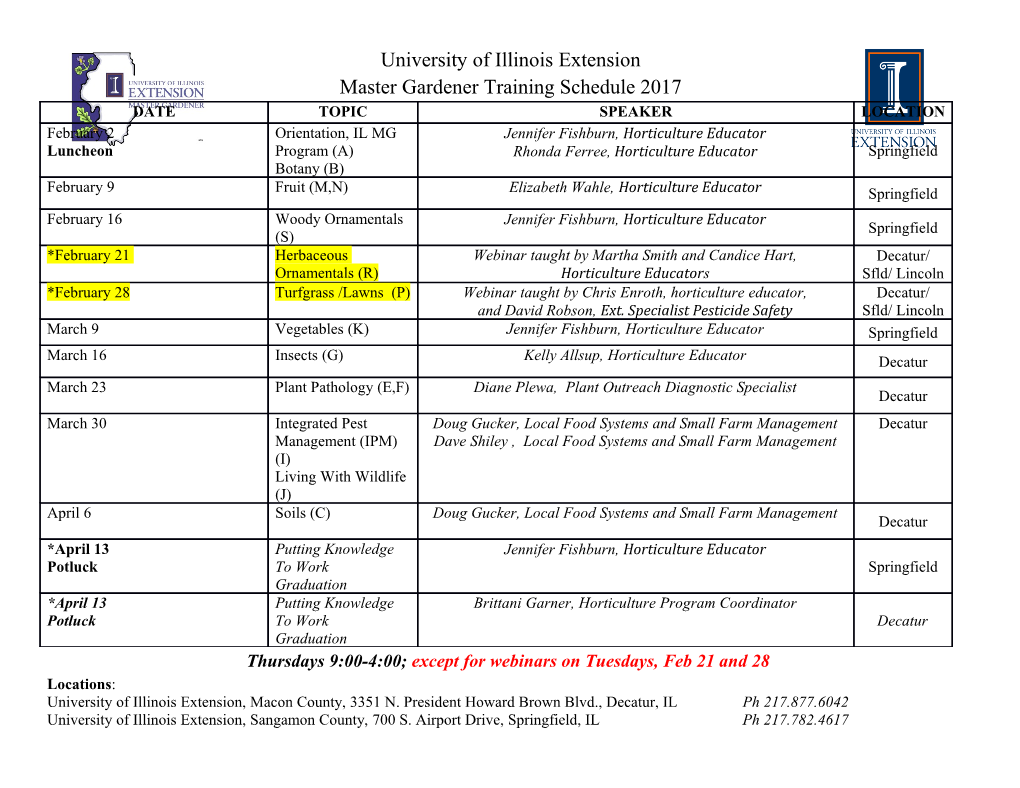
16A.4 POLARIMETRIC CLASSIFICATION OF WINTER WEATHER PRECIPITATION TYPE USING THERMODYNAMIC INPUT FROM NUMERICAL MODELS Terry J. Schuur, Alexander V. Ryzhkov, and Heather D. Reeves Cooperative Institute for Mesoscale Meteorological Studies, University of Oklahoma and NOAA/OAR/National Severe Storms Laboratory 1. INTRODUCTION related to the generation of supercooled liquid water (Hogan et al. 2002), 4) regions The classification of cold-season of high ZDR and KDP that are believed to be precipitation type at the surface is associated with dendritic growth and/or ice complicated by the broad range of crystal generation (Moisseev et al. 2008; precipitation types that might result from Andric et al. 2009; Kennedy and Rutledge processes that occur below the height of the 2011), and 5) an apparent tendency for ZDR radar’s lowest elevation sweep. For in the snow region to increase upon the example, a deep layer of subfreezing air onset of storm decay as regions of dry, near the surface might lead to either a aggregated snow (characterized by low ZDR) complete refreezing of drops (ice pellets) or are replaced by pristine ice crystals refreezing upon contact with the surface (characterized by high ZDR). (freezing rain). Both of these precipitation In this study, we present results from the types are difficult to determine using radar continued evolution of a polarimetric data alone. Existing classification schemes, classification algorithm that is designed to such as the polarimetric Hydrometeor take advantage of thermodynamic output Classification Algorithm (HCA, Park et al. from a numerical model in the classification 2009) currently being deployed on the WSR- process. An earlier version of the algorithm 88D network, also do not take full advantage described by Schuur et al. (2011) has been of observations made at several elevation modified to use output from the High- angles. This effectively eliminates the Resolution Rapid-Refresh (HRRR) model. possibility of observations made aloft to be In addition to providing more information to used in the classification process. An aid in the interpretation of polarimetric example of such signatures is summarized signatures, the thermodynamic information by Ryzhkov et al. (2011), who report on provides a mechanism to produce surface- recent observational work to identify based classification results at distant ranges repetitive polarimetric signatures associated from the radar, where low-level layers of with microphysical processes in winter warm/cold air that fall well below the lowest storms. Analyses of numerous case studies available radar data might result in revealed signatures such as 1) a low-level microphysical processes that would enhancement in ZDR that appears to be otherwise remain undiagnosed. The benefit related to the refreezing of melted or of adding the thermodynamic data is partially melted hydrometeors, 2) downward therefore twofold: 1) to enhance excursions of the bright band to the surface classification capabilities in regions where resulting in localized regions of heavy, wet polarimetric radar data are available, and 2) snow, 3) plumes of high ZDR associated with to extend classification capabilities to embedded updrafts and possibly also regions where it is not. The project also seeks to provide an algorithm framework Corresponding author address: Terry J. Schuur that allows ongoing observational work, as University of Oklahoma/CIMMS listed above, to be easily incorporated into National Weather Center future algorithm development with the long 120 David L. Boren Blvd., Norman, OK, 73072 term goals of improving automated E-mail: [email protected] precipitation type classification at both the surface and aloft, including the capability to across the model grid using T, Td, and p. If remotely diagnose conditions favorable for the surface wet-bulb temperature TWs > 3°C, liquid water generation. it is assumed that precipitation at the surface is rain. However, if TWs < 3°C, the vertical 2. ALGORITHM DEVELOPMENT profile of TW at that point is classified as belonging to one of the four different types The classification algorithm used in this shown in Fig. 1. H0, H1, and H2 in Fig. 1 paper is similar to that first reported on by depict the heights of the 0°C crossing points Schuur et al. (2011), except that it has been in the profiles. Making use of the studies by modified to use higher resolution model data Czys et al. (1996), Zerr (1997), and Rauber that is mapped to a radar-centric coordinate et al. (2001), the TW profiles are then used to system, thereby providing better resolution create a background classification that and improved diagnostic capabilities. The consists of six precipitation categories: 1) initial classification is performed using output snow (SN), 2) wet snow (WS), 3) freezing from the High-Resolution Rapid-Refresh rain (FR), 4) ice pellets (IP), 5) a (HRRR) model analyses, which are created combination of freezing rain and ice pellets by interpolating the 13-km grid-spaced (FR/IP), and 6) rain (RA). In this procedure, Rapid-Refresh analyses to a 3.1-km spacing the threshold for the maximum and minimum using a 16-point bi-linear interpolation acceptable TW profiles in the warm (TWmax) method. The analyses are produced every and cold (TWmin) layers, respectively, are hour by assimilating observed variables into derived from a visual inspection of the the 1-hr forecast from the previous cycle scatterplots presented by Figs. 5 and 6 of using a variational three-dimensional Zerr (1997). Following the flow chart analysis scheme. Vertical profiles of the presented in Fig. 2: wet-bulb temperature TW are calculated (a) (b) Type 1 Type 2 H H H0 TWs 0 T 0 TWs3 T (c) (d) H Type 3 H Type 4 H0 H0 TWmax H1 TWmax H1 TWmin H2 TWmin 0 0 TWs T TWs T Fig. 1. Four types of vertical profiles of wet-bulb temperature (Tw) corresponding to four or more types of precipitation. BEGIN Yes No Yes Tw profile TWs > 3°C ? SN Type 1? RA No T > 2°C T < 2°C T No T No Wmax No Wmax W W and and profile profile T > -5°C? T < -5°C? Type 2? Type 3? Wmin Wmin Yes Yes Yes Yes No 0°C < TWmax < 2°C H0 < 1 km? and TWmin < -5°C? FR IP FR/IP Yes No Yes No WS RA IP RA Fig. 2. Flow chart showing logistic for determination of precipitation types depending on vertical profile of wet bulb temperature. • When TWs > 3°C, the precipitation at the • For profile Type 4 (Fig. 1d), where TWs < surface is classified as RA. 0°C and the TW profile crosses the 0°C level two times, the precipitation at the • For profile Type 1 (Fig. 1a) where TW < surface is classified as FR if TWmax > 2°C 0°C throughout the entire depth of the and TWmin > -5°C and IP if TWmax < 2°C profile, the surface precipitation is and TWmin < -5°C. Otherwise the classified as SN. precipitation at the surface is classified as FR/IP. • For profile Type 2 (Fig. 1b), where 0° < TWs < 3°C and the TW profile crosses the Polarimetric radar data are then used to fine 0°C level one time, the precipitation at tune the initial classification by determining the surface is classified as WS if H0 < 1 whether or not it is consistent with the radar km. Otherwise, the precipitation at the observations. For example, a polarimetric surface is classified as RA. radar observation of a bright band would be inconsistent with a model-based surface • For profile Type 3 (Fig. 1c), where 0° < classification of dry snow. Radar data are TWs < 3°C and the TW profile crosses the also used to refine a precipitation type within 0°C level three times, the precipitation at a category, such as by using Z and ZDR the surface is classified as IP if 0°C < observations to discern between ice crystals TWmax < 2°C and TWmin < -5°C, where and dry snow. The algorithm outputs 9 TWmax is the maximum TW in the vertical classes of hydrometeors: crystals (CR), dry profile and TWmin is the minimum TW in snow (DS), wet snow (WS), ice pellets/sleet the vertical profile. Otherwise the (IP), freezing rain (FR), a mix of freezing rain precipitation is classified as RA. and ice pellets (FR/IP), rain (RA), heavy rain (HR), and hail (HA). 3. CASE STUDY EXAMPLES (FR/IP and IP categories, see Fig. 3e) also both indicate that this broad region had In this section, we examine algorithm conditions favorable towards the generation output for 3 winter storm events sampled by of ice pellets and/or a ice pellet/freezing rain the OU-PRIME radar on December 24, mix. It should also be noted that the 2009, January 28, 2010, and February 1, algorithm output also indicates that the 2011. In total, 31 volumes of radar data region of ice pellets had a “branch” that (corresponding to 31 hourly HRRR grids) extended well to the west of the OKC metro were processed for these 3 events. area. The radar modification of the Reconstructed RHIs of polarimetric variables background classification, as shown by Fig. with HRRR TW fields overlaid were then 3f, indicates that small pockets of low ρHV at produced for every 5° of azimuth. Here we the western periphery of this ice pellet show classification results and reconstructed region were reclassified to be wet snow. RHIs for each of the 3 winter events to Future work will need to include more illustrate current algorithm analysis and focused efforts to collect information on classification capabilities. In particular, we precipitation type in an attempt to better focus on some of the precipitation features validate these types of features in the that are summarized by Ryzhkov et al.
Details
-
File Typepdf
-
Upload Time-
-
Content LanguagesEnglish
-
Upload UserAnonymous/Not logged-in
-
File Pages13 Page
-
File Size-