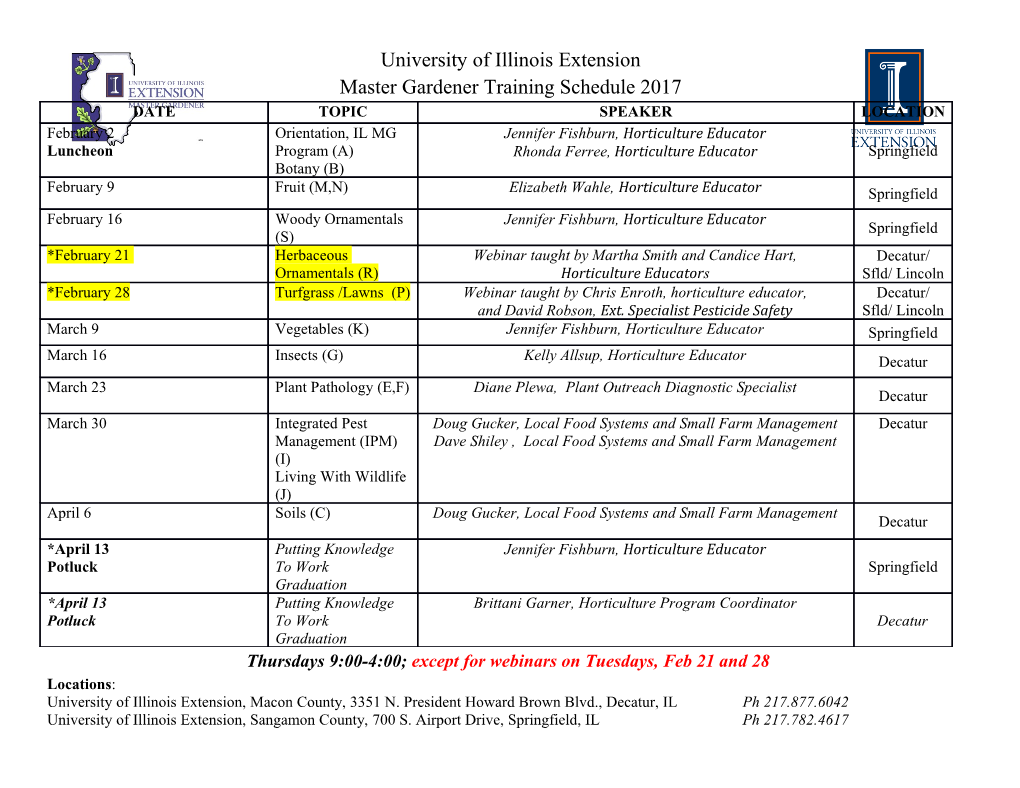
Ten Experiments on the Modeling of Polyphonic Timbre Jean-Julien Aucouturier To cite this version: Jean-Julien Aucouturier. Ten Experiments on the Modeling of Polyphonic Timbre. Sound [cs.SD]. Université Pierre et Marie Curie (Paris 6), 2006. English. tel-01970963 HAL Id: tel-01970963 https://hal.archives-ouvertes.fr/tel-01970963 Submitted on 18 Jan 2019 HAL is a multi-disciplinary open access L’archive ouverte pluridisciplinaire HAL, est archive for the deposit and dissemination of sci- destinée au dépôt et à la diffusion de documents entific research documents, whether they are pub- scientifiques de niveau recherche, publiés ou non, lished or not. The documents may come from émanant des établissements d’enseignement et de teaching and research institutions in France or recherche français ou étrangers, des laboratoires abroad, or from public or private research centers. publics ou privés. 2 Resum´ e´ La grande majorite´ des systemes` d’extraction de metadonnees´ haut-niveau a` partir de signaux mu- sicaux repose sur un modele` implicite de leur “son” ou timbre polyphonique. Ce modele` represente´ le timbre comme la distribution statistique globale d’attributs spectraux instantanes,´ calcules´ sur des trames de quelques dizaines de millisecondes. L’hypothese` sous-jacente, rarement explicitee,´ est que le timbre perc¸u d’une texture polyphonique correspond a` ses attributs instantanes´ les plus represent´ es´ statistiquement. Cette these` remet en cause la validite´ de cette hypothese.` Pour ce faire, nous construisons une mesure explicite de la similitude timbrale entre deux textures poly- phoniques, declin´ ee´ sous un grand nombre de variantes typiques du domaine. Nous montrons que la precision´ de telles mesures est limitee´ et que leur taux d’erreur residuel´ n’est pas accidentel. No- tamment, cette classe de mesures tend a` creer´ de faux-positifs qui sont toujours les memeˆ chansons, independamment´ de la requeteˆ de depart:´ des hubs. Leur etude´ etablit´ que l’importance perceptuelle des attributs instantanes´ ne depend´ pas de leur saillance statistique par rapport a` leur distribution a` long-terme. En d’autres termes, nous “entendons” quotidiennement dans la musique polyphonique des choses qui ne sont pourtant pas presentes´ de fac¸on significative (statistiquement) dans le signal sonore, mais qui sont plutotˆ le resultat´ de raisonnement cognitifs evolu´ es,´ dependant´ par exemple du contexte d’ecoute´ et de la culture de l’auditeur. La musique que nous entendons etreˆ du piano est surtout de la musique que nous nous attendons a` etreˆ du piano. Ces paradoxes statistico-perceptifs expliquent en grande partie le desaccord´ entre les modeles` etudi´ es´ ici et la perception humaine. i Abstract The majority of systems extracting high-level music descriptions from audio signals rely on a com- mon, implicit model of the global sound or polyphonic timbre of a musical signal. This model represents the timbre of a texture as the long-term distribution of its local spectral features. The underlying assumption is rarely made explicit: the perception of the timbre of a texture is assumed to result from the most statistically significant feature windows. This thesis questions the validity of this assumption. To do so, we construct an explicit measure of the timbre similarity between polyphonic music textures, and variants thereof inspired by previous work in Music Information Retrieval. We show that the precision of such measures is bounded, and that the remaining error rate is not incidental. Notably, this class of algorithms tends to create false positives - which we call hubs - which are mostly always the same songs regardless of the query. Their study shows that the perceptual saliency of feature observations is not necessarily correlated with their statistical signifi- cance with respect to the global distribution. In other words, music listeners routinely “hear” things that are not statistically significant in musical signals, but rather are the result of high-level cognitive reasoning, which depends on cultural expectations, a priori knowledge, and context. Much of the music we hear as being “piano music” is really music that we expect to be piano music. Such statis- tical/perceptual paradoxes are instrumental in the observed discrepancy between human perception of timbre and the models studied here. iii Table of Contents 1 Introduction 1 I Epistemology 7 2 Dimensions of Timbre 9 2.1 Everything but pitch and loudness . 9 2.2 Psychophysicalstudies ............................... 10 2.3 Automatic recognition of monophonic timbres . 12 2.4 Towards polyphonic textures . 14 2.4.1 The demand of Electronic Music Distribution . 14 2.4.2 The lack of perceptive models . 17 2.5 Implicit modelling . 23 2.6 Thesis Overview: Ten Experiments . 26 3 Dimensions of Timbre Models 31 3.1 The Prototypical algorithm . 32 3.1.1 Feature Extraction . 32 3.1.2 Feature Distribution Modelling . 33 3.1.3 DistanceMeasure............................... 35 3.2 MIR Design Patterns and Heuristics . 39 3.2.1 Pattern: Tuning feature parameters . 41 3.2.2 Pattern: Tuning model parameters . 43 3.2.3 Pattern: Feature Equivalence . 46 3.2.4 Pattern: Model Equivalence . 49 v vi TABLE OF CONTENTS 3.2.5 Pattern: Feature Composition . 50 3.2.6 Pattern: Cross-Fertilisation . 51 3.2.7 Pattern: Modelling Dynamics . 52 3.2.8 Pattern: Higher-level knowledge . 55 3.3 Conclusion ...................................... 57 II Experiments 59 4 Experiment 1: The Glass Ceiling 61 4.1 Experiment ...................................... 62 4.2 Method ........................................ 65 4.2.1 Explicit modelling . 65 4.2.2 GroundTruth................................. 65 4.2.3 Evaluation Metric . 66 4.3 Tools.......................................... 66 4.3.1 Architecture.................................. 67 4.3.2 Implementations . 69 4.3.3 Algorithms . 69 4.4 Results......................................... 70 4.4.1 BestResults.................................. 70 4.4.2 Significance.................................. 71 4.4.3 Dynamics don’t improve . 72 4.4.4 “Everything performs the same” . 72 4.4.5 Existence of a glass ceiling . 73 4.4.6 False Positives are very badmatches..................... 75 4.4.7 Existenceofhubs............................... 76 5 Experiment 2: The Usefulness of Dynamics 77 5.1 TheparadoxofDynamics............................... 77 5.2 Hypothesis ...................................... 78 5.3 Method ........................................ 79 5.3.1 Databases................................... 79 5.3.2 Algorithms . 81 5.3.3 EvaluationProcedure............................. 84 5.4 Results......................................... 84 TABLE OF CONTENTS vii 6 Experiments 3-8: Understanding Hubs 89 6.1 Definition....................................... 89 6.2 Why this may be an important problem . 90 6.3 Measuresofhubness ................................ 92 6.3.1 Numberofoccurrences............................ 92 6.3.2 Neighborangle................................ 94 6.3.3 Correlation between measures . 96 6.4 Power-law Distribution . 98 6.5 Experiment3:FeaturesorModel?. 101 6.5.1 Hypothesis .................................. 101 6.5.2 Experiment.................................. 101 6.5.3 Results .................................... 102 6.6 Experiment 4: Influence of modelling . 102 6.6.1 Hypothesis .................................. 102 6.6.2 Experiment.................................. 103 6.6.3 Results .................................... 103 6.7 Experiment 5: Intrinsic or extrinsic to songs ? . 105 6.7.1 Hypothesis .................................. 105 6.7.2 Experiment.................................. 105 6.7.3 Results .................................... 106 6.8 Experiment 6: The seductive, but probably wrong, hypothesis of equivalence classes 109 6.8.1 Hypothesis .................................. 109 6.8.2 Experiment.................................. 111 6.8.3 Results .................................... 112 6.9 Experiment 7: On homogeneity . 112 6.9.1 Hypothesis .................................. 112 6.9.2 Experiment.................................. 113 6.9.3 Results .................................... 114 6.10 Experiment 8: Are hubs a structural property of the algorithms ? . 118 6.10.1 Hypothesis .................................. 118 6.10.2 Experiment .................................. 118 6.10.3 Results .................................... 120 7 Experiments 9 & 10: Grounding 125 7.1 Experiment 9: Inferring high-level descriptions with timbre similarity . 126 7.1.1 Material.................................... 126 viii TABLE OF CONTENTS 7.1.2 Methods ................................... 127 7.1.3 Results .................................... 129 7.2 Experiment10:Theuseofcontext . 133 7.2.1 Method .................................... 133 7.2.2 Results .................................... 134 7.2.3 Exploiting correlations with decision trees . 137 7.3 An operational model for grounding high-level descriptions . ....... 138 7.3.1 Algorithm................................... 140 7.3.2 Preliminary results . 142 8 Conclusion: Toward Cognitive Models 147 III Synthese` en franc¸ais - Digest in French 151 IV Appendices 183 A Composition of the test database 185 B Experiment 1 - Details 189 B.1 Tuning feature and model parameters (patterns 3.2.1 and 3.2.2) . 189 B.1.1 influenceofSR................................ 190 B.1.2 influenceofDSR ............................... 191 B.1.3 influenceofN,M ............................... 191 B.1.4 influence of Windows
Details
-
File Typepdf
-
Upload Time-
-
Content LanguagesEnglish
-
Upload UserAnonymous/Not logged-in
-
File Pages302 Page
-
File Size-