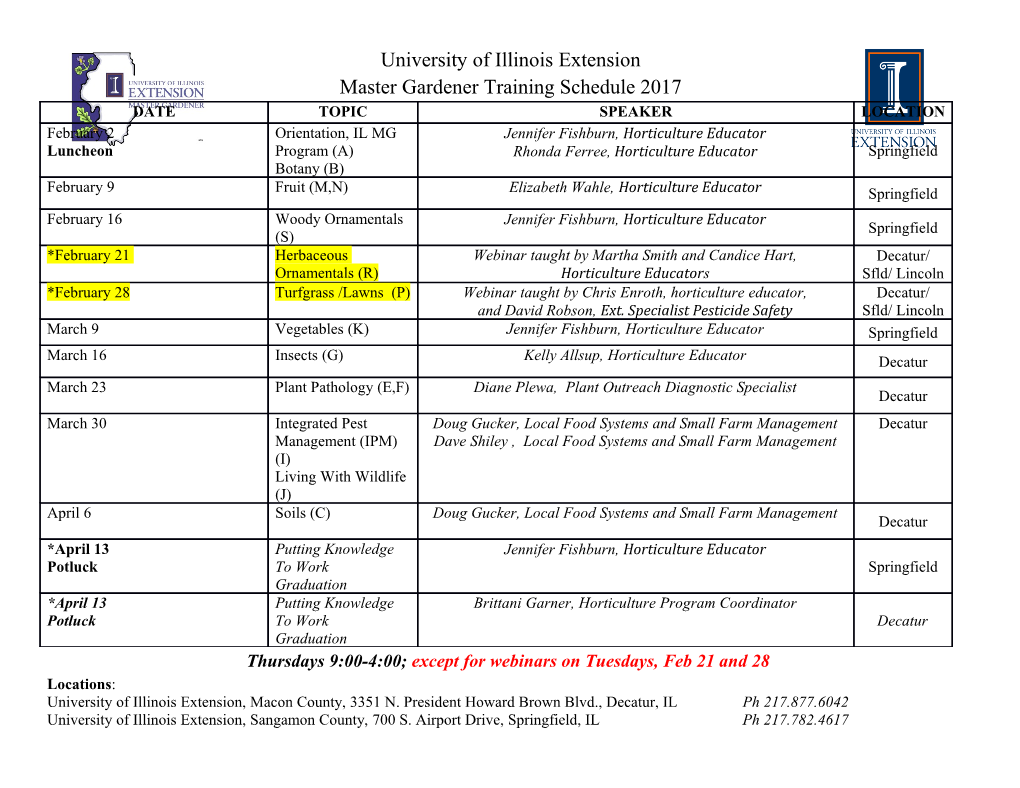
Numerical advances in pricing forward volatility sensitive equity derivatives Fiodar Kilin Centre for Practical Quantitative Finance Frankfurt School of Finance & Management A thesis submitted for the degree of Doktor rerum politicarum Supervisor: Prof. Dr. Uwe Wystup Submission date: July 7, 2009 Acknowledgements I am deeply grateful to my supervisor, Professor Uwe Wystup for many helpful suggestions, important advice and constant encouragement during this work. I also wish to thank Professor Rolf Poulsen, Professor Wolfgang Schmidt, Professor Robert Tompkins, Professor Eckhard Platen, Dr. Bernd Engel- mann, Dr. Peter Schwendner, Dr. Michael Dirkmann, Dr. Matthias Fen- gler, Dr. Friedrich Hubalek, Dr. Antonis Papapantoleon, Morten Nalholm and Martin Keller-Ressel for useful comments and discussions. I wish to express my gratitude to the sta® of the Frankfurt School of Finance & Management that have provided an excellent research environment. The ¯nancial support of Quanteam AG is gratefully acknowledged. Contents List of Figures v List of Tables vii 1 Introduction 1 2 Models 3 2.1 Heston model ................................. 3 2.2 Bates model .................................. 4 2.3 Barndor®-Nielsen&Shephard model with the Gamma-Ornstein-Uhlenbeck latent state .................................. 5 2.4 Levy models with stochastic time ...................... 6 2.5 Bergomi model ................................ 7 3 Pricing exotic options in stochastic volatility models 11 3.1 Model choice ................................. 11 3.2 Calibration and pricing of vanilla options ................. 13 3.3 Monte-Carlo pricing of exotic options .................... 17 3.4 Analytical pricing of exotic options ..................... 18 4 Accelerating the calibration of stochastic volatility models 21 4.1 Characteristic functions ........................... 22 4.2 Pricing methods ............................... 24 4.3 Caching technique .............................. 28 4.4 Numerical experiment ............................ 30 iii CONTENTS 5 Forward-start options in the Barndor®-Nielsen&Shephard model 35 5.1 Derivation ................................... 36 5.2 Numerical examples ............................. 39 6 On the cost of poor volatility modeling { The case of cliquets 51 6.1 Forward volatility and forward skew .................... 51 6.2 Cliquet options ................................ 53 6.3 Price comparison ............................... 56 6.4 Hedge performance .............................. 58 7 Conclusion 65 Bibliography 67 iv List of Figures 5.1 Price of a forward-start call in the Barndor®-Nielsen&Shephard model, sensitivity to comovement .......................... 40 5.2 Price of a forward-start call in the Barndor®-Nielsen&Shephard model, sensitivity to mean-reversion rate ...................... 40 5.3 Price of a forward-start call in the Barndor®-Nielsen&Shephard model, sensitivity to exponential law parameter .................. 41 5.4 Price of a forward-start call in the Barndor®-Nielsen&Shephard model, sensitivity to Poisson intensity ....................... 41 5.5 Price of a forward-start call in the Barndor®-Nielsen&Shephard model, sensitivity to initial latent state ....................... 42 5.6 Price and theta of a forward-start call in the Barndor®-Nielsen&Shephard model ..................................... 42 6.1 Histogram of relative cumulative hedging errors of a call spread cliquet. Hedging with recalibration using delta and short-term vega ....... 62 6.2 Histogram of relative cumulative hedging errors of a call spread cliquet. Hedging with constant parameters using delta and short-term vega ... 63 6.3 Histogram of relative cumulative hedging errors of a call spread cliquet. Hedging with constant parameters using delta and parallel shift vega .. 64 v LIST OF FIGURES vi List of Tables 3.1 Models examined and recommended in some of the existing literature on model risk. .................................. 14 4.1 Comparison of three methods for pricing vanilla options in stochastic volatility models. Grid sizes that are needed to obtain some benchmark accuracy levels ................................ 32 4.2 Comparison of three methods for pricing vanilla options in stochastic volatility models. Average calibration time ................ 33 5.1 Price of a forward-start call in the Barndor®-Nielsen&Shephard model, sensitivity to comovement .......................... 44 5.2 Price of a forward-start call in the Barndor®-Nielsen&Shephard model, sensitivity to mean-reversion rate ...................... 45 5.3 Price of a forward-start call in the Barndor®-Nielsen&Shephard model, sensitivity to exponential law parameter .................. 46 5.4 Price of a forward-start call in the Barndor®-Nielsen&Shephard model, sensitivity to Poisson intensity ....................... 47 5.5 Price of a forward-start call in the Barndor®-Nielsen&Shephard model, sensitivity to initial latent state ....................... 48 5.6 Price and theta of a forward-start call in the Barndor®-Nielsen&Shephard model ..................................... 49 6.1 Input parameters for pricing and hedging tests .............. 56 6.2 Input parameters for pricing and hedging tests .............. 56 6.3 Calibrated Heston parameters. ....................... 57 6.4 Calibrated Barndor®-Nielsen&Shephard parameters. ........... 57 vii LIST OF TABLES 6.5 Calibrated VGSA parameters. ........................ 57 6.6 Comparison of theoretical cliquet values. Scenario 1. ........... 58 6.7 Comparison of theoretical cliquet values. Scenario 2. ........... 58 6.8 Comparison of theoretical cliquet values. Scenario 3. ........... 59 6.9 Comparison of theoretical cliquet values. Scenario 4. ........... 59 6.10 Comparison of theoretical cliquet values. Scenario 5. ........... 59 viii GLOSSARY Glossary This glossary contains abbreviations for the models used in this thesis, the full name and a reference to a page, where they are ¯rst de¯ned. Bates 4 Bergomi 7 BNS (Barndor®-Nielsen&Shephard) 36 BNS-GOU (Barndor®-Nielsen&Shephard model with the Gamma-Ornstein-Uhlenbeck latent state) 5 CEV (Constant elasticity of variance) 8 Heston 3 NIG-CIR (Normal Inverse Gaussian with the Cox-Ingersoll-Ross stochastic clock) 6 NIG-GOU (Normal Inverse Gaussian with the Gamma-Ornstein-Uhlenbeck stochastic clock) 6 VG-CIR (Variance Gamma with the Cox-Ingersoll-Ross stochastic clock 6 VG-GOU (Variance Gamma with the Gamma-Ornstein-Uhlenbeck stochastic clock) 6 VGSA (Variance Gamma with stochastic arrival) 6 ix GLOSSARY x 1 Introduction The main purpose of this thesis is to describe new numerical and analytical methods for pricing equity derivatives in stochastic volatility models. We introduce an inno- vative method of high-speed calibration for this class of models. We also develop an e±cient method of pricing forward-start options. Using this method we derive a ready- to-implement formula for this type of contracts. The described results are supported by numerical examples and description of thorough tests. Before describing the main results, we provide a literature overview that motivates introducing the new calibration and pricing techniques. We analyse applications of the introduced methods in a±ne stochastic volatility models (e.g., the models of Heston, Bates, Barndor®-Nielsen&Shephard) and the Levy models with stochastic time change. Furthermore, we illustrate the use of these methods for model risk analysis and evaluating hedging performance of stochastic volatility models. Among the contracts which we consider are forward start options, variance swaps, vola- tility swaps, options on realized variance, reverse cliquets, accumulators and Napoleons. The obtained results are important in both practical and academic applications. Attrac- tive bene¯ts from using the described innovative methods in the quantitative analysis departments of investment banks are speed and accuracy of calculations, stability of calibrated model parameters in time direction, stability and reliability of greeks. From the point of view of academic research the introduced techniques signi¯cantly acceler- ate experiments that require numerous recalculations of prices in di®erent models and under di®erent market conditions. 1 1. INTRODUCTION The advantages of stochastic volatility models1 over local volatility models are the pos- sibility to produce a realistic forward smile, the leverage e®ect, the volatility clustering, the possibility to ¯t the whole market implied volatility surface with ¯ve or six param- eters only, the possibility of hedging second order greeks with respect to volatility (i.e. volga and vanna). A natural question is often posed: Why are stochastic volatility models not used ex- tensively in equity derivatives modeling, although they possess a lot of theoretical ad- vantages? We point out following reasons for this situation: (1) In calm markets (e.g. 2004-2007), the local volatility model performs quite well and it is easier to handle. This statement is corroborated by tests described in Engelmann et al.(2006b). Mercurio & Morini(2009) also show that that the dynamic and hedging behaviors of local volatility and stochastic volatility models are not qualitatively as di®erent as it is assumed in current market wisdom. (2) Application of stochastic volatility models often leads to calibration bias in cases of steep implied volatility skew. This bias negatively a®ects the quality of the valuation of down-and-out puts and makes static hedge strategies for reverse-knock-out options even more complicated.
Details
-
File Typepdf
-
Upload Time-
-
Content LanguagesEnglish
-
Upload UserAnonymous/Not logged-in
-
File Pages84 Page
-
File Size-