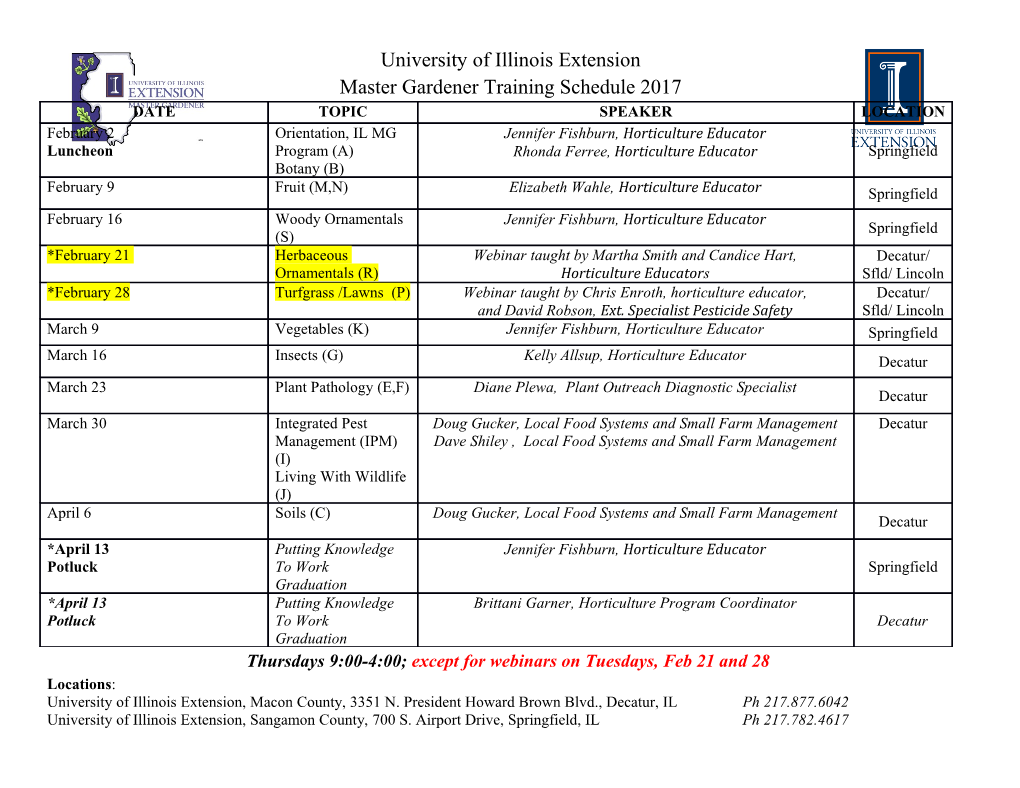
Overview of Research at IITB Computational studies on Hindustani music CompMusic Workshop, Chennai 2013 Preeti Rao Department of Electrical Engineering I.I.T. Bombay 1 Some goals • Automatic “tagging” of audio by genre, style, raga, tala and other discovered descriptors and relationships • Automatic creation of “navigation layer” for concert audio • Facilitating search for musically relevant objects such as melodic and rhythmic motifs • Building tools that facilitate musicological research on performance practices Common to all the above: Need for a music representation (aka features) and similarity measure (for classification) 2 Music CD cover information… (YouTube has even less!) 3 Hindustani music descriptors/tags • Artiste (instrument), accompanists • Genre (dhrupad, khyal, tarana…), sub-genre (gharana) • Concert structure and sections with timing – Bada khyal, Chhota khyal • Bandish: alap, vistaar, taan • Raga, Tala, Laya of major sections • Composition (bandish, identified by mukhda) The question Can the descriptions be obtained by audio content analysis and possibly enhanced with contextual semantic information? 4 Kishori Amonkar Deshkar: bada khyal (vistaar, taan) Alap (slow tempo) Alap (medium tempo) Taan 5 Kishori Amonkar Deshkar: bada khyal taan, chhota khyal taan Taan (madhylaya) Taan (drut laya) 6 Kishori Amonkar Deshkar vistaar alap taan chhota khyal (bada khyal) (bada khyal) 7 Uday Bhawalkar (dhrupad) Yaman Alap Jod (alap) Jhala (alap) 8 Uday Bhawalkar (dhrupad) Yaman alap jod jhala 9 Kishori Amonkar: Deshkar, Gaud-Sarang Raga Deshkar Raga Gaud Sarang 10 original resynthesized Raga Deshkar Raga Gaud Sarang 11 Raga Deshkar P P P P G G G G G G R S R R S R S S R S S D R S Raga Gaud Sarang R G S S S S S S S R D D S R D D D 12 Raga characteristics from pitch distribution Pt. Vidhyadhar Vyas Marwa and Puriya (share the same swaras) 13 Melodic motif (mukhda) detection Kishori Amonkar, Deshkar, Tintal Bandish: Piya Jaag 14 Phrase duration, dependence on tempo 15 Within-concert variability of motif 16 Within-concert variability across concerts Intra-phrase class distance distribution Kafi 17 Ontology for Indian music Learning metadata (textual) from forums by using NLP techniques to learn relationships between entities. • Augment audio-based music ontology for Indian music with information extracted from online music forums to achieve superior retrieval systems for Indian music. Example: Get songs with phrase ‘NDNP’ and sung by a disciple of D.K. Pattammal • Challenges: text sources are unstructured, ungrammatical… 18 Summary • High-level musical attributes (melody, rhythm) can be derived from low-level acoustic parameters such as pitch and onsets extracted by audio signal processing. • The audio signal processing is challenging due to the mixture of several instruments, strong diversity in the characteristics and the highly time-varying nature. • Melody and rhythm representations that are musicologically informed can be useful in the description of music recordings. • Knowledge can be very helpful. Creating these from available sources is a challenge. 19 Coming up… • Vedhas Pandit, Kaustuv : Characterization of melodic motifs • Vinutha T. P.: Rhythmic structure based segmentation • Joe Cheri Ross: Ontology for Indian Music: An Approach for ontology learning from online music forums • Amruta J. Vidwans and Prateek Verma: Melodic style detection in Hindustani music 20 Thank you Department of Electrical Engineering , IIT Bombay 21 .
Details
-
File Typepdf
-
Upload Time-
-
Content LanguagesEnglish
-
Upload UserAnonymous/Not logged-in
-
File Pages21 Page
-
File Size-