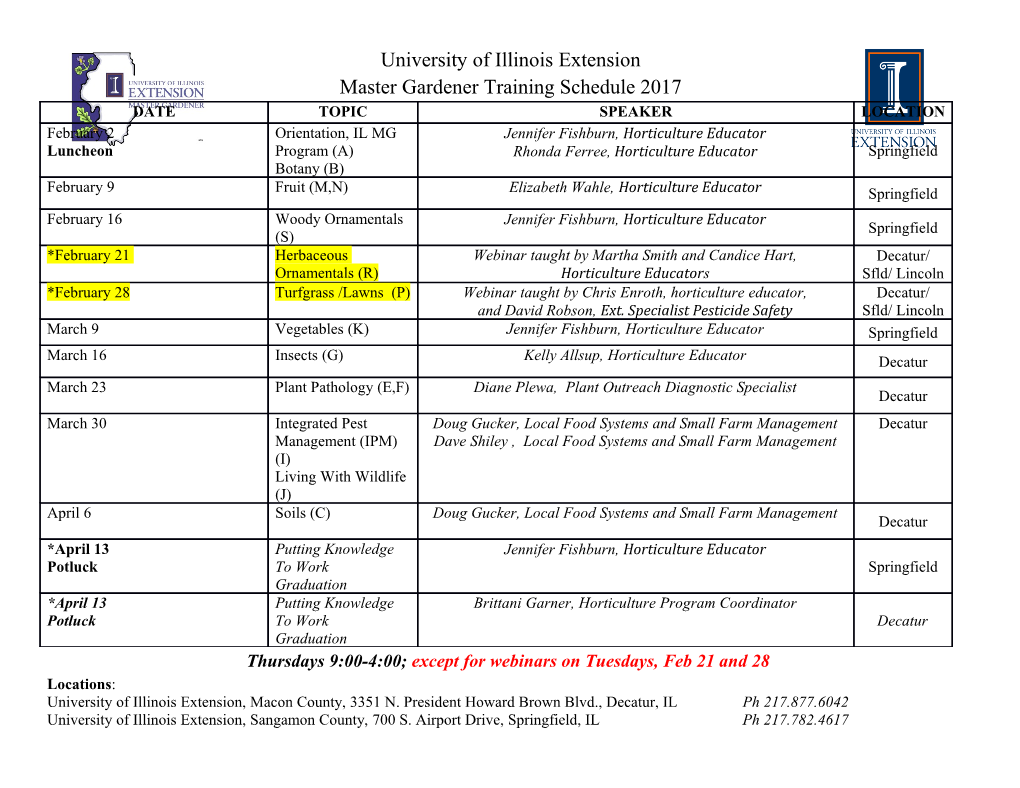
Data Mining the Genetics of Leukemia by Geoff Morton A thesis submitted to the School of Computing in conformity with the requirements for the degree of Master of Science Queen's University Kingston, Ontario, Canada January 2010 Copyright °c Geo® Morton, 2010 Library and Archives Bibliothèque et Canada Archives Canada Published Heritage Direction du Branch Patrimoine de l’édition 395 Wellington Street 395, rue Wellington Ottawa ON K1A 0N4 Ottawa ON K1A 0N4 Canada Canada Your file Votre référence ISBN: 978-0-494-65049-3 Our file Notre référence ISBN: 978-0-494-65049-3 NOTICE: AVIS: The author has granted a non- L’auteur a accordé une licence non exclusive exclusive license allowing Library and permettant à la Bibliothèque et Archives Archives Canada to reproduce, Canada de reproduire, publier, archiver, publish, archive, preserve, conserve, sauvegarder, conserver, transmettre au public communicate to the public by par télécommunication ou par l’Internet, prêter, telecommunication or on the Internet, distribuer et vendre des thèses partout dans le loan, distribute and sell theses monde, à des fins commerciales ou autres, sur worldwide, for commercial or non- support microforme, papier, électronique et/ou commercial purposes, in microform, autres formats. paper, electronic and/or any other formats. The author retains copyright L’auteur conserve la propriété du droit d’auteur ownership and moral rights in this et des droits moraux qui protège cette thèse. Ni thesis. Neither the thesis nor la thèse ni des extraits substantiels de celle-ci substantial extracts from it may be ne doivent être imprimés ou autrement printed or otherwise reproduced reproduits sans son autorisation. without the author’s permission. In compliance with the Canadian Conformément à la loi canadienne sur la Privacy Act some supporting forms protection de la vie privée, quelques may have been removed from this formulaires secondaires ont été enlevés de thesis. cette thèse. While these forms may be included Bien que ces formulaires aient inclus dans in the document page count, their la pagination, il n’y aura aucun contenu removal does not represent any loss manquant. of content from the thesis. Abstract Acute Lymphoblastic Leukemia (ALL) is the most common cancer in children under the age of 15. At present, diagnosis, prognosis and treatment decisions are made based upon blood and bone marrow laboratory testing. With advances in microarray technology it is becoming more feasible to perform genetic assessment of individual patients as well. We used Singular Value Decomposition (SVD) on Illumina SNP, A®ymetrix and cDNA gene-expression data and performed aggressive attribute se- lection using random forests to reduce the number of attributes to a manageable size. We then explored clustering and prediction of patient-speci¯c properties such as disease sub-classi¯cation, and especially clinical outcome. We determined that integrating multiple types of data can provide more meaningful information than individual datasets, if combined properly. This method is able to capture the cor- relation between the attributes. The most striking result is an apparent connection between genetic background and patient mortality under existing treatment regimes. We ¯nd that we can cluster well using the mortality label of the patients. Also, using a Support Vector Machine (SVM) we can predict clinical outcome with high accu- racy. This thesis will discuss the data-mining methods used and their application to biomedical research, as well as our results and how this will a®ect the diagnosis and treatment of ALL in the future. i Acknowledgments I would like to thank my supervisor Prof. David Skillicorn for the opportunity to work on this project, for all of the guidance he has given me along the way and for the chance to continue my work in Australia. The School of Computing at Queen's University has provided me not only with a wonderful education but also the funding that made this work possible and for that I am grateful. Thanks also to Dr. Daniel Catchpoole for providing a di®erent view into my work and making sure all of our results were practical and applicable, as well as for his hospitality for my time in Australia. To my friends and colleagues at the Children's Cancer Research Unit at The Children's Hospital at Westmead, I thank you for making my transition so easy and making it fun to come into work every day. And ¯nally I would like to thank my friends and family for their support throughout this whole process. Although my stories may sound like gibberish to you, you were always there to listen. ii Table of Contents Abstract i Acknowledgments ii Table of Contents iii List of Tables vi List of Figures viii Chapter 1: Introduction . 1 1.1 Problem . 1 1.2 My Contribution . 3 Chapter 2: Background . 4 2.1 Acute Lymphoblastic Leukemia (ALL) . 4 2.2 The Datasets . 7 2.3 Random Forests . 11 2.4 Singular Value Decomposition (SVD) . 13 iii 2.5 Support Vector Machine (SVM) . 15 2.6 Related Research . 16 Chapter 3: Experiments . 20 3.1 Pre-processing . 20 3.2 Normalization . 23 3.3 Experimental Model . 24 3.4 Combination of Datasets . 25 3.5 Attribute Selection . 26 3.6 Analysis of Selected Attributes . 27 3.7 Validation of Results . 28 3.8 Further SNP Analysis . 29 Chapter 4: Results . 31 4.1 SVD Results . 31 4.2 Combination of Datasets . 40 4.3 Analysis of Selected Data . 42 4.4 Attribute Selection . 61 4.5 Validation of Results . 81 4.6 Extended SNP Analysis . 82 4.7 Discussion of the Nature of the Data . 96 Chapter 5: Conclusion . 99 iv 5.1 Future Work . 101 Bibliography . 103 v List of Tables 2.1 Symptoms of ALL . 5 3.1 Random Forests Setup . 27 4.1 Combination Method 1 . 41 4.2 Combination Method 2 . 41 4.3 SNP Subset SVM Results . 42 4.4 cDNA Subset SVM Results . 48 4.5 A®y Subset SVM Results . 51 4.6 SNP and cDNA Subset SVM Results . 53 4.7 SNP and A®y Subset SVM Results . 56 4.8 cDNA and A®y Subset SVM Results . 57 4.9 SNP, cDNA and A®y Subset SVM Results . 60 4.10 Top 100 SNP Attributes . 62 4.11 Top 100 cDNA Attributes . 64 4.12 Top 100 A®y Attributes . 67 4.13 Top 100 SNP-cDNA Attributes . 70 4.14 Top 100 SNP-A®y Attributes . 72 4.15 Top 100 A®y-cDNA Attributes . 75 4.16 Top 100 SNP-cDNA-A®y Attributes . 78 vi 4.17 Label Shu²ing SVM Results . 82 4.18 SNP Relapse SVM Results . 85 4.19 Comparison of Top Attributes . 93 vii List of Figures 4.1 All SNP SVD . 32 4.2 All cDNA SVD . 33 4.3 All A®y SVD . 34 4.4 All Clinical SVD . 36 4.5 All SNP-cDNA SVD . 37 4.6 All SNP-A®y SVD . 38 4.7 All A®y-cDNA SVD . 39 4.8 SNP-cDNA-A®y SVD . 40 4.9 SNP Subset SVD . 45 4.10 SNP Subset SVD . 46 4.11 cDNA Subset SVD . 50 4.12 A®y Subset SVD . 52 4.13 SNP-cDNA Subset SVD . 55 4.14 SNP-A®y Subset SVD . 57 4.15 cDNA-A®y Subset SVD . 59 4.16 All Combined Subset SVD . 61 4.17 SNP Relapse SVD . 84 4.18 SNP Graph Analysis SVD . 87 4.19 Reformatted SNP Analysis SVD . 89 viii 4.20 250 SNP SVD for old and updated labels . 91 4.21 SNP SVD for updated labels . 92 4.22 Intersecting SNP SVD . 96 ix Chapter 1 Introduction 1.1 Problem Cancer, in all of its forms, is the second leading cause of death in the United States [15] and accounts for 13% of all deaths worldwide [25]. It is estimated that in the United States in 2009 a total of approximately 1.5 million people will have been diagnosed with cancer and of these, approximately 560,000 will die from their disease [22]. It is also estimated that approximately 30% of these cancer deaths are preventable [25]. The National Cancer Institute in Washington spends approximately $4.8 billion per year towards cancer research with most of the funding going towards breast, prostate, lung, colorectal and leukemia research [21]. Leukemia is the most common malignancy a®ecting children under the age of 15, but it also a®ects many adults. There are four subtypes of leukemia; acute lymphoblastic leukemia, chronic lymphoblastic leukemia, acute myelogenous leukemia and chronic myelogenous leukemia. It is estimated that approximately 45,000 new cases of leukemia will have been diagnosed in the United States in 2009 [16]. The 1 CHAPTER 1. INTRODUCTION 2 survival rate for persons with leukemia has dramatically increased over the past four decades. In the 1960s the ¯ve-year event-free survival rate was a mere 14%. In more recent years these ¯gures have been quoted as being as high as 80% [12, 17, 31]. Although there has been a signi¯cant improvement in the treatment of this disease, 20% of all leukemia cases result in death. With the completion of the Human Genome Project, the understanding of genetics has increased signi¯cantly. As such, many new technologies have been developed to study the genome in many di®erent forms. One of these technologies is the microarray, which is a high-throughput device allowing for the analysis of thousands of gene expression levels simultaneously. As a result, there is a wealth of data being generated every day for many di®erent purposes. The microarray has become a useful research tool and has allowed researchers to begin looking at problems on a much larger scale.
Details
-
File Typepdf
-
Upload Time-
-
Content LanguagesEnglish
-
Upload UserAnonymous/Not logged-in
-
File Pages120 Page
-
File Size-