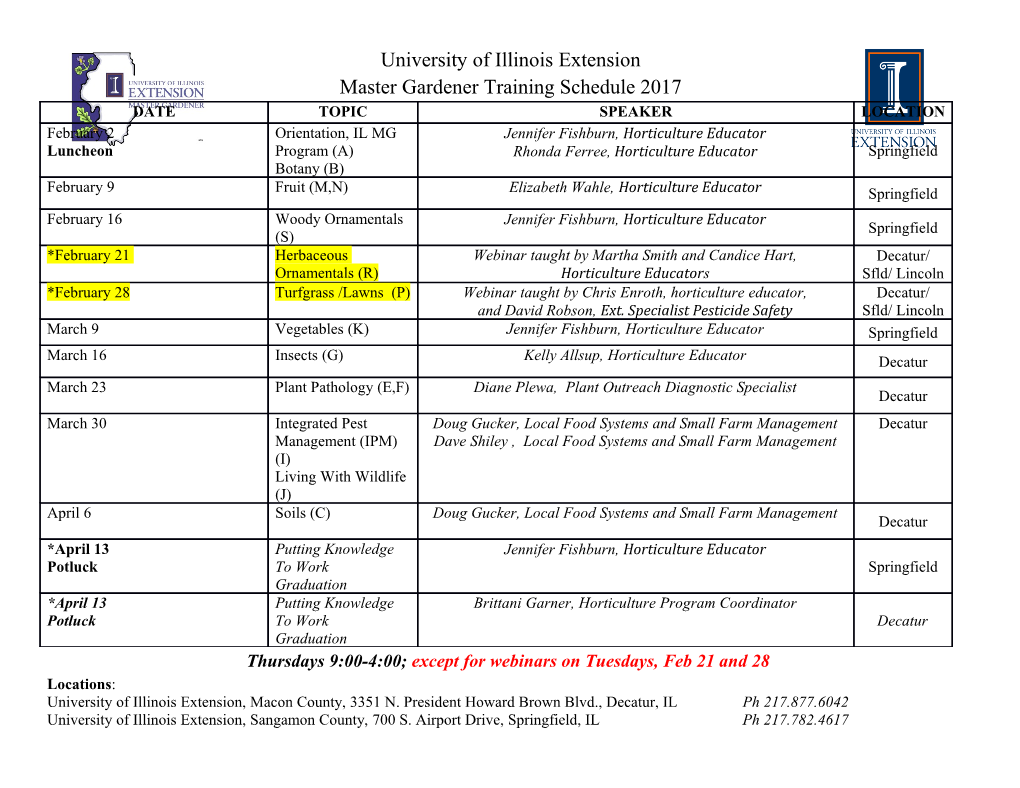
Bayesian Macroeconometrics Marco Del Negro Frank Schorfheide⇤ Federal Reserve Bank of New York University of Pennsylvania CEPR and NBER April 18, 2010 Prepared for Handbook of Bayesian Econometrics ⇤Correspondence: Marco Del Negro: Research Department, Federal Reserve Bank of New York, 33 Liberty Street, New York NY 10045: [email protected]. Frank Schorfheide: Depart- ment of Economics, 3718 Locust Walk, University of Pennsylvania, Philadelphia, PA 19104-6297. Email: [email protected]. The views expressed in this chapter do not necessarily reflect those of the Federal Reserve Bank of New York or the Federal Reserve System. Ed Herbst and Maxym Kryshko provided excellent research assistant. We are thankful for the feedback received from the editors of the Handbook John Geweke, Gary Koop, and Herman van Dijk as well as comments by Giorgio Primiceri, Dan Waggoner, and Tao Zha. Del Negro, Schorfheide – Bayesian Macroeconometrics: April 18, 2010 2 Contents 1 Introduction 1 1.1 Challenges for Inference and Decision Making . 1 1.2 How Can Bayesian Analysis Help? . 2 1.3 Outline of this Chapter . 4 2 Vector Autoregressions 7 2.1 A Reduced-Form VAR . 8 2.2 Dummy Observations and the Minnesota Prior . 10 2.3 A Second Reduced-Form VAR . 14 2.4 Structural VARs . 16 2.5 Further VAR Topics . 29 3 VARs with Reduced-Rank Restrictions 29 3.1 Cointegration Restrictions . 30 3.2 Bayesian Inference with Gaussian Prior for β ............. 32 3.3 Further Research on Bayesian Cointegration Models . 35 4 Dynamic Stochastic General Equilibrium Models 38 4.1 A Prototypical DSGE Model . 39 4.2 Model Solution and State-Space Form . 41 4.3 Bayesian Inference . 44 4.4 Extensions I: Indeterminacy . 49 4.5 Extensions II: Stochastic Volatility . 51 4.6 Extension III: General Nonlinear DSGE Models . 52 4.7 DSGE Model Evaluation . 54 4.8 DSGE Models in Applied Work . 61 Del Negro, Schorfheide – Bayesian Macroeconometrics: April 18, 2010 0 5 Time-Varying Parameters Models 62 5.1 Models with Autoregressive Coefficients . 63 5.2 Models with Markov-Switching Parameters . 68 5.3 Applications of Bayesian TVP Models . 73 6 Models for Data-Rich Environments 74 6.1 Restricted High-Dimensional VARs . 75 6.2 Dynamic Factor Models . 78 7 Model Uncertainty 90 7.1 Posterior Model Probabilities and Model Selection . 91 7.2 Decision Making and Inference with Multiple Models . 95 7.3 Difficulties in Decision-Making with Multiple Models . 99 Del Negro, Schorfheide – Bayesian Macroeconometrics: April 18, 2010 1 1 Introduction One of the goals of macroeconometric analysis is to provide quantitative answers to substantive macroeconomic questions. Answers to some questions, such as whether gross domestic product (GDP) will decline over the next two quarters, can be ob- tained with univariate time-series models by simply exploiting serial correlations. Other questions, such as what are the main driving forces of business cycles, re- quire at least a minimal set of restrictions, obtained from theoretical considerations, that allow the identification of structural disturbances in a multivariate time-series model. Finally, macroeconometricians might be confronted with questions demand- ing a sophisticated theoretical model that is able to predict how agents adjust their behavior in response to new economic policies, such as changes in monetary or fiscal policy. 1.1 Challenges for Inference and Decision Making Unfortunately, macroeconometricians often face a shortage of observations necessary for providing precise answers. Some questions require high-dimensional empirical models. For instance, the analysis of domestic business cycles might involve process- ing information from a large cross section of macroeconomic and financial variables. The study of international comovements is often based on highly parameterized mul- ticountry vector autoregressive models. High-dimensional models are also necessary in applications in which it is reasonable to believe that parameters evolve over time, for instance, because of changes in economic policies. Thus, sample information alone is often insufficient to enable sharp inference about model parameters and im- plications. Other questions do not necessarily require a very densely parameterized empirical model, but they do demand identification restrictions that are not self- evident and that are highly contested in the empirical literature. For instance, an unambiguous measurement of the quantitative response of output and inflation to an unexpected reduction in the federal funds rate remains elusive. Thus, document- ing the uncertainty associated with empirical findings or predictions is of first-order importance for scientific reporting. Many macroeconomists have a strong preference for models with a high degree of theoretical coherence such as dynamic stochastic general equilibrium (DSGE) mod- els. In these models, decision rules of economic agents are derived from assumptions Del Negro, Schorfheide – Bayesian Macroeconometrics: April 18, 2010 2 about agents’ preferences and production technologies and some fundamental prin- ciples such as intertemporal optimization, rational expectations, and competitive equilibrium. In practice, this means that the functional forms and parameters of equations that describe the behavior of economic agents are tightly restricted by op- timality and equilibrium conditions. Thus, likelihood functions for empirical models with a strong degree of theoretical coherence tend to be more restrictive than like- lihood functions associated with atheoretical models. A challenge arises if the data favor the atheoretical model and the atheoretical model generates more accurate forecasts, but a theoretically coherent model is required for the analysis of a partic- ular economic policy. 1.2 How Can Bayesian Analysis Help? In Bayesian inference, a prior distribution is updated by sample information con- tained in the likelihood function to form a posterior distribution. Thus, to the extent that the prior is based on nonsample information, it provides the ideal framework for combining di↵erent sources of information and thereby sharpening inference in macroeconometric analysis. This combination of information sets is prominently used in the context of DSGE model inference in Section 4. Through informative prior distributions, Bayesian DSGE model inference can draw from a wide range of data sources that are (at least approximately) independent of the sample informa- tion. These sources might include microeconometric panel studies that are infor- mative about aggregate elasticities or long-run averages of macroeconomic variables that are not included in the likelihood function because the DSGE model under consideration is too stylized to be able to explain their cyclical fluctuations. Many macroeconometric models are richly parameterized. Examples include the vector autoregressions (VARs) with time-varying coefficients in Section 5 and the multicountry VARs considered in Section 6. In any sample of realistic size, there will be a shortage of information for determining the model coefficients, leading to very imprecise inference and di↵use predictive distributions. In the context of time-varying coefficient models, it is often appealing to conduct inference under the assumption that either coefficient change only infrequently, but by a potentially large amount, or that they change frequently, but only gradually. Such assumptions can be conveniently imposed by treating the sequence of model parameters as a Del Negro, Schorfheide – Bayesian Macroeconometrics: April 18, 2010 3 stochastic process, which is of course nothing but a prior distribution that can be updated with the likelihood function. To reduce the number of parameters in a high-dimensional VAR, one could of course set many coefficients equal to zero or impose the condition that the same co- efficient interacts with multiple regressors. Unfortunately, such hard restrictions rule out the existence of certain spillover e↵ects, which might be undesirable. Conceptu- ally more appealing is the use of soft restrictions, which can be easily incorporated through probability distributions for those coefficients that are “centered” at the desired restrictions but that have a small, yet nonzero, variance. An important and empirically successful example of such a prior is the Minnesota prior discussed in Section 2. An extreme version of lack of sample information arises in the context of struc- tural VARs, which are studied in Section 2. Structural VARs can be parameterized in terms of reduced-form parameters, which enter the likelihood function, and an orthogonal matrix ⌦, which does not enter the likelihood function. Thus, ⌦is not identifiable based on the sample information. In this case, the conditional distri- bution of ⌦given the reduced-form parameters will not be updated, and its condi- tional posterior is identical to the conditional prior. Identification issues also arise in the context of DSGE models. In general, as long as the joint prior distribution of reduced-form and nonidentifiable parameters is proper, meaning that the total probability mass is one, so is the joint posterior distribution. In this sense, the lack of identification poses no conceptual problem in a Bayesian framework. However, it does pose a challenge: it becomes more important to document which aspects of the prior distribution are not updated by the likelihood function and to recognize the extreme sensitivity of those aspects to the specification of the prior distribution. Predictive distributions of future observations
Details
-
File Typepdf
-
Upload Time-
-
Content LanguagesEnglish
-
Upload UserAnonymous/Not logged-in
-
File Pages127 Page
-
File Size-