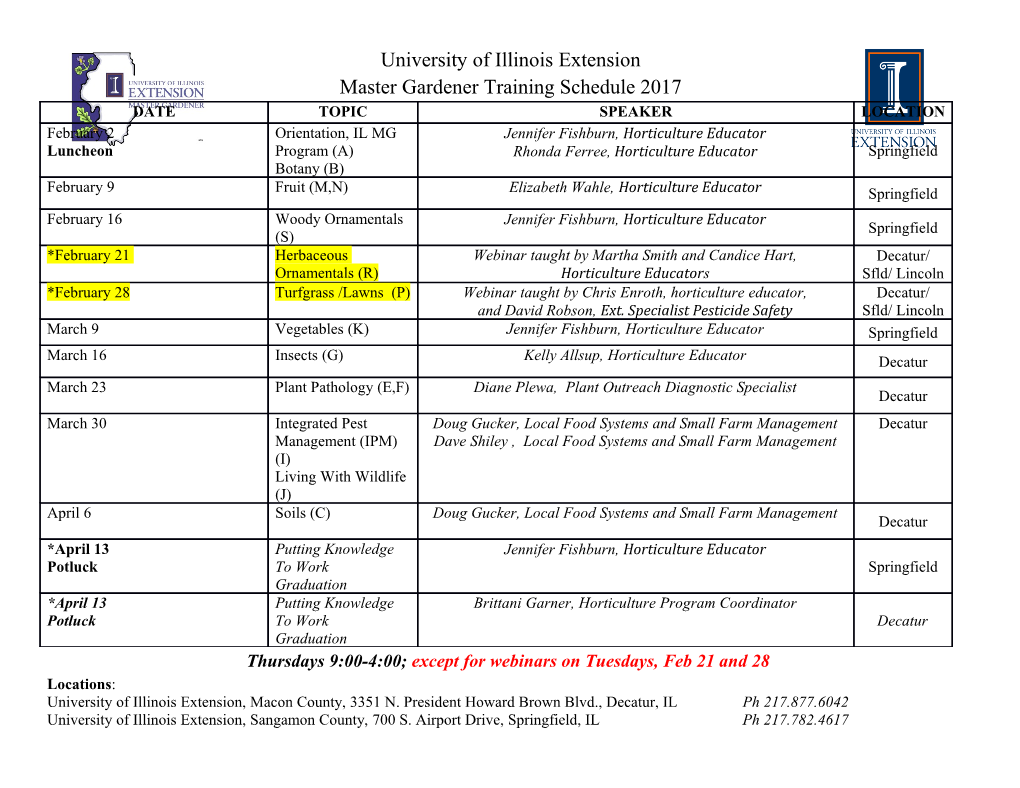
An Architecture for Accelerated Large-Scale Inference of Transformer-Based Language Models Amir Ganiev∗ and Colt Chapin and Anderson de Andrade and Chen Liu∗ Wattpad Toronto, ON, Canada [email protected], {colt, anderson}@wattpad.com, [email protected] Abstract other steps and can be computed in parallel, which can make both training and inference faster. BERT This work demonstrates the development pro- also easily accommodates different applications by cess of a machine learning architecture for in- allowing the fine-tuning of its parameters on dif- ference that can scale to a large volume of re- quests. In our experiments, we used a BERT ferent tasks. Despite these benefits, exposing these model that was fine-tuned for emotion analysis, models and communicating with them efficiently returning a probability distribution of emotions possesses some challenges. given a paragraph. The model was deployed as Machine learning frameworks are often used to a gRPC service on Kubernetes. Apache Spark train, evaluate, and perform inference on predic- was used to perform inference in batches by tive models. TensorFlow (Abadi et al., 2016) has calling the service. We encountered some per- formance and concurrency challenges and cre- been shown to be a reliable system that can operate ated solutions to achieve faster running time. at a large scale. A sub-component called Tensor- Starting with 3.3 successful inference requests Flow Serving allows loading models as services per second, we were able to achieve as high that handle inference requests concurrently. as 300 successful requests per second with the System architectures for inference have changed same batch job resource allocation. As a result, over time. Initial approaches favored offline set- we successfully stored emotion probabilities tings where batch jobs make use of distributed plat- for 95 million paragraphs within 96 hours. forms to load models and data within the same 1 Introduction process and perform inference. For example, Ijari, 2017 suggested an architecture that uses Apache As data in organizations becomes more available Hadoop (Hadoop, 2006) and Apache Pig for large- for analysis, it is crucial to develop efficient ma- scale data processing, where results are written chine learning pipelines. Previous work (Al-Jarrah to a Hadoop Distributed File System (HDFS) for et al., 2015) has highlighted the growing number later consumption. Newer distributed platforms of data centers and their energy and pollution reper- such as Apache Spark (Zaharia et al., 2016) have cussions. Machine learning models that require less gained prominence because of their memory opti- computational resources to generate accurate re- mizations and more versatile APIs, compared to sults reduce these externalities. On the other hand, Apache Hadoop (Zaharia et al., 2012). many machine learning applications also require As part of this architecture, inference services results in nearly real-time in order to be viable and would often be reserved for applications that may also require results from as many data samples require faster responses. The batch-based and as possible in order to produce accurate insights. service-based platforms have different use cases Hence, there are also opportunity costs associated and often run in isolation. Collocating data and with missed service-level objectives. models in a batch job has some disadvantages. Attention-based language models such as BERT Loading models in the same process as the data (Devlin et al., 2019) are often chosen for their forces them both to scale the same way. Moreover, relative efficiency, and empirical power. Com- models are forced to be implemented using the pro- pared to recurrent neural networks (Hochreiter and gramming languages supported by the distributed Schmidhuber, 1997), each step in a transformer data platform. Their APIs often place some limita- layer (Vaswani et al., 2017) has direct access to all tions on what can be done. ∗ Work done while the author was working at Wattpad. With the evolution of machine learning frame- 163 Proceedings of NAACL HLT 2021: IndustryTrack Papers, pages 163–169 June 6–11, 2021. ©2021 Association for Computational Linguistics works and container-orchestration systems such as when conducting model inference at scale in a Kubernetes,1 it is now simpler to efficiently build, MapReduce program such as Apache Spark is to deploy, and scale models as services. A scalable broadcast an instance of the model to each dis- architecture was presented in (Gómez et al., 2014) tributed worker process to allow for parallel pro- that proposes the use of RESTful API calls exe- cessing. However, when the footprint of these in- cuted by batch jobs in Hadoop to reach online ser- stances becomes too large, they begin to compete vices that provide real-time inference. Approaches with the dataset being processed for the limited like this simplify the architecture and address the memory resources of the underlying cluster and, in issues discussed previously. many cases, exceeding the capacity of the underly- In this work, we present an architecture for batch ing hardware. inference where a data processing task relies on ex- While this issue does not preclude the use of ternal services to perform the computation. The Apache Spark for running inferences on large mod- components of the architecture will be discussed in els at scale, it does complicate the process of im- detail along with the technical challenges and solu- plementing the job in a cost-efficient manner. It tions we developed to accelerate this process. Our is possible to allocate more resources, but because application is a model for emotion analysis that the clusters are static in size, a lot of work has to produces a probability distribution over a closed go into properly calculating resource allocation to set of emotions given a paragraph of text (Liu et al., avoid over or under-provisioning. This is where the 2019). We present benchmarks to justify our ar- idea of offloading the model to Kubernetes comes chitecture decisions and settings. The proposed into play. architecture is able to generate results for 95 mil- While our MapReduce clusters struggled to scale lion paragraphs within 96 hours. and accommodate the larger models being broad- casted, by leveraging Kubernetes we were able to 2 Architecture design monitor and optimize resource usage as well as We deployed our model as a TensorFlow service define autoscaling behaviors independently of this in a Kubernetes cluster. A sidecar service prepro- cluster. That said, while there are clear benefits to cessed and vectorized paragraphs and forwarded isolating your model from your MapReduce job requests to this service. We used gRPC to commu- we must now consider the added overhead of the nicate with the services,2 which is an efficient com- network calls and the effort to build and maintain munication protocol on HTTP/2. Both nearly real- containerized services. time and offline use cases made calls to these ser- 2.2 Kubernetes node pool vices. We used Apache Spark for batch processing, which we ran on Amazon’s AWS EMR service.3 To ensure optimal resource usage, we provisioned Our batch job was developed using Apache Spark’s a segregated node pool dedicated to hosting in- Python API (PySpark). The batch job fetched a stances of our models. A node pool is a collec- dataset of relevant paragraphs, called the inference tion of similar resources with predefined autoscal- service, and stored the results. The job had two ing behaviors. We leveraged Kubernetes’ built- modes: a backfill mode and a daily mode, which in taint/toleration functionality to establish the re- ran on a subset of mutated and new paragraphs. quired behavior. In Kubernetes, Taints designate This batch job was part of a data pipeline, sched- resources as non-viable for allocation, unless de- uled using Apache AirFlow4 and Luigi.5 Figure1 ployments are specifically annotated as having a shows the main components of this architecture. Toleration for said Taint. For this node pool, we selected instance types that offer faster CPUs, but 2.1 Kubernetes vs. Apache Spark provide an adequate amount of memory to load our One of the key issues we faced in scaling up our models. inference services was the growing size of the mem- 2.3 REST vs. gRPC ory footprint of an instance. A standard practice Once we made the decision to deploy our model 1https://kubernetes.io as a service, we had to determine which network 2https://grpc.github.io 3https://aws.amazon.com/emr protocol to use. While representational state trans- 4https://airflow.apache.org fer (REST) (Pautasso et al., 2013) is a well-known 5https://github.com/spotify/luigi standard, there were two aspects of our use case 164 Figure 1: Architecture overview. that made us consider alternatives. The first is that that there is enough disk capacity to process the architecturally, our use case was far more func- data and the number of cores is as high as possible tional in nature than REST. Second, the nature of without exceeding the cost constraints. Addition- our data means that request messages can be large. aly, we selected these to be memory-optimized to It was for this reason that we found the efficiency ensure we provide the job with enough RAM to offered by the Protobuf protocol a natural fit for efficiently process our joins. 6 our use case. The EMR cluster configuration is kept constant Having decided to use gRPC and Protobuf, we as a controlled variable throughout the project and encountered two issues. First, gRPC uses the in all of our experiments. This ensures that only HTTP/2 protocol which multiplexes requests over the implementation changes affect the performance a single persistent TCP connection. Because of this of the inference job. persistent connection, Layer-4 load balancers that can only route connections are not able to recog- nize requests within them that could be balanced 2.5 Monitoring across multiple replicas of a service.
Details
-
File Typepdf
-
Upload Time-
-
Content LanguagesEnglish
-
Upload UserAnonymous/Not logged-in
-
File Pages7 Page
-
File Size-