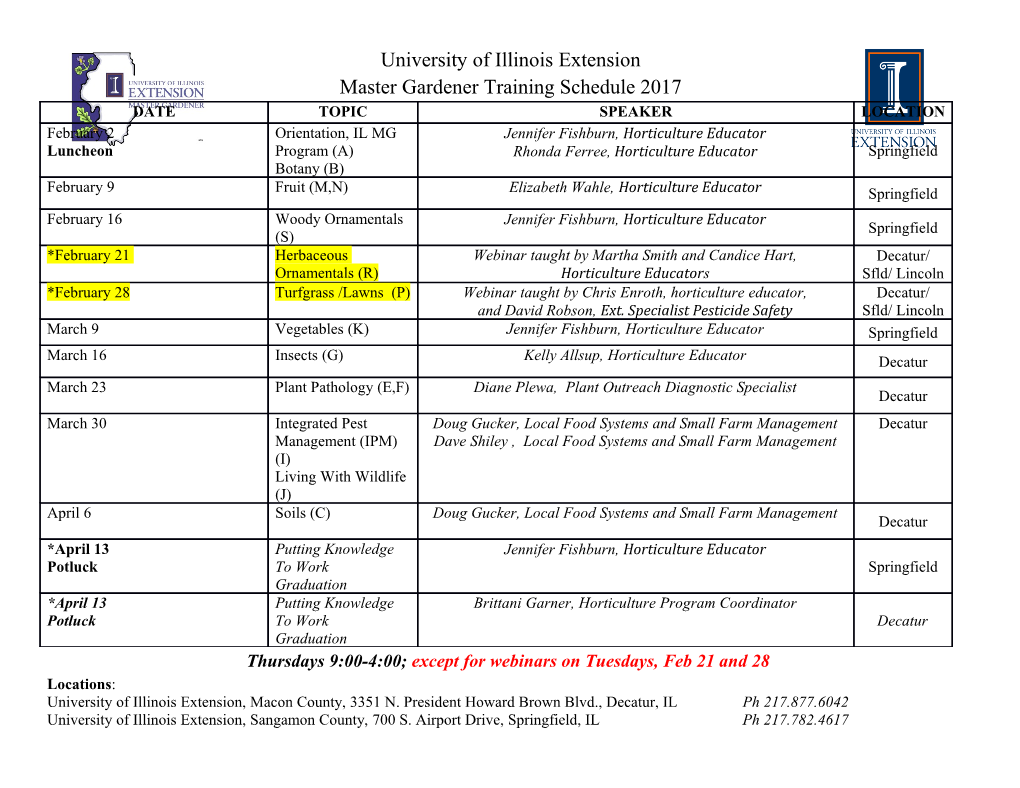
LARGE SCALE CHARGING OF ELECTRIC VEHICLES: TECHNOLOGY AND ECONOMY A Dissertation Presented to the Faculty of the Graduate School of Cornell University in Partial Fulfillment of the Requirements for the Degree of Doctor of Philosophy by Zhe Yu January 2017 © 2017 Zhe Yu ALL RIGHTS RESERVED LARGE SCALE CHARGING OF ELECTRIC VEHICLES: TECHNOLOGY AND ECONOMY Zhe Yu, Ph.D. Cornell University 2017 The electrification of the transportation sector through the diffusion of plug-in electric vehicles (EVs), coupled with cleaner electricity generation, is consid- ered a promising pathway to reduce air pollution from on-road vehicles and to strengthen energy security. The annual new EV sales increased 8-fold since 2011. However, the market share of EVs is still less than 1% in the new ve- hicle market. One of the significant barriers to large-scale adoption of EVs is the charging facilities. Well developed EV charging services are essential for the successful launch of electric vehicles and provide valuable vehicle-to-grid (V2G) services to the smart grid. On the other hand, unplanned charging brings instability, inefficiency and higher cost to the power grid and hinders the rise of clean transportation. This work focuses on the EV charging, including the methodology, develop- ment, and economy. In different contexts, the technologies of EV charging are presented. Both online and off-line approaches are discussed, in decentralized and centralized scenarios. The interaction between the EVs and the power grid is studied, in particular for the V2G services including load shifting, frequency regulation and spinning reserves. The indirect network effect between EVs and EV charging are illustrated. A stylized model for both the EV consumers and the charging facility investor is developed, including the EV price, the coverage of the charging stations, and the price of charging. BIOGRAPHICAL SKETCH Zhe Yu received his B.E. degree from Department of Electrical Engineering, Ts- inghua University, Beijing, China in 2009, and M.S. from Department of Elec- trical and Computer Engineering, Carnegie Mellon University, Pittsburgh, PA, USA in 2010. He is currently working toward the Ph.D. degree in the School of Electrical and Computer Engineering, Cornell University. His current research interests are in smart grid, demand response, dynamic programming and opti- mization. iii This document is dedicated to all Cornell graduate students. iv ACKNOWLEDGEMENTS Firstly, I would like to express my sincere gratitude to my advisor Prof. Tong for the continuous support of my Ph.D study and related research, for his patience, motivation, and immense knowledge. His guidance helped me in all the time of research and writing of this thesis. I could not have imagined having a better advisor and mentor for my Ph.D study. Besides my advisor, I would like to thank the rest of my committee: Prof. Bitar and Prof. Li, for their insightful comments and encouragement, but also for the hard questions which incented me to widen my research from various perspectives. My sincere thanks also goes to Prof. Xu and Jianwei Xing, who provided me the stimulating discussions and precious suggestions on my work. Without their support, it would not be possible to conduct my research. Last but not the least, I would like to thank my family: my parents and my wife for supporting me spiritually throughout writing this thesis and my life in general. v TABLE OF CONTENTS Biographical Sketch . iii Dedication . iv Acknowledgements . .v Table of Contents . vi List of Tables . ix List of Figures . .x 1 Introduction 1 1.1 Electric Vehicle history . .1 1.2 Importance of EV charging. .7 1.3 Overview of technology: challenges and limitations. 10 1.4 Broad classifications of EV charging methodologies . 14 2 EV Charging Models and Energy Management Systems 16 2.1 Charging Standards . 16 2.2 Charging behavior of an EV battery . 18 2.2.1 A Simplified EV Charging model . 22 2.3 Home charging models and impacts on distribution systems. 23 2.3.1 Charging scheduling of single EV . 25 2.4 Large Scale Charging in Distribution System . 27 2.5 Large scale charging at public facilities . 29 2.6 Interaction between EV charging and the grid . 32 3 An online centralized approach of large-scale EV charging 36 3.1 An MDP Formulation of Stochastic Deadline Scheduling . 38 3.1.1 State space . 41 3.1.2 Action . 42 3.1.3 State evolution . 42 3.1.4 Reward . 44 3.1.5 Objective Function . 45 3.1.6 Constrained MDP and Optimal Policies . 45 3.1.7 A Linear Approach . 46 3.1.8 A Performance Upper Bound . 47 3.2 Deadline Scheduling as An RMAB Problem . 48 3.3 Whittle’s Index Policy . 51 3.3.1 Indexability . 51 3.3.2 Whittle’s index policy . 52 3.4 Performance of Whittle’s Index Policy for Finite-Armed Restless Bandits . 54 3.4.1 Performance in the Finite Power limit Cases . 55 3.4.2 An Upper Bound of the Gap-to-Optimality . 57 3.4.3 Least Laxity and Longer Processing Time (LLLP) Principle 57 vi 3.5 Asymptotic performance of the Whittle’s Index Policy . 58 3.6 Performance . 61 3.6.1 Asymptotic optimality . 66 3.7 Literature Review . 66 3.7.1 Worst case analysis . 68 3.7.2 Average case analysis . 71 4 EV charging for vehicle to grid services 73 4.1 Literature Review . 73 4.1.1 EVs as distributed storage and load-shifting agents . 73 4.1.2 EVs as ancillary service providers . 74 4.2 Load Shifting . 75 4.3 Frequency Regulation . 77 4.3.1 Large Scale EV Charging with Ancillary Service . 78 4.3.2 Ancillary Service Bidding . 80 4.3.3 Real-time Scheduling . 82 4.3.4 Participation of EVs in the Frequency Regulation Market . 85 5 Market Dynamics and Indirect Network Effects in Electric Vehicle D- iffusion 91 5.1 Literature Review . 94 5.2 A Sequential Game Model . 97 5.2.1 Two-sided market structure . 98 5.2.2 The investor’s decision model . 99 5.2.3 The consumer’s decision model . 101 5.2.4 The sequential game model . 104 5.3 Consumer Decisions . 105 5.3.1 Consumer Decision Model and Assumptions . 105 5.3.2 Consumer Decisions and EV Market Share . 106 5.4 Investor Decisions . 110 5.4.1 Investor Decision Model and Assumptions . 110 5.4.2 Optimal Charging Price . 111 5.4.3 Optimal Charging Station Locations . 114 5.4.4 Social welfare optimization . 116 5.5 Discussions . 119 5.5.1 Effects of subsidy . 119 5.5.2 Socially optimal solution vs. private market solution . 121 5.5.3 Other policies . 124 5.5.4 Applications . 126 A Appendices for Chapter 3 128 A.1 Proof of Theorem 1 . 128 A.1.1 Indexability of dummy arms . 128 A.1.2 Indexability of regular arms . 128 vii A.2 Proof of Theorem 2 . 133 A.2.1 When T = 2 ........................... 134 A.2.2 When T > 2 ........................... 135 A.3 Proof of Lemma 1 . 137 A.3.1 Proof of Lemma 2 . 141 A.3.2 Proof of Lemma 3 . 142 A.4 Proof of Theorem 3 . 145 A.5 Proof of Theorem 4 . 145 B Appendices for Chapter 4 147 B.1 Proof of Theorem 8 . 147 B.2 Proof of Theorem 9 . 149 B.3 Proof of Theorem 10 . 151 Bibliography 154 viii LIST OF TABLES 2.1 EV charging classification . 16 ix LIST OF FIGURES 1.1 First Electric horseless Carriage, by Sibrandus Stratingh. It was simply a wooden platform carrying a battery and a motor. Now it is on display at the Museum Boerhaave in Netherlands. .1 1.2 Queen Alexandra’s electric car for driving around the grounds of Sandringham House. .3 1.3 Tesla model S: powered by a battery pack with capacity 85 kWh, driving range 265 miles, 0 to 60 mph in 2.5 seconds. .5 1.4 Nissan leaf: the most popular EV model by 2016. Powered by a Li-ion battery pack with capacity 24 kWh, driving range 107 miles.6 2.1 Level 1 charger . 17 2.2 Level 2 charger . 19 2.3 DC charger . 20 2.4 Discharge curve . 20 2.5 EV attributes. 23 2.6 Architecture of home energy management system . 24 2.7 Architecture of home energy management system . 29 2.8 Architecture for network switched charging and iEMS. 31 2.9 Load curve of New York ISO, November 8th, 2016 . 33 2.10 Solar power output in a typical day.can not find usable plot or data 34 2.11 Charging station participating demand response. 35 3.1 Architecture of a charging station . 38 3.2 An illustration for the charger’s state. ri is the arrival time of an EV at charger i, di the deadline for completion, ji[t] the amount of charging to be completed by di, Ti[t] the lead time to deadline. 41 3.3 An illustration for the charging cost evolution. Given the current state ck, the next state will be ck0 with probability Pk;k0 ........ 43 3.4 An illustration for the charger state evolution given the charger is activated. T¯ is the maximum lead time and ¯j the maximum charging demand. 44 3.5 Performance comparison: constant charging cost c[t] = 0:5, Q(0; 0) = 0:3, T¯ = 12, ¯j = 9, β = 0:999, F( j) = 0:2 j2, N = 10...... 62 3.6 Performance comparison: constant charging cost c[t] = 0:5, Q(0; 0) = 0:3, T¯ = 12, ¯j = 9, β = 0:999, F( j) = 0:2 j2, M=N = 0:5...
Details
-
File Typepdf
-
Upload Time-
-
Content LanguagesEnglish
-
Upload UserAnonymous/Not logged-in
-
File Pages180 Page
-
File Size-