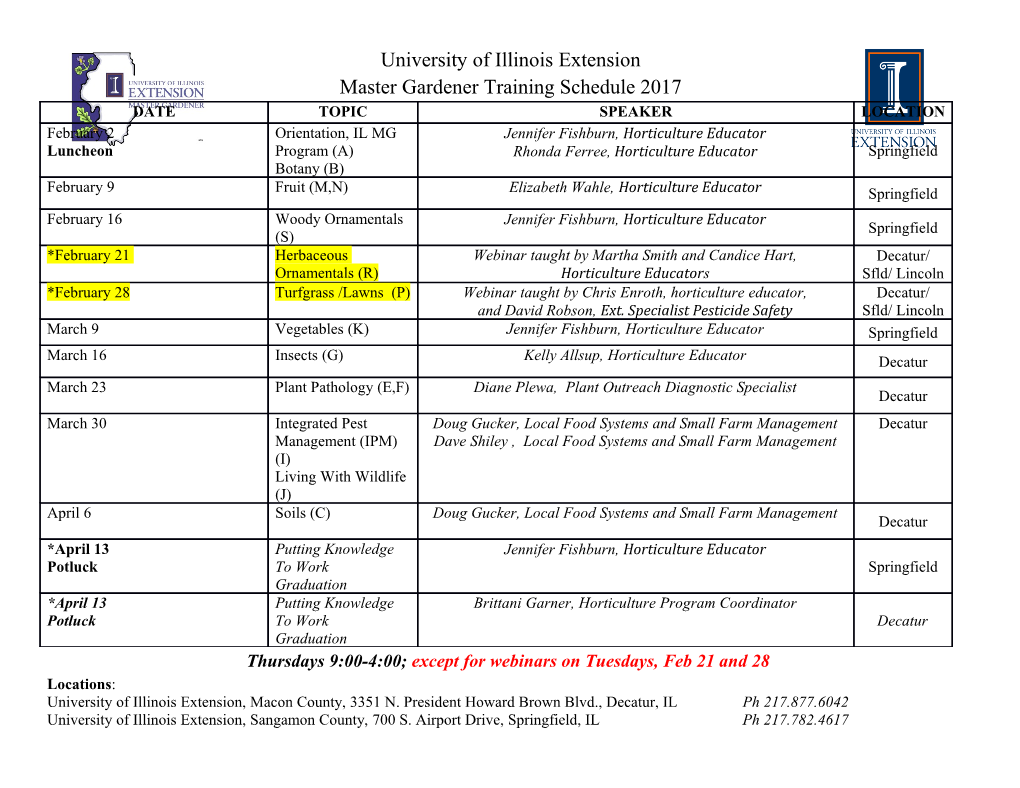
CPXXXX10.1177/2167702614565359Wiecki et al.Computational Psychiatry: Clustering and Classification 565359research-article2015 Special Series: Computational Psychiatry Clinical Psychological Science 2015, Vol. 3(3) 378 –399 Model-Based Cognitive Neuroscience © The Author(s) 2015 Reprints and permissions: sagepub.com/journalsPermissions.nav Approaches to Computational Psychiatry: DOI: 10.1177/2167702614565359 Clustering and Classification cpx.sagepub.com Thomas V. Wiecki, Jeffrey Poland, and Michael J. Frank Department of Cognitive, Linguistic, and Psychological Science, Brown University Abstract Psychiatric research is in crisis. We highlight efforts to overcome current challenges by focusing on the emerging field of computational psychiatry, which might enable the field to move from a symptom-based description of mental illness to descriptors based on objective computational multidimensional functional variables. We survey recent efforts toward this goal and describe a set of methods that together form a toolbox to aid this research program. We identify four levels in computational psychiatry: (a) behavioral tasks that index various psychological processes, (b) computational models that identify the generative psychological processes, (c) parameter-estimation methods concerned with quantitatively fitting these models to subject behavior by focusing on hierarchical Bayesian estimation as a rich framework with many desirable properties, and (d) machine-learning clustering methods that identify clinically significant conditions and subgroups of individuals. As a proof of principle, we apply these methods to two different data sets. Finally, we highlight challenges for future research. Keywords computational psychiatry, drift diffusion model, computational cognitive neuroscience, RDoC, response inhibition Received 9/27/13; Revision accepted 7/15/14 Imagine going to a doctor because of chest pain that has the heart, our understanding of the normally functioning been bothering you for a couple of weeks. The doctor brain is still, arguably, in its infancy. would sit down with you, listen carefully to your descrip- Despite this complexity, concerted efforts in the brain tion of symptoms, and prescribe medication to lower sciences have led to an explosion of knowledge and blood pressure in case you have a heart condition. After understanding about the healthy and diseased brain in a couple of weeks, your pain has not subsided. The doc- the past decades. The discovery of highly effective psy- tor now prescribes medication against reflux, which choactive drugs in the 1950s and 1960s raised expecta- finally seems to help. In this scenario, not a single medi- tions that psychiatry would progress in a similar fashion. cal analysis (e.g., electrocardiogram, blood work, or a It is unfortunate that, in retrospect, it appears that these gastroscopy) was performed, and medication with poten- discoveries were serendipitous in nature, given that little tially severe side effects was prescribed on a trial-and- progress has been made since (e.g., Hyman, 2012; Insel error basis. Although highly unlikely to occur if you et al., 2010). This lack of progress also has caused many walked into a primary care unit with these symptoms major pharmaceuticals companies, such as AstraZeneca today, this scenario resembles much of contemporary and GlaxoSmithKline, to withdraw from psychiatric drug psychiatry diagnosis and treatment. development and to close large research centers (Cressey, There are several reasons for this discrepancy in sophistication between psychiatry and other fields of medicine. First and foremost, mental illness affects the Corresponding Authors: brain—the most complex biological system yet encoun- Michael J. Frank and Thomas V. Wiecki, Department of Cognitive, Linguistic, and Psychological Science, Brown University, 190 Thayer tered. Compared with the level of scientific understand- St., Providence, RI 02912-1821 ing achieved on other organs of the human body, such as E-mail: [email protected] and [email protected] Downloaded from cpx.sagepub.com by guest on May 5, 2015 Computational Psychiatry: Clustering and Classification 379 2011; Nutt & Goodwin, 2011). In addition, research on after nuisance variables are regressed out and that this mental illness, based on conventional psychiatric diag- clustering shows consistently better recovery of the age- nostic categories and practices (as reflected in the various groups than if behavioral summary statistics (e.g., mean editions of the Diagnostic and Statistical Manual of RT and accuracy) are used alone. Moreover, factor analy- Mental Disorders, DSM; e.g., American Psychiatric sis on the computational parameters extracts meaningful Association, 2013), has been widely viewed as disap- latent variables that describe cognitive ability. For this pointing, and the DSM system of classification itself has data set, no identified brain-based mechanism was ana- been viewed as an impediment to more productive lyzed. In contrast, for the second data set, we relied on a research. As a consequence, psychiatry is a field in crisis hypothesis-driven approach that suggested a mechanism (Hyman, 2012; Insel et al., 2010; Poland, Von Eckardt, & for how a specific decision parameter—the decision Spaulding, 1994; Sahakian, Malloch, & Kennard, 2010). threshold—varies as a function of activity communicated As outlined in more detail later, a central issue is a lack between frontal cortex and the subthalamic nucleus of sufficiently powerful theoretical and methodological (STN). A previous study showed that STN deep-brain resources for managing the features of mental illness stimulation disrupted decision-threshold regulation (e.g., a lack of measurable quantitative descriptors). This across a group of patients with Parkinson’s disease (PD; lacuna prevents effective management of the multidi- Cavanagh et al., 2011). In the following, we show that we mensional hierarchical complexity, dynamic interactivity, can classify individual patients’ brain-stimulation status causal ambiguity, and heterogeneity of mental illness. (off or on) with relatively high accuracy, given model And it leads to an explanatory gap of how basic neuro- parameters, and better than that achieved on the basis of biological processes and other causes result in complex brain-behavior correlations alone. disorders of the mind (Hyman, 2012; Montague, Dolan, Friston, & Dayan, 2011). Current Challenges in Psychiatry In the present study, we review current challenges in psychiatry and recent efforts to overcome them. Several The current crisis in psychiatry has complex causes that examples from the domain of decision making show the are deeply rooted in existing classification systems (e.g., promise of moving away from symptom-based descrip- DSM, International Classification of Diseases). In this sec- tion of mental illness and instead formulating objective, tion, we identify some of the problems these systems quantifiable computational biomarkers as a basis for fur- introduce and provide indications of the sorts of resources ther psychiatric research. We then introduce a computa- required for more productive research programs. In the tional cognitive toolbox that is suited to construct these subsequent section, we review recent attempts to meet computational biomarkers. We focus on sequential sam- these challenges and the sorts of resources that have pling models (SSMs) of decision making, which serve as been introduced for this purpose. As other researchers a case study for how computational models, when fit to before us have done, we proceed to suggest an approach behavior, have successfully been used to identify and to research of mental disorders that aims to link cognitive quantify latent neurocognitive processes in healthy and pure neuroscience to mental illness without the humans. Bayesian methods provide a resourceful frame- restrictions of prior classification schemes (Robbins, work to fit these models to behavior and establish indi- Gillan, Smith, de Wit, & Ersche, 2012). vidualized descriptors of neurocognitive function. After establishing the validity of these models to provide neu- DSM and research rocognitive descriptors of individuals, we review how clustering techniques can be used to construct a map of For decades, the DSM has been the basis of clinical diag- individual differences based on these neurocognitive nosis, treatment, and research of mental illness. At its descriptors. core, the DSM defines distinct disorder categories, such To demonstrate the viability and potential of these as schizophrenia (SZ) and depression, in a way that is methods, we reanalyze two data sets, thereby providing atheoretical (i.e., with no reference to specific causal a proof of principle before discussing future challenges hypotheses) and focused on clinical phenomenology. in application to psychiatric populations. The first data Thus, these categories are mainly derived from translat- set consists of a group of young and old subjects who ing subjective experience to objective symptomatology performed three different decision-making tasks (Ratcliff, while assuming unspecified biological, psychological, or Thapar, & McKoon, 2010). After fitting subjects’ choices behavioral dysfunctions (Poland et al., 1994). and response-time (RT) distributions with the drift- Although primarily intended to be of value to clini- diffusion model (DDM) using hierarchical Bayesian cians, the DSM has also played a substantial role as a parameter estimation,
Details
-
File Typepdf
-
Upload Time-
-
Content LanguagesEnglish
-
Upload UserAnonymous/Not logged-in
-
File Pages22 Page
-
File Size-