θ; Ájy) p(Ájy) = p(θjÁ; y) ² Sampling from p(Ájy) { easy if known distribution { grid if Á is low-dimensional { more sophisticated methods (later) 71 Beta-binomial example ² Series of toxicology studies ² Study j: nj exchangeable individuals yj develop tumors ² Model speci¯cation: { yjjθj » Bin(nj; θj); j = 1;:::;J (indep) { θj; j = 1;:::;J j ®; ¯ » Beta(®; ¯) (iid) { p(®; ¯) { to be speci¯ed later, hopefully "non-informative" ² Marginal model: { can integrate out θj; j = 1;:::;J in this case Z Z J µ ¶ Y ¡(® + ¯) ®¡1 ¯¡1 nj yj n ¡y p(yj®; ¯) = ¢ θ (1 ¡ θ ) θ (1 ¡ θ ) j j dθ ¢ dθ j j j j 1 J j=1 ¡(®)¡(¯) yj J µ ¶ Y nj ¡(® + ¯) ¡(® + yj )¡(¯ + nj ¡ yj ) = j=1 yj ¡(®)¡(¯) ¡(® + ¯ + nj ) { yj; j = 1;:::;J are ind { distn of yj is known as beta-binomial distn 72 Beta-binomial example ² Conditional distn of θ's given ®; ¯; y Q { p(θj®; ¯; y) = j Beta(® + yj; ¯ + nj ¡ yj) { independent conjugate analyses { ¯nd this by algebra or by inspection of p(θ; ®; ¯jy) { analysis is thus reduced to ¯nding (and simulating from) p(®; ¯jy) ² Marginal posterior distn of ®; ¯ YJ ¡(® + ¯) ¡(® + y )¡(¯ + n ¡ y ) p(®; ¯jy) / p(®; ¯) j j j ¡(®)¡(¯) ¡(® + ¯ + n ) j=1 j { could derive from marginal distn on previous slide { could also derive from joint posterior distn { not a known distn (on ®; ¯) but easy to evaluate 73 Beta-binomial example ² Hyperprior distn p(®; ¯) { First try: p(®; ¯) / 1 (flat, noninformative?) ¤ equivalent to p(®=(® + ¯); ® + ¯) / (® + ¯) ¤ equivalent to p(log(®=¯); log(® + ¯)) / ®¯ ¤ check to see if posterior is proper ¢ consider di®'t cases (e.g., ® ! 0; ¯ ¯xed) ¢ if ®; ¯ ! 1 with ®=(® + ¯) = c, then p(®; ¯jy) / constant (not integrable) ¢ this is an improper distn ¢ contour plot would also show this (lots of probability extending out towards in¯nity) 74 Beta-binomial example ² Hyperprior distn p(®; ¯) { Second try: p(®=(® + ¯); ® + ¯) / 1 (flat on prior mean and precision) ¤ more intuitive, these two params are plausibly independent ¤ equivalent to p(®; ¯) / 1=(® + ¯) ¤ still leads to improper posterior distn { Third try: p(log(®=¯); log(® + ¯)) / 1 (flat on natural transformation of prior mean and variance) ¤ equivalent to p(®; ¯) / 1=(®¯) ¤ still leads to improper posterior distn { Fourth try: p(®=(® + ¯); (® + ¯)¡1=2) / 1 (flat on prior s.d. and prior mean) ¤ equivalent to p(®; ¯) / (® + ¯)¡5=2 ¤ "¯nal answer" - proper posterior distn ¤ equivalent to p(log(®=¯); log(® + ¯)) / ®¯(® + ¯)¡5=2 (this will come up later) 75 Beta-binomial example ² Computing { later consider more sophisticated approaches { for now, use grid approach ¤ simulate ®; ¯ from grid approx to posterior distn ¤ then simulate θ's using conjugate beta posterior distn { convenient to use (log(®=¯); log(® + ¯)) scale because contours "look better" and we can get away with smaller grid ² Illustrate with rat tumor data (separate handout) 76 Normal-normal hierarchical model ² Data model 2 { yjjθj » N(θj; σj ); j = 1;:::;J (indep) 2 { σj 's are assumed known (can release this assumption later) { motivation: yj could be a summary statistic with (approx) normal distn from the j-th study (e.g., regression coe±cient, sample mean) ² Prior distn { need a prior distn p(θ1; : : : ; θJ ) { if exchangeable, then model θ's as iid given parameters Á { some additional comments follow 77 Normal-normal hierarchical model ² Constructing a prior distribution Can think of this data model as a one-way ANOVA model (especially if yj is a sample mean of nj obs in group j).
Details
-
File Typepdf
-
Upload Time-
-
Content LanguagesEnglish
-
Upload UserAnonymous/Not logged-in
-
File Pages96 Page
-
File Size-