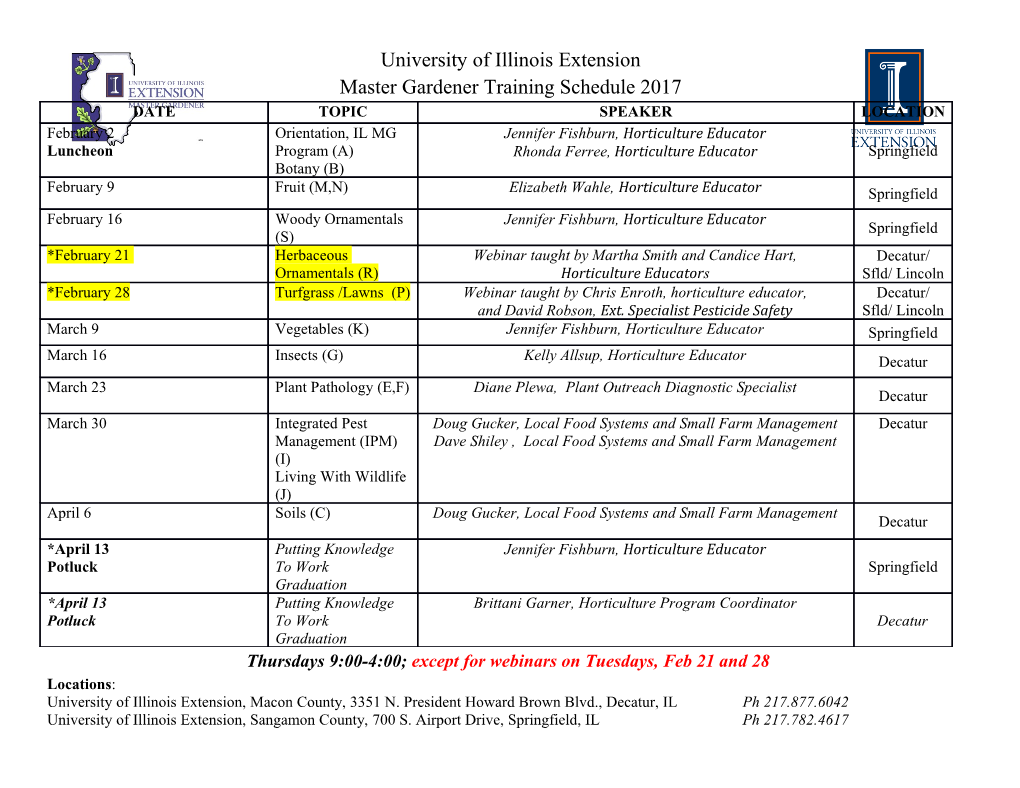
Proceedings of the Twenty-Sixth International Joint Conference on Artificial Intelligence (IJCAI-17) Modeling Hebb Learning Rule for Unsupervised Learning Jia Liu1, Maoguo Gong1∗, Qiguang Miao2 1Key Laboratory of Intelligent Perception and Image Understanding, Xidian University, Xi’an, China 2School of Computer Science and Technology, Xidian University, Xi’an, China [email protected], [email protected], [email protected] Abstract are unavailable. Various single-layer feature learning mod- els have been used and proposed since the layer-wise train- This paper presents to model the Hebb learning rule ing by restricted Boltzmann machine (RBM) [Hinton et al., and proposes a neuron learning machine (NLM). 2006] and auto-encoder (AE) [Bengio et al., 2006], includ- Hebb learning rule describes the plasticity of the ing principal component analysis (PCA) [Valpola, 2014], s- connection between presynaptic and postsynaptic parse coding [He et al., 2013], denoising AE [Vincent et al., neurons and it is unsupervised itself. It formulates 2010], and sparse versions of AE and RBM [Lee et al., 2007; the updating gradient of the connecting weight in Ji et al., 2014; Ranzato et al., 2007; Gong et al., 2015]. artificial neural networks. In this paper, we con- Good representations eliminate irrelevant variabilities of struct an objective function via modeling the Hebb the input data, while preserving the information that is useful rule. We make a hypothesis to simplify the mod- for the ultimate task [Ranzato et al., 2007]. For this purpose, el and introduce a correlation based constraint ac- an intuitive way is to construct the encoder-decoder model. cording to the hypothesis and stability of solution- AE, RBM, PCA and most unsupervised single-layer feature s. By analysis from the perspectives of maintain- learning models follow this paradigm. Based on the encoder- ing abstract information and increasing the ener- decoder architecture, more useful features can be learned by gy based probability of observed data, we find that adding constrains to extract more abstract concepts, includ- this biologically inspired model has the capabili- ing sparse constraints and sensitivity constraint [Rifai et al., ty of learning useful features. NLM can also be 2011]. Another type of learning models only have a decoder, stacked to learn hierarchical features and reformu- sparse coding for example. The above models can be taken as lated into convolutional version to extract features task based models which model the task and find the solution from 2-dimensional data. Experiments on single- that can well handle the task. However, some tasks are am- layer and deep networks demonstrate the effective- phibolous to define and model. The feature learning task for ness of NLM in unsupervised feature learning. instance, there are many ways to define the abstraction, e.g., sparse representation, noise corruption [Vincent et al., 2010], and contractive constraint [Rifai et al., 2011]. 1 Introduction In this paper, we propose a learning rule based model A promising but challenging research in artificial intelligence which attempts to model the learning process of neurons with has been mimicking the process the human brain represents less considerations of the task. The model consists of only information for decades [Arel et al., 2010]. Neural science the encoder. The learning behaviours of neurons have been findings [Kruger et al., 2013] have provided new ideas for researched for a long time for revealing the mechanism of designing information representation systems. This has mo- human cognition. One of the most famous theory is the Hebb tivated the development of hierarchical learning architectures learning rule [Hebb, 1949] proposed by Donald Olding Heb- and feature learning models. b. By applying the Hebb rule in the study of artificial neural Unsupervised feature learning aims to learn good represen- networks, we can obtain powerful models of neural compu- tations from unlabeled input data. One of the interests in un- tation that might be close to the function of structures found supervised learning is the stacked single-layer learning mod- in neural systems of many diverse species [Kuriscak et al., els for learning hierarchical representations [Hinton et al., 2015]. Recent works on Hebb learning are the series on “bi- 2006; Hinton and Salakhutdinov, 2006; Bengio et al., 2006; ologically plausible backprop” [Scellier and Bengio, 2016] 2013]. Unsupervised learning had a catalytic effect in reviv- by Bengio, et al. They linked Hebb and other biological- ing interest in deep neural networks [LeCun et al., 2015]. Al- ly formulated rules to back-propagation algorithm and used though it has little effect in many purely supervised appli- them to train the neural network. Hebb rule gives the updat- cations, it still works when large amount of labeled samples ing gradient of the connecting weights and it is unsupervised itself. We establish the model with the single layer network ∗Corresponding author as many unsupervised learning models and attempt to mod- 2315 Proceedings of the Twenty-Sixth International Joint Conference on Artificial Intelligence (IJCAI-17) el the updating gradient as the objective function. However, the architecture of artificial neural network is highly simpli- Hidden Hidden units units fied from the neural network in brain. Therefore, when the learning rule is implemented, several properties have to be Visible Visible considered [Kuriscak et al., 2015] in order to adapt to and units units reinforce the simplified network. We call the model neuron Max-clique (a) Max-clique (b) learning machine (NLM) and find that NLM has many simi- lar properties to other unsupervised feature learning models. Figure 1: Undirected graphical models of network with and with- From the analysis and experiments, NLM is capable of learn- out competition. (a) Network without competition (b) Network with ing useful features and outperform the compared task based competition models. For learning features from 2-dimensional data, e.g., images and videos, we also extend the model into a convolu- status of hidden units, including status of visible units, infor- tional version. mation from other nucleus, competition between hidden neu- This paper is organized in five sections. In the next section, rons and adjacent connections and limitations of the neuron the proposed NLM is formulated. In Section 3, we explain itself. Then, a connection of the simplified network has less how the NLM works and learns useful features. The exper- influence on h. Therefore, for convenience, we assume that imental studies are provided in Section 4. In Section 5, we the connecting weight has negligible influence on the con- conclude this paper. nected hidden unit. Under this omitting assumption, h can be taken as a set of constants wrt W and the integral is ap- P R P T parent, i.e., max hivj = Wij hivj = v W h. 2 The Model ij Wij ij Although this term is derived under the hypothesis, optimiz- The structure of NLM follows the common structure of ing this term will increase the correlation between Wij and single-layer neural network, e.g., the encoder of the AE and vjhi which is consistent with the Hebb rule. Specially, this RBM. The values of hidden units are computed by: term is also similar to the energy defined in RBM and Hop- field network. h = s(W v + b ) (1) i i i However, without the probabilistic model in RBM and the where h is the hidden vector and v is the visible vector. W recurrent architecture in Hopfield network, maximizing this and b denote the connecting weight matrix and bias vector term merely would not work because the simplified network respectively. s is the activation function and here we use the cannot approximate this assumption and guarantee the sta- sigmoid function s(x) = 1=(1 + exp(−x)). bility of solutions without any constraints. Components in T Hebb learning rule can be summarised by the most cited W will be +1 or −∞ when v W h is maximized. In or- sentence in [Hebb, 1949]: “When an axon of cell A is near der to make the network approximate the assumption and enough to excite a cell B and repeatedly or persistently takes guarantee the long term stability of W and h, a correlation part in firing it, some growth process or metabolic change based constraint is introduced. The assumption omits the takes place in one or both cells such that A’s efficiency, as one effect of W on the hidden units h. Therefore, both posi- of the cells firing B, is increased.” In the training of an artifi- tive and negative correlations between W and h should be cial neural network, Hebb rule describes the updating gradi- minimized which can be represented by the cosine between the two vectors cos2(h; W ) where W is the j-th row of ent of each connecting weight Wij = Wij + ∆Wij . This can :j :j be simplified by the product of the values of the units at the the matrix W . Meanwhile, the length of W and h should two ends of the connection: be limited in order to prevent the infinite increase or de- crease of W . Then the correlation constraint can be formu- ∆Wij = αhivj (2) lated as the square of inner product between the two vectors P 2 P 2 2 2 2 jhh; W:j i = j kW:j k2khk2 cos (h; W:j ) = kW hk2. where α is the learning rate. Hebb rule itself is an unsuper- With the simplified Hebb model and the correlation based vised learning rule which formulates the learning process of constraint, we can obtain the objective function of NLM: neurons. Therefore, we attempt to model the network based T 2 on this learning rule in order to learn in the neuronal way. max J(W; b) = v W h − λkW hk2 (3) With the gradient, a model can be obtained by integrating where λ is a user defined parameter that controls the impor- over ∆W .
Details
-
File Typepdf
-
Upload Time-
-
Content LanguagesEnglish
-
Upload UserAnonymous/Not logged-in
-
File Pages7 Page
-
File Size-