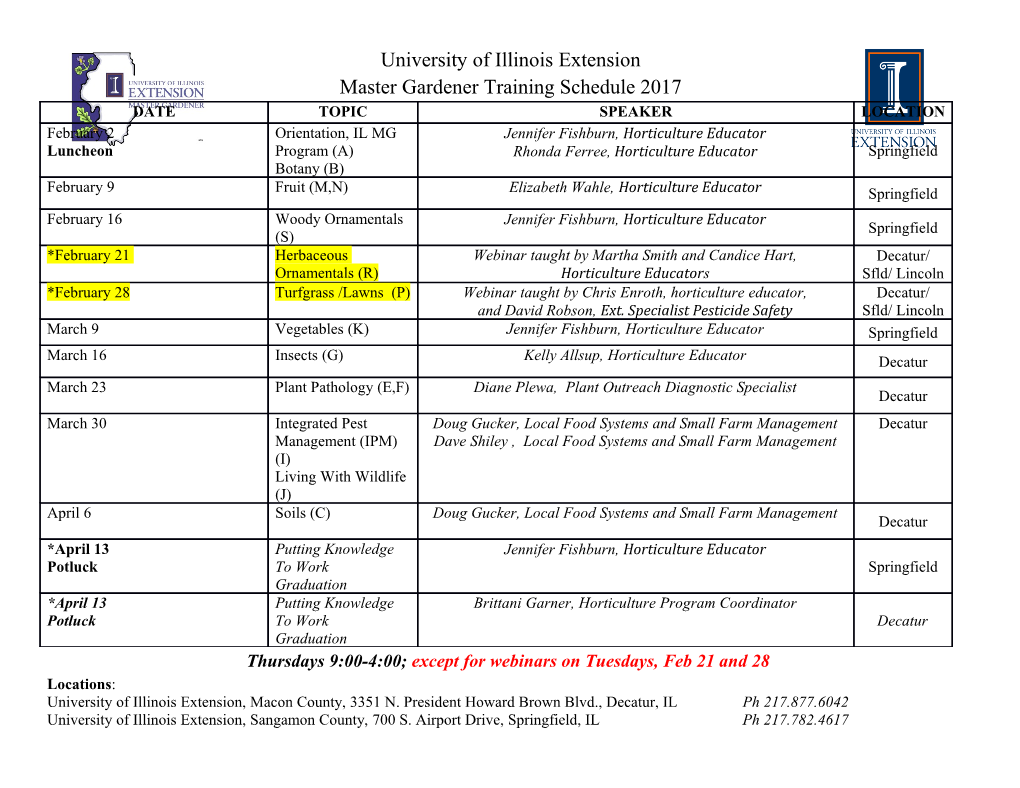
Combined Neyman–Pearson Chi-square: An Improved Approximation to the Poisson-likelihood Chi-square Xiangpan Ji∗, Wenqiang Gu, Xin Qian, Hanyu Wei, Chao Zhang∗∗ Physics Department, Brookhaven National Laboratory, Upton, NY, USA Abstract We describe an approximation to the widely-used Poisson-likelihood chi-square using a linear combination of Neyman’s and Pearson’s chi-squares, namely “combined Neyman–Pearson chi- 2 2 square” (χCNP). Through analytical derivations and toy model simulations, we show that χCNP leads to a significantly smaller bias on the best-fit model parameters compared to those using either Neyman’s or Pearson’s chi-square. When the computational cost of using the Poisson-likelihood 2 chi-square is high, χCNP provides a good alternative given its natural connection to the covariance matrix formalism. Keywords: test statistics, Poisson-likelihood chi-square, Neyman’s chi-square, Pearson’s chi-square 1. Introduction In high-energy physics experiments, it is often convenient to bin the data into a histogram with n bins. The number of measured events Mi in each bin typically follows a Poisson distribution with the mean value µi¹θº predicted by a set of model parameters θ = ¹θ1; :::; θN º. The likelihood function of this Poisson histogram can be written as: n − M Ö e µi µ i L¹µ¹θº; Mº = i : (1) M ! i i A maximum-likelihood estimator (MLE) of θ can be constructed by maximizing the likelihood ratio [1, 2] L¹µ¹θº; Mº L¹µ¹θº; Mº λ¹θº = 0 = ; (2) max L¹µ ; Mº L¹M; Mº where the denominator is a model-independent constant that maximizes the likelihood of the data without any restriction on the model1. Maximizing this likelihood ratio is equivalent to minimizing arXiv:1903.07185v3 [physics.data-an] 25 Feb 2020 ∗Corresponding author. Email: [email protected] ∗∗Corresponding author. Email: [email protected] 1While the estimation of model parameters θ does not depend on the denominator of the likelihood ratio, the chi- square test statistic constructed in this way, such as that in Eq. (3), can be used to examine the data-model compatibility with a goodness-of-fit test. Preprint submitted to Nuclear Instruments and Methods A February 26, 2020 the Poisson-likelihood chi-square function [3, 4]: n Õ Mi χ2 = −2 ln λ¹θº = 2 µ ¹θº − M + M ln : (3) Poisson i i i µ ¹θº i=1 i The MLE is commonly used in the high-energy physics, as it is generally an asymptotically unbiased estimator, and has the advantage of being consistent and efficient [5]. At large statistics, the previous Poisson distribution can be approximated by a normal (or 2 Gaussian) distribution with mean µi¹θº and variance σi = µi¹θº. The likelihood then becomes: 2 Ö 1 ¹µi¹θº − Miº L ¹µ¹θº; Mº = exp − : (4) Gauss p 2µ ¹θº i 2πµi¹θº i The Gauss-MLE can be similarly constructed through a likelihood ratio: LGauss¹µ¹θº; Mº λGauss¹θº = 0 ; (5) max LGauss¹µ ; Mº where the denominator is the maximum of LGauss without any restriction on the model, and can 0 be derived by calculating @LGauss/@µi = 0. Maximizing λGauss¹θº is equivalent to minimizing the Gauss-likelihood chi-square function 2 0 2 ! Õ ¹µ ¹θº − M º µ ¹θº ¹µ − Miº χ2 = −2 ln λ ¹θº = i i + ln i − i ; Gauss Gauss µ ¹θº µ0 µ0 i=1 i i i (6) 0 q 2 with µi = 1/4 + Mi − 1/2 : While the Gauss-likelihood chi-square is relatively well-known (see e.g. [6, 7]) 2, interestingly, it is not widely used in high-energy physics experiments. Instead, a direct chi-square test statistic, namely the Pearson’s chi-square, is constructed through: 2 Õ ¹µi¹θº − Miº χ2 = : (7) Pearson µ ¹θº i i 2 2 Comparing with Eq. (6), we see χPearson consists of only the first term in χGauss. These two chi-squares become asymptotically equivalent when Mi is large. 2 In practice, the variance σi is often approximated by the measured value Mi, which is indepen- dent of the model parameters. This leads to another popular chi-square test statistic in high-energy physics experiments, namely the Neyman’s chi-square: 2 Õ ¹µi¹θº − Miº χ2 = : (8) Neyman M i i 2We further provide some relevant formulas for the Gauss-likelihood chi-square in Appendix D. 2 Comparing to the MLE from the Poisson-likelihood chi-square, it is known that the estimator of model parameters constructed from Pearson’s or Neyman’s chi-square leads to biases especially 2 when the large-statistics condition is not met [4, 8, 9]. Despite this shortcoming, both χPearson and 2 χNeyman are commonly used in physics data analysis, partly because of their close connection to the covariance-matrix formalism: 2 T −1 χcov = ¹M − µ¹θºº · V · ¹M − µ¹θºº; (9) where Vij = cov»µi; µj¼ is the covariance matrix of the prediction, and can often be calculated through Monte Carlo methods based on the statistical and systematic uncertainties of the experiment 2 prior to the minimization of χcov. In situations where many nuisance parameters [5] are required in the likelihood function L as in Eq. (1), the covariance matrix format Eq. (9) has a natural advantage of reducing the number of nuisance parameters, thus leads to a faster minimization of the χ2 function. 2 One method to remove the bias of the estimator from χPearson is through an iteration of the 2 weighted least-squares fit, where the variance in one round of χPearson minimization is replaced by the prediction from the best-fit value in the previous round of iteration [10, 11, 12]. Several modified chi-square test statistics have also been proposed in past literatures to mitigate the bias 2 2 issue. For example, χGauss defined in Eq. (6) is a good replacement of χPearson when the number of 2 2 measurements is large. Similarly, χγ as proposed by Mighell [13] is a good alternative to χNeyman 2 2 when the number of measurements is large. Both χGauss and χγ , however, still lead to biases when the number of measurements is small. Redin proposed a solution by including a cubic term in 2 2 2 χNeyman and χPearson [14], or by reporting a weighted average of fitting results from χNeyman and 2 χPearson [15]. In this paper, we propose a new method through the construction of a chi-square test statistic 2 (χCNP) with a linear combination of Neyman’s and Pearson’s chi-squares. As an improved approximation to the Poisson-likelihood chi-square with respect to either Neyman’s or Pearson’s 2 chi-square, the χCNP significantly reduces the bias while keeping the advantage of the covariance 2 matrix formalism. This paper is organized as follows. The construction of χCNP and its covariance matrix format is described in Sec. 2. Three toy examples are presented in Sec. 3 to illustrate the 2 features and advantages of χCNP. Finally, we summarize the recommended usage in data analysis of counting experiments in Sec. 4. 2 2. Combined Neyman–Pearson Chi-square (χCNP) The bias in the estimator of model parameters θ using Neyman’s or Pearson’s chi-square can be traced back to the different χ2 definitions in approximating the Poisson-likelihood chi-square. To illustrate this, we start with a simple example. A set of n independent counting experiments were performed to measure a common expected value µ. Each experiment measured Mi events. 3 The three chi-square functions in this case are 3: n Õ M χ2 = 2 µ − M + M ln i ; Poisson i i µ i=1 n 2 Õ ¹µ − Miº χ2 = ; (10) Neyman M i i n Õ ¹µ − M º2 χ2 = i : Pearson µ i µ^ (the estimator of µ) can be calculated through the minimization of Eq. (10): @ χ2/@µ = 0. We obtain: s Ín Ín 2 Mi n i=1 M µ^ = i=1 ; µ^ = ; µ^ = i : (11) Poisson n Neyman Ín 1 Pearson n i=1 Mi Given Eq. (11), it is straightforward to show that µ^Neyman ≤ µ^Poisson ≤ µ^Pearson, where the equal sign is only established when all values of Mi are the same. Since µ^Poisson is unbiased in this simple example, we see that µ^Pearson and µ^Neyman are biased in the opposite directions. We further examine the difference in chi-square values. Assuming that Mi and µ are reasonably 2 large so that Mi is close to µ, a Taylor expansion of χPoisson yields: n Õ µ − M χ2 = 2 µ − M − M ln 1 + i Poisson i i M i=1 i n " # (12) Õ ¹µ − M º2 2 ¹µ − M º3 ¹µ − M º4 ≈ i − i + O¹ i º : M 3 2 3 i=1 i Mi Mi From Eq. (12), it is straightforward to deduce: n Õ 2 ¹µ − M º3 χ2 − χ2 ≈ − i ; Poisson Neyman 3 2 i Mi n (13) Õ 1 ¹µ − M º3 χ2 − χ2 ≈ i : Poisson Pearson 3 2 i Mi Naturally, we can define a new chi-square function as a linear combination of Neyman’s and Pearson’s chi-squares: n 2 1 Õ ¹µ − Miº χ2 ≡ χ2 + 2χ2 = ; (14) CNP 3 Neyman Pearson 3/( 1 + 2 º i=1 Mi µ 3 The treatment for bins where Mi = 0 is described in Appendix A. 4 4 2 ¹µ−Miº 2 2 which is approximately equal to χPoisson up to O¹ 3 º, better than either χNeyman or χPearson Mi 2 alone. In this example, the estimator µ^ from minimizing χCNP can be derived as: v tÍn M2 q µ^ = 3 i=1 i = 3 µ^2 · µ^ ; CNP Ín 1 Pearson Neyman (15) i=1 Mi which is the geometric mean of two µ^Pearson and one µ^Neyman.
Details
-
File Typepdf
-
Upload Time-
-
Content LanguagesEnglish
-
Upload UserAnonymous/Not logged-in
-
File Pages21 Page
-
File Size-