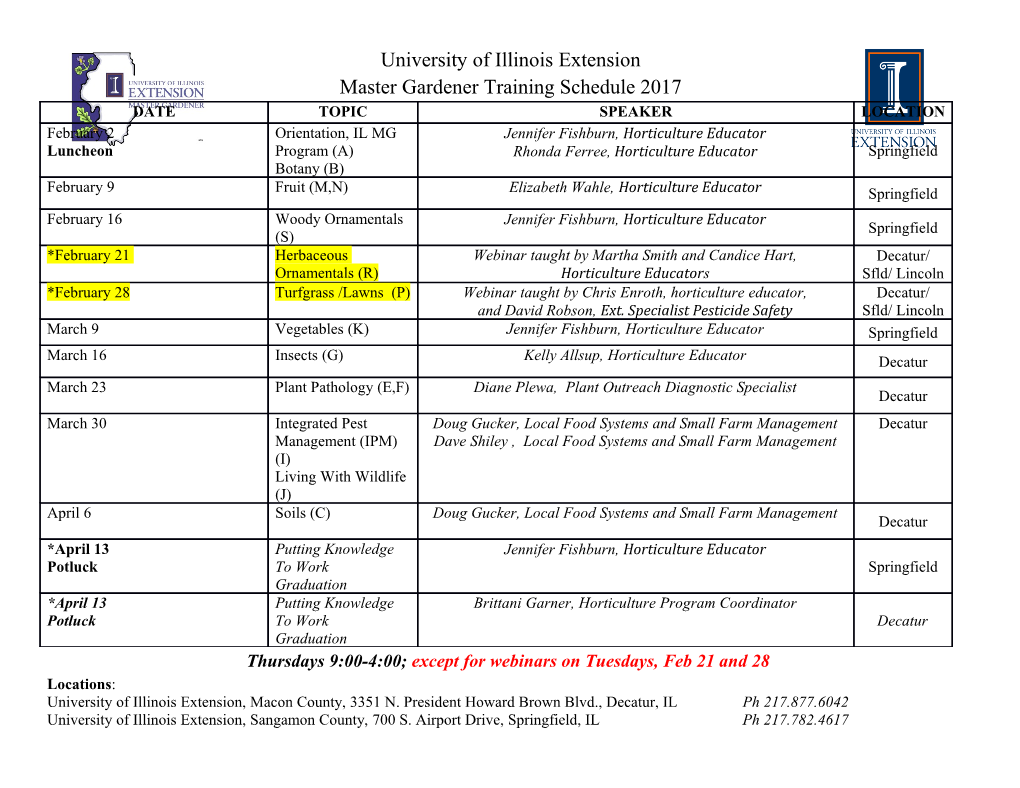
City University of New York (CUNY) CUNY Academic Works All Dissertations, Theses, and Capstone Projects Dissertations, Theses, and Capstone Projects 2-2019 A Defense of Pure Connectionism Alex B. Kiefer The Graduate Center, City University of New York How does access to this work benefit ou?y Let us know! More information about this work at: https://academicworks.cuny.edu/gc_etds/3036 Discover additional works at: https://academicworks.cuny.edu This work is made publicly available by the City University of New York (CUNY). Contact: [email protected] A DEFENSE OF PURE CONNECTIONISM by ALEXANDER B. KIEFER A dissertation submitted to the Graduate Faculty in Philosophy in partial fulfillment of the requirements for the degree of Doctor of Philosophy, The City University of New York 2019 © 2019 ALEXANDER B. KIEFER All Rights Reserved ii A Defense of Pure Connectionism By Alexander B. Kiefer This manuscript has been read and accepted by the Graduate Faculty in Philosophy in satisfaction of the dissertation requirement for the degree of Doctor of Philosophy. _________________________ _________________________________________ 1/22/2019 Eric Mandelbaum Chair of Examining Committee _________________________ _________________________________________ 1/22/2019 Nickolas Pappas Executive Officer Supervisory Committee: David Rosenthal Jakob Hohwy Eric Mandelbaum THE CITY UNIVERSITY OF NEW YORK iii ABSTRACT A Defense of Pure Connectionism by Alexander B. Kiefer Advisor: David M. Rosenthal Connectionism is an approach to neural-networks-based cognitive modeling that encompasses the recent deep learning movement in artificial intelligence. It came of age in the 1980s, with its roots in cybernetics and earlier attempts to model the brain as a system of simple parallel processors. Connectionist models center on statistical inference within neural networks with empirically learnable parameters, which can be represented as graphical models. More recent approaches focus on learning and inference within hierarchical generative models. Contra influential and ongoing critiques, I argue in this dissertation that the connectionist approach to cognitive science possesses in principle (and, as is becoming increasingly clear, in practice) the resources to model even the most rich and distinctly human cognitive capacities, such as abstract, conceptual thought and natural language comprehension and production. Consonant with much previous philosophical work on connectionism, I argue that a core principle—that proximal representations in a vector space have similar semantic values—is the key to a successful connectionist account of the systematicity and productivity of thought, language, and other core cognitive phenomena. My work here differs from preceding work in philosophy in several respects: (1) I compare a wide variety of connectionist responses to the systematicity challenge and isolate two main strands that are both historically important and reflected in ongoing work today: (a) vector symbolic architectures and (b) (compositional) vector space semantic models; (2) I consider very recent applications of these approaches, including iv their deployment on large-scale machine learning tasks such as machine translation; (3) I argue, again on the basis mostly of recent developments, for a continuity in representation and processing across natural language, image processing and other domains; (4) I explicitly link broad, abstract features of connectionist representation to recent proposals in cognitive science similar in spirit, such as hierarchical Bayesian and free energy minimization approaches, and offer a single rebuttal of criticisms of these related paradigms; (5) I critique recent alternative proposals that argue for a hybrid Classical (i.e. serial symbolic)/statistical model of mind; (6) I argue that defending the most plausible form of a connectionist cognitive architecture requires rethinking certain distinctions that have figured prominently in the history of the philosophy of mind and language, such as that between word-, phrase-, and sentence-level semantic content, and between inference and association. v Acknowledgements This dissertation is dedicated to my daughter Evelyn Stelmak Kiefer, who was born just before it was. I’m not sure what she would need it for, but it’s hers. Thanks to my partner Natallia Stelmak Schabner for her patience, love and inspiration during the writing of this work, and to her daughter Sophia. I am deeply grateful to my family (all of you, truly—I won’t name names here in case there’s some cousin I forgot about) for the same, and for believing in my potential since probably before I was born in many cases. The list of friends that I have to thank for accompanying me through life and sustaining my very existence is long, but I wish to thank here those who have specifically encouraged and inspired my work in philosophy over the years: Andrew, Ian, Rose, Rachel, Richard, Hakwan, and Pete. I am also grateful to the chiptune crew and other friends in music and the arts (particularly Jeff, Kira, Haeyoung, Jamie, Chris, Tamara, Josh, Dok, Amy, Pietro, and Jeremy) for being the truest of friends and for informing my work in oblique but essential ways. If I’ve forgotten anyone, get in touch and I’ll put you in the dedication when I write a book. Thanks to my advisor David Rosenthal and the members of my dissertation committee, Jakob Hohwy, Eric Mandelbaum, Richard Brown, and Gary Ostertag, for helpful feedback on drafts of this work. I’d additionally like to thank my advisor for introducing me to cognitive science, for expert and dedicated guidance over the years, for serving as an exemplar and inspiration, and for his patience in helping me work through many iterations of these ideas (and many others). I would like to thank Jakob Hohwy for taking an early interest in my work, for encouraging me to develop its most promising aspects, and for generously sharing his insight and enthusiasm. Thanks to Richard Brown and Gary Ostertag for their support over the years, and for being involved with this project since the prospectus days. And additional thanks to Eric Mandelbaum for posing the stiffest challenges to my work in the sincere hope of improving it. vi I am also grateful to teachers, friends and fellow graduate students, including Pete Mandik, Josh Weisberg, Kenneth Williford, Hakwan Lau, Graham Priest, Janet Fodor, David Pereplyotchik, Jake Quilty-Dunn, Zoe Jenkin, Grace Helton, Jona Vance, Ryan DeChant, Jesse Atencio, Kate Pendoley, Jacob Berger, Henry Shevlin, Kate Storrs, Eric Leonardis, Hudson Cooper, and the cognitive science group at the CUNY Graduate Center, for indispensable exchanges that have informed my thinking. Thanks also to Geoffrey Hinton for kindly replying to my two brash and overly familiar emails. It was more inspiring than you know. And not least to Jerry Fodor, for his love of the philosophy of mind and for goading the connectionists on back when their success seemed less than certain. vii A Defense of Pure Connectionism - Contents Chapter 1: Preliminaries on Connectionism and Systematicity Introduction………………………………………………………………………………………1 1.1 Systematicity, productivity and compositionality……………………………………….. 10 1.2 Fodor and Pylyshyn’s challenge to connectionism…………………………………….. 14 1.3 Systematicity and vector spaces………………………………………………………… 19 Chapter 2: Vector Symbolic Architectures 2.1 Smolensky architectures………………………………………………………………….. 26 2.2 Smolensky’s take on Smolensky architectures………………………………………… 32 2.3 Fodor’s take on Smolensky architectures………………………………………………. 36 2.4 McLaughlin’s take on Smolensky architectures……………………………………….. 42 2.5 Holographic Reduced Representations…………………………………………………. 48 2.6 Processing in Vector Symbolic Architectures………………………………………….. 52 2.7 Compositional VS combinatorial systems………………………………………………. 56 2.8 Inferential systematicity…………………………………………………………………… 62 2.9 Some problems for VSAs……………………………………………………………….... 67 Chapter 3: Neural Vector Space Models 3.1 Distributional semantics………………………………………………………………….. 76 3.2 Vector space models in semantics………………………………………………………. 81 3.3 Neural language models and vector space embeddings……………………………… 85 3.4 GloVe vectors……………………………………………………………………………….92 viii 3.5 Collobert et al’s model: syntax from distribution………………………………………. 97 3.6 Compositionality in vector space semantic models…………………………………….104 Chapter 4: Parsing in Connectionist Networks 4.1 The RAAM system and autoencoders………………………………………………….. 110 4.2 Discovering parse trees……………………………………………………………………117 4.3 More powerful tree-shaped models……………………………………………………… 122 4.4 Applying computer vision techniques to language…………………………………….. 129 4.5 Hinton’s critique of CNNs…………………………………………………………………. 134 4.6 The capsules approach…………………………………………………………………… 139 Chapter 5: Concepts and Translation 5.1 Concepts and propositions……………………………………………………………….. 148 5.2 Words as regions, concepts as operators………………………………………………. 155 5.3 The prototype theory of concepts revisited…………………………………………….. 162 5.4 Machine translation and the language of thought……………………………………... 168 5.5 Multimodal connectionist nets……………………………………………………………. 175 5.6 Larger-scale multimodal models…………………………………………………………. 182 Chapter 6: Reasoning 6.1 Inference, association and vector spaces……………………………………………… 189 6.2 Induction and semantic entailment………………………………………………………. 197 6.3 Inductive reasoning in connectionist systems………………………………………….. 204 6.4 Deductive reasoning in connectionist systems………………………………………… 211 6.5 The PLoT
Details
-
File Typepdf
-
Upload Time-
-
Content LanguagesEnglish
-
Upload UserAnonymous/Not logged-in
-
File Pages271 Page
-
File Size-