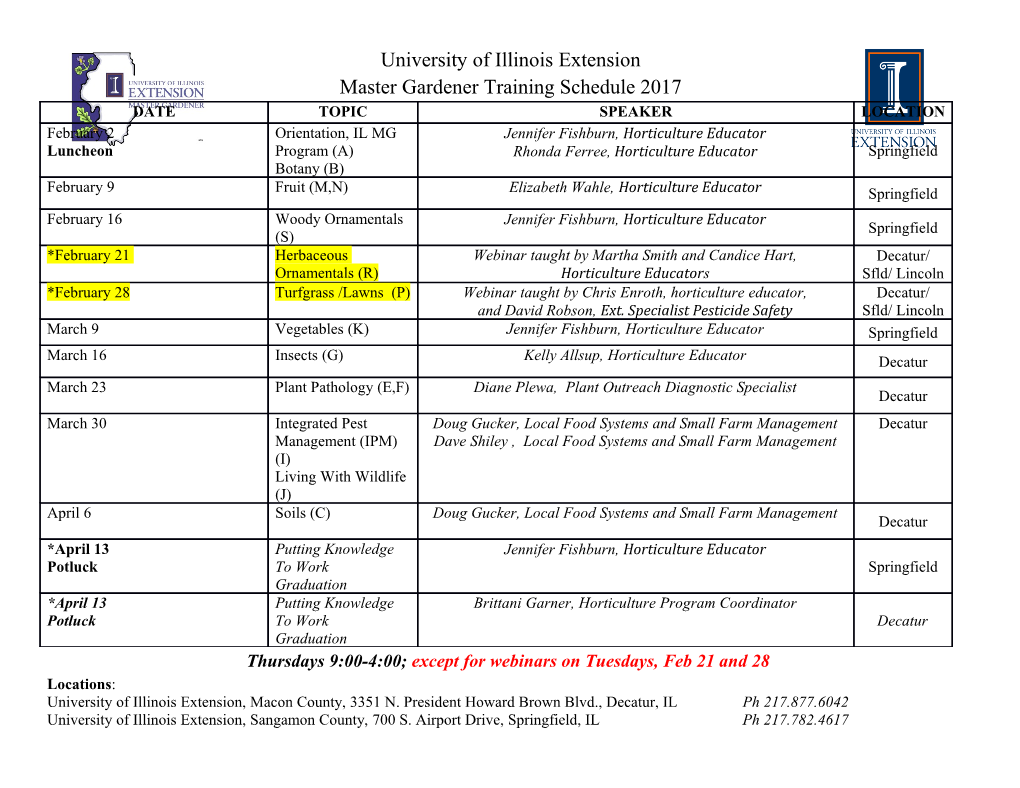
La Habana, November 2001 ' $ Bayesian and Conditional Frequentist Hypothesis Testing and Model Selection James O. Berger Duke University, USA VIII C.L.A.P.E.M. La Habana, Cuba, November 2001 & %1 La Habana, November 2001 ' $ Overall Outline Basics ² Motivation for the Bayesian approach to model ² selection and hypothesis testing Conditional frequentist testing ² Methodologies for objective Bayesian model selection ² Testing when there is no alternative hypothesis ² & %2 La Habana, November 2001 ' $ Basics Brief History of Bayesian statistics ² Basic notions of Bayesian hypothesis testing (through ² an example) Di±culties in interpretation of p-values ² Notation and example for model selection ² & %3 La Habana, November 2001 ' $ Brief History of (Bayesian) Statistics 1760 { 1920 : Statistical Inference was primarily Bayesian Bayes (1764) : Binomial distribution, ¼(θ) = 1. Laplace (. , 1812) : Many distributions, ¼(θ) = 1. Edgeworth . Pearson . & %4 La Habana, November 2001 ' $ A curiosity - the name: 1764 { 1838: called \probability theory" 1838 { 1945: called \inverse probability" (named by Augustus de Morgan 1945 { : called \Bayesian analysis" 1929 { 1955 : Fisherian and Frequentist approaches developed and became dominant, because now one could do practical statistics \without the logical flaws resulting from always using ¼(θ) = 1." & %5 La Habana, November 2001 ' $ Voices in the wilderness: Harold Je®reys: ¯xed the logical flaw in inverse probability (objective Bayesian analysis) Bruno de Finetti and others: developed the logically sound subjective Bayes school. 1955 { : Reemergence of Bayesian analysis, and development of Bayesian testing and model selection. & %6 La Habana, November 2001 ' Psychokinesis Example $ The experiment: Schmidt, Jahn and Radin (1987) used electronic and quantum-mechanical random event generators with visual feedback; the subject with alleged psychokinetic ability tries to “influence” the generator. { Stream of particles arrive at a `quantum gate'; each goes on to either a red or a green light { Quantum mechanics implies particles are 50/50 to go to each light { Individual tries to “influence” particles to go to red light & %7 La Habana, November 2001 ' $ Data and model: Each \particle" is a Bernoulli trial (red = 1, green = 0) ² θ = probability of \1" n = 104; 900; 000 trials X = # \successes" (# of 1's), X Binomial(n; θ) » x = 52; 263; 000 is the actual observation 1 To test H0 : θ = 2 (subject has no influence) versus H : θ = 1 (subject has influence) 1 6 2 P-value = Pθ= 1 (X x) :0003. ² 2 ¸ ¼ Is there strong evidence against H0 (i.e., strong evidence that the subject influences the particles) ? & %8 La Habana, November 2001 ' $ Bayesian Analysis: (Je®erys, 1990) Prior distribution: P r(Hi) = prior probability that Hi is true, i = 0; 1; On H : θ = 1 , let ¼(θ) be the prior density for θ. 1 6 2 Subjective Bayes: choose the P r(Hi) and ¼(θ) based on personal beliefs Objective (or default) Bayes: choose 1 P r(H0) = P r(H1) = 2 ¼(θ) = 1 (on 0 < θ < 1) & %9 La Habana, November 2001 ' $ Posterior distribution: P r(H x) = probability that H true, given data, x 0j 0 f(x θ= 1 ) P r(H ) j 2 0 = P r(H ) f(x θ= 1 )+P r(H ) f(x θ)¼(θ)dθ 0 j 2 1 j For the objective prior, R P r(H x = 52; 263; 000) 0:94 0j ¼ (recall, p-value .0003) ¼ Key ingredients for Bayesian Inference: { the model for the data { the prior ¼(θ) { ability to compute or approximate integrals & %10 La Habana, November 2001 ' $ Bayes Factor: 1 An `objective' alternative to choosing P r(H0) = P r(H1) = 2 is to report the Bayes factor likelihood of observed data under H0 B01 = 0 `average likelihood of observed data under H1 f(x θ= 1 ) = j 2 15:4 1 f(x θ)¼(θ)dθ ¼ 0 j R P r(H0 x) P r(H0) Note: j = B01 P r(H1 x) P r(H1) £ (posterior jodds) (prior odds) (Bayes factor) so B01 is often thought of as \the odds of H0 to H1 provided by the data" & %11 La Habana, November 2001 'Bayesian Reporting in Hypothesis Testing $ The complete posterior distribution is given by ² { P r(H x), the posterior probability of null hypothesis 0j { ¼(θ x; H ), the posterior distribution of θ under H j 1 1 A useful summary of the complete posterior is ² { P r(H x) 0j { C, a (say) 95% posterior credible set for θ under H1 In the psychokinesis example ² { P r(H x) = :94 ; gives the probability of H 0j 0 { C = (:50008; :50027) ; shows where θ is if H1 is true For testing precise hypotheses, con¯dence intervals ² alone are not a satisfactory inferential summary & %12 La Habana, November 2001 ' $ Crucial point: In this example, θ = :5 (or θ :5) is ¼ plausible. If θ = :5 has no special plausibility, a di®erent analysis will be called for. Example: Quality control for truck transmissions { θ = % of transmissions that last at least 250,000 miles { the manufacturer wants to report that θ is at least 1=2 { test H : θ 0:5 vs H : θ < 0:5 0 ¸ 1 Here θ = :5 has no special plausibility. Note: whether one does a one-sided or two-sided test is not very important. What is important is whether or not a point null has special plausibility. & %13 La Habana, November 2001 ' $ Clash between p-values and Bayesian answers In the example, the p-value :0003, but the posterior ¼ probability of the null 0:94 (equivalently, the Bayes ¼ factors gives 15.4 odds in favor of the null). Could this ¼ conflict be because of the prior distribution used? But it was a neutral, objective prior. ² Any sensible prior produces Bayes factors orders of ² magnitude larger than the p-value. For instance, any symmetric (around :5), unimodal prior would produce a Bayes factor at least 30 times larger than the p-value. & %14 La Habana, November 2001 ' $ P-values also fail frequentist evaluations An Example Experimental drugs D ; D ; D ; : : : ; are to be tested ² 1 2 3 (same illness or di®erent illnesses; independent tests) For each drug, test ² H1 : Di has negligible e®ect vs H2 : Di is e®ective Observe (independent) data for each test, and compute ² each p-value (the probability of observing hypothetical data as or more \extreme" than the actual data). & %15 La Habana, November 2001 ' $ Results of the Tests of the Drugs: ² TREATMENT D1 D2 D3 D4 D5 D6 P-VALUE 0.41 0.04 0.32 0.94 0.01 0.28 TRATAMIENTO D7 D8 D9 D10 D11 D12 P-VALOR 0.11 0.05 0.65 0.009 0.09 0.66 Question: How strongly do we believe that D has a ² i non negligible e®ect when: (i) the p-value is approximately :05? (ii) the p-value is approximately :01? & %16 La Habana, November 2001 ' $ A surprising fact: Suppose it is known that, a priori, about 50% of the Di will have negligible e®ect. Then (i) of the D for which p-value 0.05, at least 25% i ¼ (and typically over 50%) will have negligible e®ect; (ii) of the D for which p-value 0.01, at least 7% i ¼ (and typically over 15%) will have negligible e®ect; (Berger and Sellke, 1987, Berger and Delampady, 1987) & %17 La Habana, November 2001 ' $ An interesting simulation for normal data: Generate random data from H , and see where the ² 0 p-values fall. Generate random data from H , and see where the ² 1 p-values fall. Under H0, such random p-values have a uniform distribution on (0; 1). & %18 La Habana, November 2001 ' $ Under H1, \random data" might arise from either: (i) Picking a θ = 0 and generating the data 6 (ii) Picking some sequence of θ's and generating a corresponding sequence of data (i) Picking a distribution ¼(θ) for θ; generating θ's from ¼; and then generating data from these θ's Example: Picking ¼(θ) to be N(0; 2) and n = 20, yields the following fraction of p-values in each interval & %19 La Habana, November 2001 ' $ 0.10 0.05 0.0 1.0 0.9 0.8 0.7 0.6 0.5 0.4 0.3 0.2 0.1 P-value 0.02 0.01 0.0 0.10 0.09 0.08 0.07 0.06 0.05 0.04 0.03 0.02 0.01 P-value 0.015 0.001 0.0 0.010 0.009 0.008 0.007 0.006 0.005 0.004 0.003 0.002 0.001 P-value & %20 La Habana, November 2001 'Surprise : No matter how one generates \random" $ p-values under H1, at most 3.4% will fall in the interval (:04; :05), so a p-value near :05 (i.e., t near 1.96) j j provides at most 3.4 to 1 odds in favor of H1 (Berger and Sellke, J. Amer.Stat. Assoc., 1987) Message : Knowing that data is \rare" under H0 is of little use unless ones determines whether or not it is also \rare" under H1. In practice : For moderate or large sample sizes, data under H1, for which the p-value is between 0:04 and 0:05, is typically as rare or more rare than data under H0, so that odds of about 1 to 1 are then reasonable. & %21 La Habana, November 2001 ' $ The previous simulation can be performed on the web, using an applet available at: http://www.stat.duke.edu/ berger » & %22 La Habana, November 2001 'Notation for general model selection $ Models (or hypotheses) for data x: M1; M2; : : : ; Mq Under model Mi : Density of X: f (x θ ), θ unknown parameters i j i i Prior density of θi: ¼(θi) 1 Prior probability of model Mi: P (Mi), ( = q here) Marginal density of X: m (x) = f (x θ )¼ (θ ) dθ i i j i i i i Posterior density: ¼(θ x) = f (x θ )¼ (θ )=m (x) ij i Rj i i i i Bayes factor of Mj to Mi: Bji = mj(x)=mi(x) Posterior probability of Mi: 1 P (Mi)mi(x) q P (Mj ) ¡ P (Mi x) = q = Bji P (M )m (x) j=1 P (Mi) j j=1 j j hP i P & %23 La Habana, November 2001 ' $ Particular case : P (Mj) = 1=q : mi(x) 1 P (Mi x) = m¹ i(x) = q = q j j=1 mj(x) j=1 Bji P P Reporting : It is useful to separately report m¹ (x) and f i g P (M ) .
Details
-
File Typepdf
-
Upload Time-
-
Content LanguagesEnglish
-
Upload UserAnonymous/Not logged-in
-
File Pages126 Page
-
File Size-