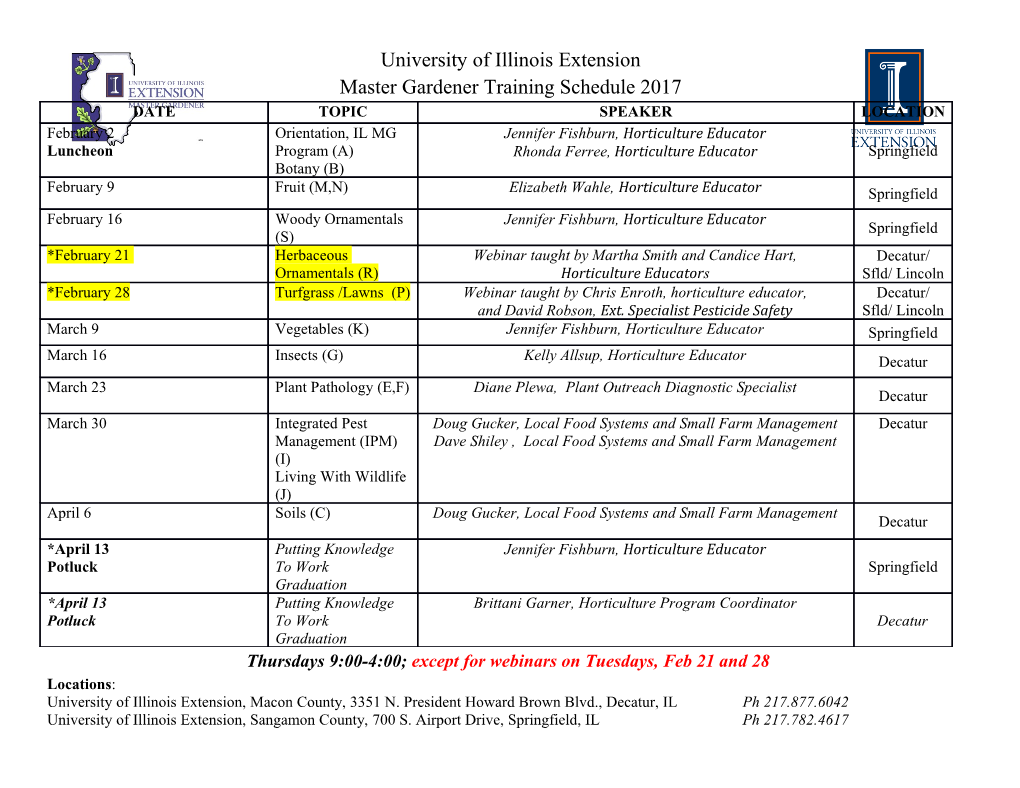
14_TPPT_2015_Sep Agenda item 6.8 Environ Monit Assess (2014) 186:2729–2740 DOI 10.1007/s10661-013-3574-8 Assessing environmentally significant effects: a better strength-of-evidence than a single P value? Graham McBride & Russell G. Cole & Ian Westbrooke & Ian Jowett Received: 28 May 2013 /Accepted: 28 November 2013 /Published online: 20 December 2013 # Springer Science+Business Media Dordrecht 2013 Abstract Interpreting a P value from a traditional nil with two types of equivalence tests (one testing that the hypothesis test as a strength-of-evidence for the exis- difference that does exist is contained within an interval tence of an environmentally important difference be- of indifference, the other testing that it is beyond that tween two populations of continuous variables (e.g. a interval—also known as bioequivalence testing). This chemical concentration) has become commonplace. Yet, gives rise to a strength-of-evidence procedure that lends there is substantial literature, in many disciplines, that itself to a simple confidence interval interpretation. It is faults this practice. In particular, the hypothesis tested is accompanied by a strength-of-evidence matrix that has virtually guaranteed to be false, with the result that P many desirable features: not only a strong/moderate/ depends far too heavily on the number of samples dubious/weak categorisation of the results, but also rec- collected (the ‘sample size’). The end result is a swing- ommendations about the desirability of collecting fur- ing burden-of-proof (permissive at low sample size but ther data to strengthen findings. precautionary at large sample size). We propose that these tests be reinterpreted as direction detectors (as Keywords Environmental significance . P value . has been proposed by others, starting from 1960) and Strength-of-evidence . Confidence interval that the test’s procedure be performed simultaneously Electronic supplementary material The online version of this Introduction article (doi:10.1007/s10661-013-3574-8) contains supplementary material, which is available to authorized users. Environmental impact assessment frequently relies on G. McBride (*) advice based on statistical hypothesis testing. Like many NIWA (National Institute of Water & Atmospheric Research), others (http://warnercnr.colostate.edu/~anderson/ PO Box 11115, Hamilton 3216, New Zealand thompson1.html), we are of the view that the results e-mail: [email protected] while appearing to be instructive can in fact be R. G. Cole misleading. This is because standard tests (e.g. t tests, NIWA, analysis of variance) are most usually tests of a PO Box 893, Nelson 7010, New Zealand particular form of null hypotheses, i.e. ‘nil hypotheses’ I. Westbrooke (Cohen 1994). Typically denoted as H0, they postulate Department of Conservation, that differences between populations’ parameters (means, Private Bag 4715, Christchurch, New Zealand medians, …)differbyanexact amount, most usually zero. We should have little interest in such an implausible I. Jowett Jowett Consulting Ltd., hypothesis because we already know that such a state 123 Butcher Road, Pukekohe, New Zealand between populations’ parameters is extremely unlikely: 14_TPPT_2015_Sep Agenda item 6.8 2730 Environ Monit Assess (2014) 186:2729–2740 ‘All we know about the world teaches us that the effects have to regard more extreme samples also of A and B are always different’ (Tukey 1991). Yet, the as evidence against H0.Therefore,P is the test’s calculation procedure is derived from key results probability of declaring there to be evi- in the mathematical theory of statistics that are built on dence against H0 when it is in fact true the assumption that the hypothesis to be tested is true. and the data under analysis are regarded That hypothesis is only rejected if the test’s ‘P value’ is as just decisive’ (Cox 1987). Other statisti- less than a chosen significance level (often taken as cians maintain that this line of reasoning α=5 %) in which case the result is called ‘statistically does not resolve the problem of appealing significant’—or sometimes just ‘significant’—and the to data that were not obtained (e.g. Berger ‘alternative hypothesis’ is accepted (i.e. the first and Delampady 1987). And note that, as is population’s mean or median differs from the second). common in much statistical writing, the Conversely, if the P value is greater than α, the best we above quotes assume that H0 may be true, can say is that the hypothesis is ‘not rejected’ which, on whereas we (and others, such as Tukey logical grounds, cannot and should not be taken to mean 1991) say the chances of that being so (for that such a hypothesis can be accepted—although this continuous variables at least) are vanish- incorrect inference is often made. As stated by Zar ingly small. We will propose a possible (1984,p.45):“… failing to reject a null hypothesis is resolution of this disagreement. not ‘proof’ that the hypothesis is true. It denotes only Problem 2 P depends on the number of data collected. that there is not sufficient evidence to conclude that it is Consider a simple t test, addressing the false” (a point first made in print by Berkson 1942). In hypothesis that the difference between the other words, how can it be legitimate to accept as true means of two normal populations is zero in something that we already understand to be false? two populations where the means can be Especially when, as implied by Zar, were more and taken as normally distributed (typically more data to be collected the hypothesis would based on the central limit theorem), with eventually be rejected. common variance. That is, H0 posits that If this significant/not significant dichotomy is aban- μ1−μ2=0, where μ1 and μ2 denote the doned, the practice can arise of using the P value as an unknown population means. The alterna- apparent strength-of-evidence concerning the changes tive hypothesis is HA: μ1−μ2≠0. If we or trends being analysed. This too is generally erroneous draw n samples from each population, we (Germano 1999), on two grounds. To see why, we need can then calculate the test statistic T, which to consider the P value in a little more detail. In partic- follows the t distribution when H0 is true. It pffiffi ular, note that it is defined as the probability of obtaining X 1−X 2 n is defined as T ¼ 2 where X 1 data at least as extreme as has been obtained if the tested Sp hypothesis is true. Two problems arise. and X 2 are the means of each set of sam- ples, Sp is the pooled standard deviation Problem 1 Using P as a strength-of-evidence provided obtained from the variances of the data by the data that we collect is to include in and the vertical bars denote absolute mag- that metric data that we did not collect (i.e. nitude. Observe that the term in vertical all data more extreme than was obtained). bars measures the relative ‘effect size’ in This problem has been criticised and the data (as defined by Cohen 1988,also defended in the statistical literature. A fa- called the ‘normal deviate’) and is com- mous criticism is: ‘What the use of P im- prised of unbiased and consistent estima- plies, therefore, is that a hypothesis that tors of means and variances (sensu the may be true may be rejected because it mathematical theory of statistics, e.g. has not predicted observable results that Freund 1992). As a consequence, were we have not occurred’ (Jeffreys 1961). In its to have taken less than sample size n (i.e. n defence another statistician stated ‘… if we samples) from each population, on aver- were to regard the data under analysis as age, the value of the effect size would just decisive against H0 then we would remain roughly constant. So in essence, 14_TPPT_2015_Sep Agenda item 6.8 Environ Monit Assess (2014) 186:2729–2740 2731 pffiffiffiffiffiffiffiffi 0.4 the presence of the n=2 term means that the test statistic T will be substantially af- fected by the number of data we have. In 0.3 particular, the more data we have from a given population, the larger T will tend to 0.2 be. And because larger values of T auto- Larger tail area matically cause smaller P values, the more when t = 1.6 data we have the smaller P will be (larger Probability density 0.1 Smaller tail area values of T cutoff smaller areas in the tail of when t = 2.3 the t distribution, as shown on Fig. 1). 0 So with many samples, nil hypothesis tests on differ- -4 -3 -2 -1 0 1 2 3 4 ences in population means will routinely return a statisti- t 12 degrees of freedom cally significant result when in fact the differences that are present would be considered by environmental scien- Fig. 1 The larger the test statistic, the smaller the tail area of the t distribution tists to be trivial—reflecting a precautionary approach. Conversely, with only a few samples, environmentally seldom pointed out that P values may have some merits important differences may often be declared to be ‘not when testing hypotheses that can be true, and so, critics significant’—reflecting a permissive approach.1 (See may ignore some possible benefits of including tests of Appendix 1 for a numerical example of how this works.) nil hypotheses in a strength-of-evidence approach (e.g. Now, if the true difference is environmentally important, Fleiss 1986;Frick1995;Chow1996; Hagen 1997, this behaviour is desirable—collecting yet more data Harris 1997a). from a given population can lead to rejection of a false Herein, we present the essentials of an approach to hypothesis that was previously not found to be wanting hypothesis testing that retains useful features of nil (as is implied by the quote from Zar above, a feature well- hypothesis test procedures, marrying them with tests of known among statisticians).
Details
-
File Typepdf
-
Upload Time-
-
Content LanguagesEnglish
-
Upload UserAnonymous/Not logged-in
-
File Pages12 Page
-
File Size-