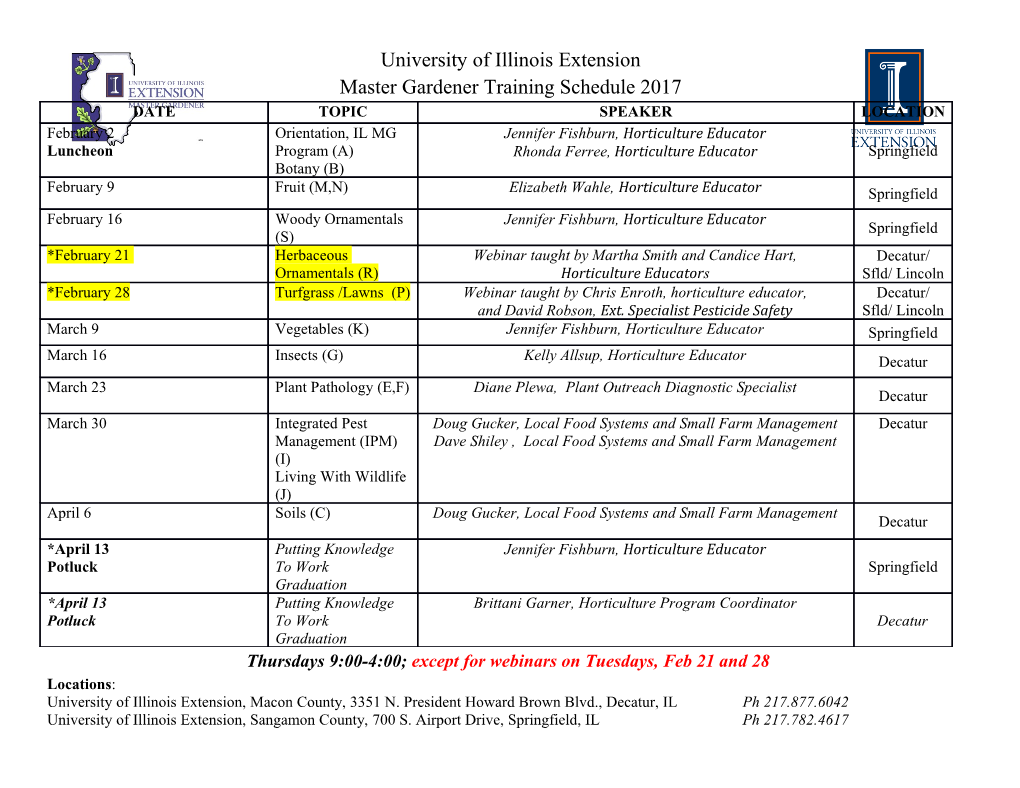
Chapter 2 Linear Spaces Contents FieldofScalars ........................................ ............. 2.2 VectorSpaces ........................................ .............. 2.3 Subspaces .......................................... .............. 2.5 Sumofsubsets........................................ .............. 2.5 Linearcombinations..................................... .............. 2.6 Linearindependence................................... ................ 2.7 BasisandDimension ..................................... ............. 2.7 Convexity ............................................ ............ 2.8 Normedlinearspaces ................................... ............... 2.9 The `p and Lp spaces ............................................. 2.10 Topologicalconcepts ................................... ............... 2.12 Opensets ............................................ ............ 2.13 Closedsets........................................... ............. 2.14 Boundedsets......................................... .............. 2.15 Convergence of sequences . ................... 2.16 Series .............................................. ............ 2.17 Cauchysequences .................................... ................ 2.18 Banachspaces....................................... ............... 2.19 Completesubsets ....................................... ............. 2.19 Transformations ...................................... ............... 2.21 Lineartransformations.................................. ................ 2.21 Continuity............................................. ........... 2.22 Compactness......................................... .............. 2.23 Upper semicontinuous functions . ................. 2.26 QuotientSpaces ....................................... .............. 2.27 Denseness .......................................... .............. 2.27 Separability ........................................... ............ 2.27 Schauderbasis....................................... ............... 2.27 Summary ............................................ ............ 2.28 2.1 2.2 c J. Fessler, October 4, 2004, 12:44 (student version) In systems analysis, linear spaces are ubiquitous. Why? Linear systems/models are easier to analyze; many systems, particularly in signal processing, are deliberately designed to be linear; linear models are a useful starting point (approximation) for more complicated nonlinear cases. Formal definitions of a vector space use the concept of a field of scalars, so we first review that. Field of Scalars (from Applied Linear Algebra, Noble and Daniel, 2nd ed.) A field of scalars is a collection of elements α,β,γ,... along with an “addition” and a “multiplication” operator. F To every pair of scalars α, β in , there must correspond a scalar α + β in , called the sum of α and β, such that Addition is commutative: α F+ β = β + α F • Addition is associative: α + (β + γ) = (α + β) + γ • There exists a unique element 0 , called zero, for which α + 0 = α, α • For every α , there corresponds∈F a unique scalar ( α) for which∀α +∈F ( α) = 0. • ∈F − ∈F − To every pair of scalars α, β in , there must correspond a scalar αβ in , called the product of α and β, such that Multiplication is commutative:F αβ = βα F • Multiplication is associative: α(βγ) = (αβ)γ • Multiplication distributes over addition: α(β + γ) = αβ + αγ • There exists a unique element 1 , called one, or unity, or the identity element, for which 1α = α, α • ∈F 1 ∀ ∈F1 For every nonzero α , there corresponds a unique scalar α− , called the inverse of α for which αα− = 1. • ∈F ∈F Simple facts for fields: 0+0=0 (use α = 0 in the definition of 0) • 0 = 0 Proof. For any α, by the associative property (α + 0) + ( 0) = α + (0 + ( 0)) hence α + ( 0) = α. Hence, since •the − zero element is unique, 0 = 0. − − − − Example. The set of rational numbers Q (with the usual definition of addition and multiplication) is a field. The only fields that we will need are the field of real numbers R and the field of complex numbers C. Therefore, hereafter we will use when describing results that hold for either R or C. F c J. Fessler, October 4, 2004, 12:44 (student version) 2.3 Vector Spaces In simple words, a vector space is a space that is closed under vector addition and under scalar multiplication. Definition. A vector space or linear space consists of the following four entities. 1. A field of scalars. F 2. A set of elements called vectors. X 3. An operation called vector addition that associates a sum x + y with each pair of vectors x, y such that Addition is commutative: x + y = y + x ∈X ∈X • Addition is associative: x + (y + z) = (x + y) + z • There exists an element 0 , called the zero vector, for which x + 0 = x, x • For every x , there corresponds∈X a unique vector ( x) for which x +∀ ( x∈X) = 0. • ∈X − ∈X − 4. An operation called multiplication by a scalar that associates with each scalar α and vector x a vector αx , called the product of α and x, such that: ∈F ∈X ∈X Associative: α(βx) = (αβ)x • Distributive α(x + y) = αx + αy • Distributive (α + β)x = αx + βx • If 1 is the identify element of , then 1x = x. x . • 0x = 0 for any x . F ∀ ∈X • ∈X The requirement that x + y and αx is sometimes called the closure property. ∈X ∈X Simple facts for vector spaces: 0 is unique. • ( 1)x = x for x . • Proof.− x +− ( 1)x =∈X 1x + ( 1)x = (1 + ( 1))x = 0x = 0. α0 = 0 for α− . − − • Proof. α0 = α∈F0 + 0 = α0 + [α0 + ( α0)] = [α0 + α0] + ( α0) = α(0 + 0) + ( α0) = α0 + ( α0) = 0. x + y = x + z implies y = z (cancellation− law) − − − • αx = αy and α = 0 implies x = y (cancellation law) • αx = βx and x 6= 0 implies α = β (cancellation law) • α(x y) = αx 6 αy (distributive law) • (α −β)x = αx − βx (distributive law) • α−x = α( x) =− (αx) • − − − 2.4 c J. Fessler, October 4, 2004, 12:44 (student version) What are some examples? (Linear algebra classes focus on finite-dimensional examples.) Important Vector Spaces Euclidean space or n-tuple space: = Rn. If x , then x = (a , a ,...,a ) where a R and we use ordinary addition • X ∈X 1 2 n i ∈ and multiplication: x + y = (a1 + b1, a2 + b2,...,an + bn) and αx = (αa1,...,αan). (As a special case, the set of real numbers R (with ordinary addition and multiplication) is a trivial vector space.) 2 2 = 1 R . The set of functions f : R R that are absolutely (Lebesgue) integrable: ∞ ∞ f(x,y) dx dy < , •with X theL usual pointwise definition of addition→ and scalar multiplication. −∞ −∞ | | ∞ 2 2 R R To show show that f, g 1 R implies f + g 1 R , one can apply the triangle inequality: f + g f + g . The set of functions on the∈ L plane R2 that are zero∈ outside L of the unit square. | | ≤ | | | | • The set of solutions to a homogeneous linear system of equati ons Ax = 0. • C[a, b]: the space of real-valued, continuous functions defined on the interval [a, b]. • The space of band-limited signals. • Many more in Luenberger... • Example. For 1 p < , define the following infinite-dimensional1 space: ≤ ∞ 1 = R [a, b] = f : [a, b] R : f is Riemann integrable and f(t) p dt < , X p → | | ∞ Z0 (with the usual pointwise definitions of addition of functions and multiplication of functions by a scalar). To show that this space is a vector space, the only nontrivial work is verifying closure. Clearly if f then αf since 1 αf(t) p dt = α p 1 f(t) p dt < , so is closed under scalar multiplication. ∈X ∈X 0 | | | | 0 | | ∞ X To show that f +g if f, g , i.e.R , closure under addition,R requires a bit more work. Note that since a+b 2 max a , b , it follows for p 1∈Xthat ∈X | | ≤ {| | | |} ≥ a + b p 2p max a p, b p 2p[ a p + b p]. | | ≤ {| | | | } ≤ | | | | Hence if f, g : ∈X 1 1 1 1 f(t) + g(t) p dt [2p f(t) p + 2p f(t) p] dt 2p f(t) p dt +2p f(t) p dt < + = . | | ≤ | | | | ≤ | | | | ∞ ∞ ∞ Z0 Z0 Z0 Z0 showing closure under addition. Example. Can = (0, ) with = R be a vector space? ?? X ∞ F Cartesian product We can make a “larger” vector space from two vector spaces and (having a common field ) by forming the Cartesian product of and , denoted , which is the collection ofX orderedY pairs (x, y) where x andF y . X Y X ×Y ∈X ∈Y = (x, y) : x and y . X ×Y { ∈X ∈ Y} To be a vector space we must define vector addition and scalar multiplication operations, which we define component-wise: (x1, y1) + (x2, y2) = (x1 + x2, y1 + y2) • α(x, y) = (αx, αy), α . • ∀ ∈F Fact. With these definitions the Cartesian product of two vector spaces is indeed a vector space. The above definition generalizes easily to higher-order combinations. Example. R3 = R R R. × × 1A definition of dimension is forthcoming... c J. Fessler, October 4, 2004, 12:44 (student version) 2.5 2.3 Subspaces A (nonempty) subset S of a vector space is called a subspace of if S, when endowed with the addition and scalar multiplication operations defined for , is a vector space,X i.e., αx + βy S wheneverX x, y S and α, β . X ∈ ∈ ∈F Example. The subset of = R2[ 1, 1] consisting of symmetric functions (f( t) = f(t)) is a subspace of . It is clearly closed under addition and scalarX multiplication.− − X What are the four types of subspaces of R3? ?? Intuition: think of a subspace like a line or plane (or hyperplane) through the origin. Properties of subspaces 0 S • 0∈ is a subspace of • { }is a subspace of X •A X subspace not equalX to the entire space is called a proper subspace • If M and N are subspaces of a vector spaceX , then the intersection M N is also a subspace of . Proof. see text • Think: intersection of planes (through the origin)X in 3d. ∩ X Typically the union of two subspaces is not a subspace. • Think: union of planes (through the origin) in 3d. Although unions usually fail, we can combine two subspaces by an appropriate sum, defined next. Sum of subsets Definition. If S and T are two subsets of a vector space, then the sum of those subsets, denoted S + T is defined by S + T = x = s + t : s S, t T . { ∈ ∈ } Example. What is the sum of a plane and a line (both through origin) in R3? ?? Example. Consider = R2, with S = (x, 0) : x [0, 1] and T = (0,y) : y [0, 1] . Then S + T is the unit square. X { ∈ } { ∈ } Proposition. If M and N are subspaces of a vector space , then the sum M + N is a subspace of .
Details
-
File Typepdf
-
Upload Time-
-
Content LanguagesEnglish
-
Upload UserAnonymous/Not logged-in
-
File Pages29 Page
-
File Size-