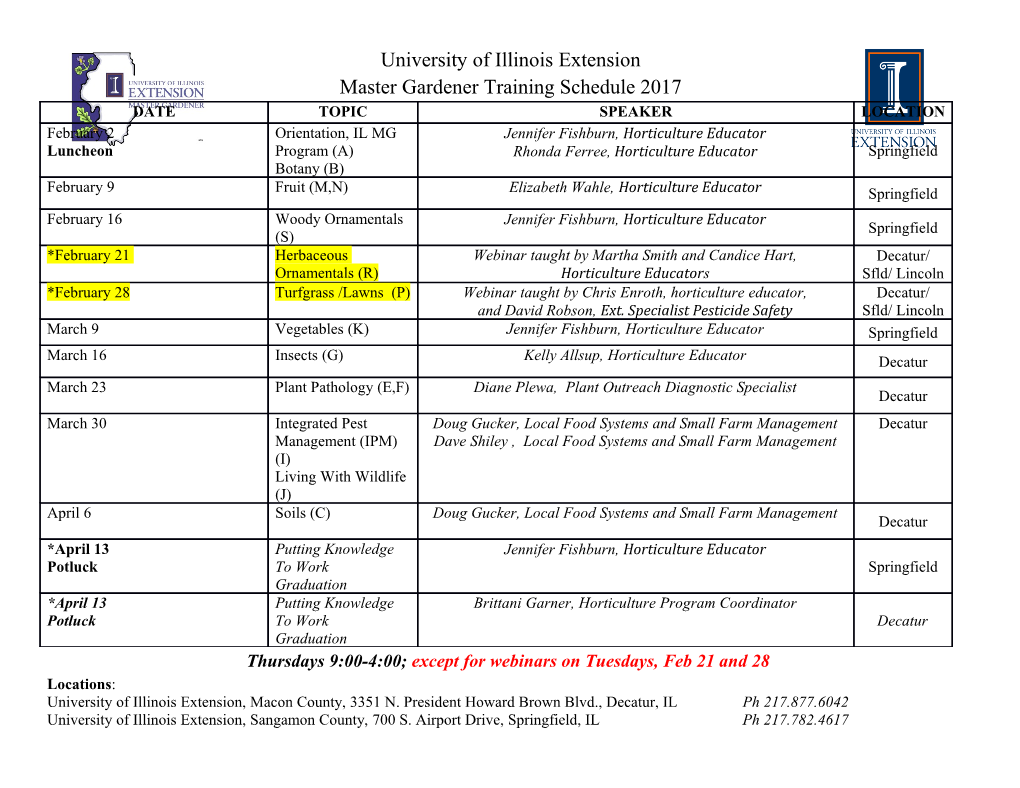
Subgradients Ryan Tibshirani Convex Optimization 10-725/36-725 Last time: gradient descent Consider the problem min f(x) x n for f convex and differentiable, dom(f) = R . Gradient descent: (0) n choose initial x 2 R , repeat (k) (k−1) (k−1) x = x − tk · rf(x ); k = 1; 2; 3;::: Step sizes tk chosen to be fixed and small, or by backtracking line search If rf Lipschitz, gradient descent has convergence rate O(1/) Downsides: • Requires f differentiable next lecture • Can be slow to converge two lectures from now 2 Outline Today: crucial mathematical underpinnings! • Subgradients • Examples • Subgradient rules • Optimality characterizations 3 Subgradients Remember that for convex and differentiable f, f(y) ≥ f(x) + rf(x)T (y − x) for all x; y I.e., linear approximation always underestimates f n A subgradient of a convex function f at x is any g 2 R such that f(y) ≥ f(x) + gT (y − x) for all y • Always exists • If f differentiable at x, then g = rf(x) uniquely • Actually, same definition works for nonconvex f (however, subgradients need not exist) 4 Examples of subgradients Consider f : R ! R, f(x) = jxj 2.0 1.5 1.0 f(x) 0.5 0.0 −0.5 −2 −1 0 1 2 x • For x 6= 0, unique subgradient g = sign(x) • For x = 0, subgradient g is any element of [−1; 1] 5 n Consider f : R ! R, f(x) = kxk2 f(x) x2 x1 • For x 6= 0, unique subgradient g = x=kxk2 • For x = 0, subgradient g is any element of fz : kzk2 ≤ 1g 6 n Consider f : R ! R, f(x) = kxk1 f(x) x2 x1 • For xi 6= 0, unique ith component gi = sign(xi) • For xi = 0, ith component gi is any element of [−1; 1] 7 n Let f1; f2 : R ! R be convex and differentiable, and consider f(x) = maxff1(x); f2(x)g 15 10 f(x) 5 0 −2 −1 0 1 2 x • For f1(x) > f2(x), unique subgradient g = rf1(x) • For f2(x) > f1(x), unique subgradient g = rf2(x) • For f1(x) = f2(x), subgradient g is any point on the line segment between rf1(x) and rf2(x) 8 Subdifferential Set of all subgradients of convex f is called the subdifferential: n @f(x) = fg 2 R : g is a subgradient of f at xg • @f(x) is closed and convex (even for nonconvex f) • Nonempty (can be empty for nonconvex f) • If f is differentiable at x, then @f(x) = frf(x)g • If @f(x) = fgg, then f is differentiable at x and rf(x) = g 9 Connection to convex geometry n n Convex set C ⊆ R , consider indicator function IC : R ! R, ( 0 if x 2 C IC (x) = Ifx 2 Cg = 1 if x2 = C For x 2 C, @IC (x) = NC (x), the normal cone of C at x, recall n T T NC (x) = fg 2 R : g x ≥ g y for any y 2 Cg Why? By definition of subgradient g, T IC (y) ≥ IC (x) + g (y − x) for all y • For y2 = C, IC (y) = 1 • For y 2 C, this means 0 ≥ gT (y − x) 10 ● ● ● ● 11 Subgradient calculus Basic rules for convex functions: • Scaling: @(af) = a · @f provided a > 0 • Addition: @(f1 + f2) = @f1 + @f2 • Affine composition: if g(x) = f(Ax + b), then @g(x) = AT @f(Ax + b) • Finite pointwise maximum: if f(x) = maxi=1;:::m fi(x), then [ @f(x) = conv @fi(x) i:fi(x)=f(x) the convex hull of union of subdifferentials of all active functions at x 12 • General pointwise maximum: if f(x) = maxs2S fs(x), then [ @f(x) ⊇ cl conv @fs(x) s:fs(x)=f(x) and under some regularity conditions (on S; fs), we get an equality above • Norms: important special case, f(x) = kxkp. Let q be such that 1=p + 1=q = 1, then T kxkp = max z x kzkq≤1 Hence @f(x) = argmax zT x kzkq≤1 13 Why subgradients? Subgradients are important for two reasons: • Convex analysis: optimality characterization via subgradients, monotonicity, relationship to duality • Convex optimization: if you can compute subgradients, then you can minimize (almost) any convex function 14 Optimality condition For any f (convex or not), f(x?) = min f(x) () 0 2 @f(x?) x I.e., x? is a minimizer if and only if 0 is a subgradient of f at x?. This is called the subgradient optimality condition Why? Easy: g = 0 being a subgradient means that for all y f(y) ≥ f(x?) + 0T (y − x?) = f(x?) Note the implication for a convex and differentiable function f, with @f(x) = frf(x)g 15 Derivation of first-order optimality Example of the power of subgradients: we can use what we have learned so far to derive the first-order optimality condition. Recall that for f convex and differentiable, the problem min f(x) subject to x 2 C x is solved at x if and only if rf(x)T (y − x) ≥ 0 for all y 2 C Intuitively says that gradient increases as we move away from x. How to see this? First recast problem as min f(x) + IC (x) x Now apply subgradient optimality: 0 2 @(f(x) + IC (x)) 16 But 0 2 @ f(x) + IC (x) () 0 2 frf(x)g + NC (x) () − rf(x) 2 NC (x) () − rf(x)T x ≥ −∇f(x)T y for all 2 C () rf(x)T (y − x) ≥ 0 for all y 2 C as desired Note: the condition 0 2 @f(x) + NC (x) is a fully general condition for optimality in a convex problem. But this is not always easy to work with (KKT conditions, later, are easier) 17 Example: lasso optimality conditions n n×p Given y 2 R , X 2 R , lasso problem can be parametrized as: 1 2 min ky − Xβk2 + λkβk1 β2Rp 2 where λ ≥ 0. Subgradient optimality: 1 0 2 @ ky − Xβk2 + λkβk 2 2 1 T () 0 2 −X (y − Xβ) + λ∂kβk1 () XT (y − Xβ) = λv for some v 2 @kβk1, i.e., 8 f1g if β > 0 <> i vi 2 {−1g if βi < 0 ; i = 1; : : : p > :[−1; 1] if βi = 0 18 Write X1;:::Xp for columns of X. Then subgradient optimality reads: ( T Xi (y − Xβ) = λ · sign(βi) if βi 6= 0 T jXi (y − Xβ)j ≤ λ if βi = 0 Note: the subgradient optimality conditions do not directly lead to an expression for a lasso solution ... however they do provide a way to check lasso optimality They are also helpful in understanding the lasso estimator; e.g., if T jXi (y − Xβ)j < λ, then βi = 0 19 Example: soft-thresholding Simplfied lasso problem with X = I: 1 2 min ky − βk2 + λkβk1 β2Rn 2 This we can solve directly using subgradient optimality. Solution is β = Sλ(y), where Sλ is the soft-thresholding operator: 8 y − λ if y > λ <> i i [Sλ(y)]i = 0 if − λ ≤ yi ≤ λ ; i = 1; : : : n > :yi + λ if yi < −λ Check: from last slide, subgradient optimality conditions are ( yi − βi = λ · sign(βi) if βi 6= 0 jyi − βij ≤ λ if βi = 0 20 Now plug in β = Sλ(y) and check these are satisfied: • When yi > λ, βi = yi − λ > 0, so yi − βi = λ = λ · 1 • When yi < −λ, argument is similar • When jyij ≤ λ, βi = 0, and jyi − βij = jyij ≤ λ 1.0 0.5 Soft-thresholding in 0.0 one variable: −0.5 −1.0 −1.0 −0.5 0.0 0.5 1.0 21 Example: distance to a convex set Recall the distance function to a convex set C: dist(x; C) = min ky − xk2 y2C This is a convex function. What are its subgradients? Write dist(x; C) = kx − PC (x)k2, where PC (x) is the projection of x onto C. Then when dist(x; C) > 0, x − P (x) @dist(x; C) = C kx − PC (x)k2 Only has one element, so in fact dist(x; C) is differentiable and this is its gradient 22 We will only show one direction, i.e., that x − P (x) C 2 @dist(x; C) kx − PC (x)k2 Write u = PC (x). Then by first-order optimality conditions for a projection, (u − x)T (y − u) ≥ 0 for all y 2 C Hence C ⊆ H = fy :(u − x)T (y − u) ≥ 0g Claim: for any y, (x − u)T (y − u) dist(y; C) ≥ kx − uk2 Check: first, for y 2 H, the right-hand side is ≤ 0 23 T Now for y2 = H, we have (x − u) (y − u) = kx − uk2ky − uk2 cos θ where θ is the angle between x − u and y − u. Thus (x − u)T (y − u) = ky − uk2 cos θ = dist(y; H) ≤ dist(y; C) kx − uk2 as desired Using the claim, we have for any y (x − u)T (y − x + x − u) dist(y; C) ≥ kx − uk2 x − u T = kx − uk2 + (y − x) kx − uk2 Hence g = (x − u)=kx − uk2 is a subgradient of dist(x; C) at x 24 References and further reading • S. Boyd, Lecture notes for EE 264B, Stanford University, Spring 2010-2011 • R. T. Rockafellar (1970), \Convex analysis", Chapters 23{25 • L. Vandenberghe, Lecture notes for EE 236C, UCLA, Spring 2011-2012 25.
Details
-
File Typepdf
-
Upload Time-
-
Content LanguagesEnglish
-
Upload UserAnonymous/Not logged-in
-
File Pages25 Page
-
File Size-