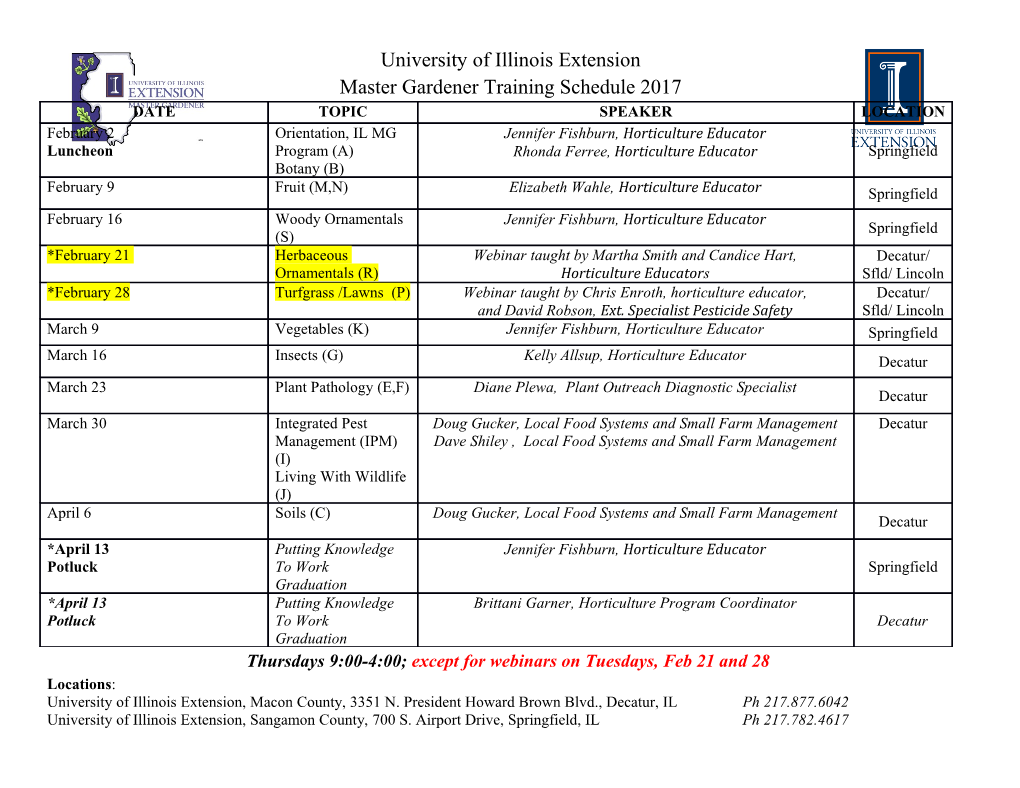
Cell Prolif., 2011, 44, 291–299 doi: 10.1111/j.1365-2184.2011.00752.x Cyclin-dependent kinase inhibitor 3 (CDKN3) novel cell cycle computational network between human non-malignancy associated hepatitis⁄cirrhosis and hepatocellular carcinoma (HCC) transformation L. Wang*, L. Sun*, J. Huang* and M. Jiang† *Biomedical Center, School of Electronic Engineering, Beijing University of Posts and Telecommunications, Beijing, China, and †Laboratory of Computational Linguistics, School of Humanities and Social Sciences, Tsinghua University, Beijing, China Received 19 September 2010; revision accepted 3 January 2011 Abstract mitotic G2 checkpoint in non-malignancy associated The relationship of cyclin-dependent kinase inhibi- hepatitis ⁄cirrhosis; (iii) with stronger cell cycle tor 3 (CDKN3) with tumours has previously been and weaker cytokinesis, as a result forming multi- presented in a number of publications. However, the nucleate cells in HCC. Thus, it is useful to identify molecular network and interpretation of CDKN3 CDKN3 cell cycle networks for comprehension through the cell cycle between non-malignancy of molecular mechanism between non-malignancy associated hepatitis⁄cirrhosis and hepatocellular associated hepatitis⁄ cirrhosis and HCC transfor- carcinoma (HCC) have remained to be elucidated. mation. Here, we have constructed and analysed significant high expression gene CDKN3 activated and inhib- Introduction ited cell cycle networks from 25 HCC versus 25 non-malignancy associated hepatitis⁄cirrhosis Cyclin-dependent kinase inhibitor 3 (CDKN3) is one of patients (viral infection HCV or HBV) in GEO Data- the genes our group has identified as highly expressed in set GSE10140-10141, by combination of a gene 25 HCC versus 25 non-malignancy associated hepati- tis ⁄ cirrhosis cases. We data-mined CDKN3 roles in cell regulatory network inference method based on linear components, molecular functions, biological processes, programming, and decomposition procedure using KEGG, GenMAPP, BioCarta and disease, from Capital- CapitalBio MAS 3.0 software, based on integration Bio MAS 3.0 software (CapitalBio Corporation, Beijing, of public databases including Gene Ontology, China). CDKN3 cell component is localized in perinuclear KEGG, BioCarta, GenMapp, Intact, UniGene, regions, molecular function is comprised of protein serine/ OMIM, and others. Comparing the same and differ- threonine phosphatase activity, protein tyrosine phospha- ently activated and inhibited CDKN3 networks with tase activity, protein binding, protein tyrosine • serine • GO analysis, between non-malignancy associated threonine phosphatase activity and hydrolase activity, bio- hepatitis ⁄cirrhosis and HCC, our results suggest a logical process includes regulation of cyclin-dependent CDKN3 cell cycle network (i) with stronger DNA protein kinase activity, G1 ⁄ S transition after mitosis in the replication and with weaker ubiquitin-dependent cell cycle, cell cycle in general, cell cycle arrest, negative protein catabolism as common characteristics in regulation of cell proliferation, dephosphorylation (GO (http: ⁄⁄www.geneontology.org)). CDKN3 is relative to both non-malignancy associated hepatitis⁄cirrhosis hs_1-tissue-endocrine_and_cns, regulation of protein and HCC; (ii) with more cell division and weaker kinase activity, regulation of transferase activity, protein amino acid dephosphorylation, negative regulation of cell proliferation and regulation of cell proliferation (Gen- Correspondence: L. Wang, Biomedical Center, School of Electronics MAPP (http: ⁄⁄www.genmapp.org/)). Studies into CDKN3 Engineering, Beijing University of Posts and Telecommunications, Beijing 100876, China. Tel.: 8610 86885531; Fax: 8610 62785736; relationships in tumours has already been presented in a E-mail: [email protected] number of papers (1–13). However, distinct molecular All authors contributed equally to this study. networks and interpretation concerning CDKN3 in the cell Ó 2011 Blackwell Publishing Ltd. 291 292 L. Wang et al. cycle during non-malignancy associated hepatitis ⁄ cirrho- acc.cgi?acc=GSE10141) (15) and we pre-processed raw sis and hepatocellular carcinoma (HCC) transformation microarray data as log 2. have remained to be elucidated. HCC is one of the most common causes of cancer- Gene selection algorithms related death the world over. Thus, to develop novel drugs for treatment of HCC has become a challenge for biolo- Potential HCC molecular markers were identified using gists. Here, we have constructed and analysed signifi- significant analysis of microarrays (SAM), which is a cantly higher expression of the gene CDKN3 activated statistical technique for finding significant genes in a set and inhibited in cell cycle networks from 25 HCC versus of microarray experiments. Input to SAM comprised 25 non-malignancy associated hepatitis ⁄ cirrhosis patients gene expression measurements from a set of microarray (viral infection HCV or HBV) in GEO Dataset experiments, as well as a response variable from each GSE10140-10141, by combination of gene regulatory net- experiment. The response variable might be a grouping work inference methods based on linear programming and such as untreated, treated, groups and so on. SAM com- decomposition procedures, with the CapitalBio MAS 3.0 putes a statistic di for each gene i, and measures strength software, based on integration of public databases includ- of relationship between gene expression and response ing Gene Ontology, KEGG, BioCarta, GenMapp, Intact, variable. It uses repeated permutations of data to deter- UniGene, OMIM, and more. mine whether expression of any genes is significantly Mechanisms that inhibit a signal are as important as related to the response. Cut-off for significance is deter- mechanisms that initiate it. The GRNInfer tool (14) identi- mined by a tuning parameter delta (chosen by the user) fies molecular activation and inhibition relationships based on the false positive rate. We normalized data by based on a novel mathematic method called Gene Net- log 2, selected two classes, paired and minimum fold work Reconstruction tool (GNR), using linear program- change ‡2, and chose significant (high expression genes ming and a decomposition procedure for inferring gene of HCC compared to non-malignancy associated hepati- networks. The method theoretically ensures derivation of tis ⁄ cirrhosis) genes under the false-discovery rate and the most consistent network structures with respect to all q-value being 0%. q-value [invented by John Storey the datasets, thereby not only significantly alleviating the (16)] is similar to the well-known P-value, but adapted problem of data scarcity but also remarkably improving to multiple-testing situations. reconstruction reliability. Here, we have compared the same and different, acti- Molecule annotation system vated and inhibited, CDKN3 networks with GO analysis, between non-malignancy associated hepatitis ⁄ cirrhosis Molecule Annotation System (MAS) is a web-based and HCC. Our results suggest CDKN3 cell cycle network software toolkit for whole data mining and function (i) with stronger DNA replication and weaker ubiquitin- annotation solution, to extract & analyse biological dependent protein catabolism, as common characteristics molecule relationships from public database. MAS uses in both non-malignancy associated hepatitis ⁄ cirrhosis and relational databases of biological networks created from HCC; (ii) with the stronger cell division and the weaker millions of individually modelled relationships between G2 phase checkpoint in non-malignancy associated hepa- genes, proteins, complexes, cells, disease and tissues. titis ⁄ cirrhosis; (iii) with stronger ell cycle and the weaker MAS allows view on our data, integrated in biological cytokinesis, as a result forming multinucleate cells in networks according to different kinds of biological con- HCC. Thus, it is useful to be able to identify the CDKN3 text. This unique feature results from multiple lines of cell cycle network for understanding molecular mecha- evidence, integrated in MASCORE. MAS helps com- nisms between non-malignancy associated hepatitis ⁄ prehension of relationships of gene expression data cirrhosis and HCC transformation. through the given molecular symbols list, and provides thorough, unbiased and visible results. Primary databases of MAS integrated various well- Materials and methods known biological resources including Genbank, EMBL, SwissProt, Gene Ontology, KEGG, BioCarta, GenMapp, Microarray data mirBase, EPD, HPRD, MIND, BIND, Intact, TRANS- We used microarrays containing 6144 genes from 25 FAC, UniGene, dbSNP, OMIM, InterPro, HUGO, MGI non-malignancy associated hepatitis ⁄ cirrhosis and 25 and RGD. MAS offers various query entries and graphical HCC patients, in the same GEO Dataset GSE10140- results. The system represents an alternative approach to 10141 (http://www.ncbi.nlm.nih.gov/geo/query/acc.cgi? mining biological signification for high-throughput array acc=GSE10140, http://www.ncbi.nlm.nih.gov/geo/query/ data (17). Ó 2011 Blackwell Publishing Ltd, Cell Proliferation, 44, 291–299. Relationship between CDKN3 and HCC 293 Network establishment of candidate genes Candidate novel activated and inhibited genes of CDKN3 upstream and downstream network in human The entire network was constructed using GRNInfer (42) non-malignancy associated hepatitis ⁄ cirrhosis and HCC and GVedit tools (http://www.graphviz.org/About.php). by GRNInfer GRNInfer is a novel mathematical method called GNR based on linear programming
Details
-
File Typepdf
-
Upload Time-
-
Content LanguagesEnglish
-
Upload UserAnonymous/Not logged-in
-
File Pages9 Page
-
File Size-