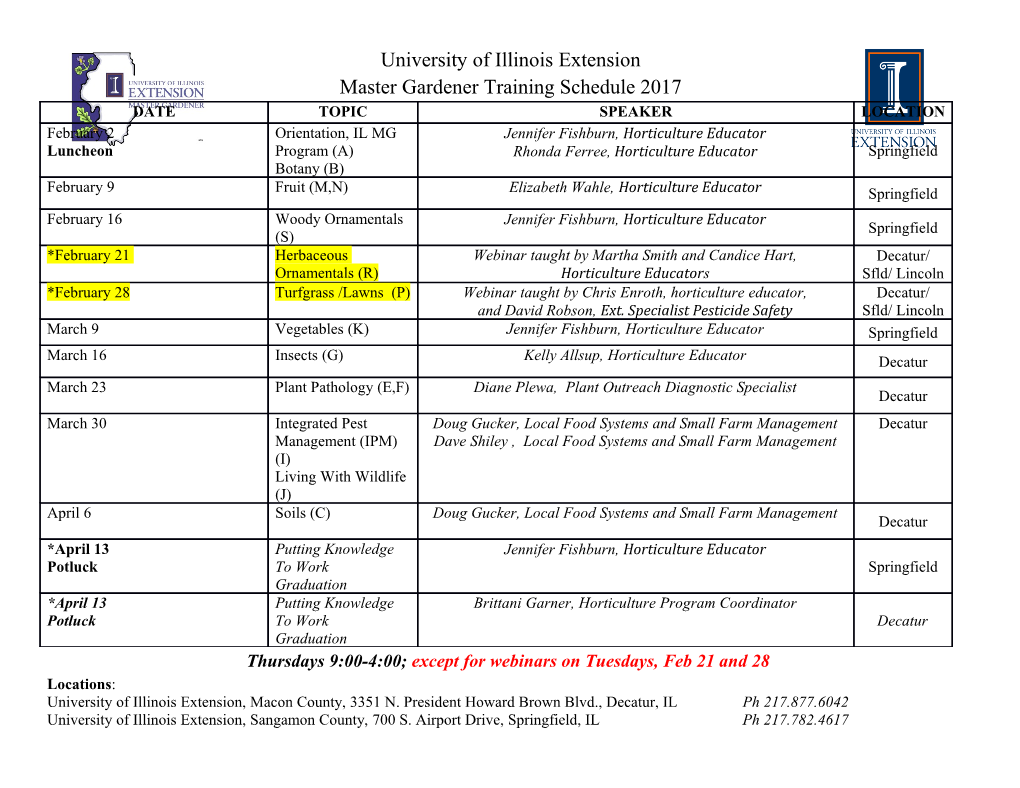
Efficient Query Processing with Optimistically Compressed Hash Tables & Strings in the USSR Tim Gubner Viktor Leis Peter Boncz CWI Friedrich Schiller University Jena CWI [email protected] [email protected] [email protected] Abstract—Modern query engines rely heavily on hash tables Hash Table Optimistically Compressed Hash Table for query processing. Overall query performance and memory Hot Area Cold Area footprint is often determined by how hash tables and the tuples within them are represented. In this work, we propose three complementary techniques to improve this representation: Speculate & Domain-Guided Prefix Suppression bit-packs keys and values Compress tightly to reduce hash table record width. Optimistic Splitting decomposes values (and operations on them) into (operations on) frequently-accessed and infrequently-accessed value slices. By removing the infrequently-accessed value slices from the hash 24 byte 8 byte 8 byte table record, it improves cache locality. The Unique Strings Self- Fig. 1: Optimistically Compressed Hash Table, which is split aligned Region (USSR) accelerates handling frequently-occurring strings, which are very common in real-world data sets, by into a thin hot area and a cold area for exceptions creating an on-the-fly dictionary of the most frequent strings. This allows executing many string operations with integer logic While heavyweight compression schemes tend to result in and reduces memory pressure. larger space savings, they also have a high CPU overhead, We integrated these techniques into Vectorwise. On the TPC-H benchmark, our approach reduces peak memory consumption by which often cannot be amortized by improved cache locality. 2–4× and improves performance by up to 1.5×. On a real-world Therefore, we propose a lightweight compression technique BI workload, we measured a 2× improvement in performance called Domain-Guided Prefix Suppression. It saves space by and in micro-benchmarks we observed speedups of up to 25×. using domain information to re-pack multiple columns in- flight in the query pipeline into much fewer machine words. I. INTRODUCTION For each attribute, this requires only a handful of simple In modern query engines, many important operators like bit-wise operations, which are easily expressible in SIMD join and group-by are based on in-memory hash tables. Hash instructions, resulting in extremely low per-tuple cost (sub- joins, for example, are usually implemented by materializing cycle) for packing and subsequent unpacking operations. the whole inner (build) relation into a hash table. Hash tables Rather than saving space, the second technique, called Opti- are therefore often large and determine the peak memory mistic Splitting, aims at improving cache locality. As Figure 1 consumption of a query. Since hash table sizes often exceed the illustrates, it splits the hash table into a hot (frequently- capacity of the CPU cache, memory latency or bandwidth be- accessed) and a cold (infrequently-accessed) area containing come the performance bottleneck in query processing. Due to exceptions. These exceptions may, for example, be overflow the complex cache-hierarchy of modern CPUs, the access time bits in aggregations, or pointers to string data. By separating to a random tuple varies by orders of magnitude depending on hot from cold information, Optimistic Splitting improves cache the size of the working set. This means that shrinking hash utilization even further. tables does not only reduce memory consumption but also has An often ignored, yet costly part of query processing is the potential of improving query performance through better string handling. String data is very common in many real- CPU cache utilization [3], [4], [26]. world applications [22], [31]. In comparison with integers, To decrease a hash table’s hunger for memory and, con- strings occupy more space, are much slower to process, and are sequently, increase cache-efficiency, one can combine two less amenable to acceleration using SIMD. To speed up string orthogonal approaches: to increase the fill factor and to reduce processing, we therefore propose the Unique Strings Self- the bucket/row size. Several hash table designs like Robin aligned Region (USSR), an efficient dynamic string dictionary Hood Hashing [8], Cuckoo Hashing [23], and the Concise for frequently-occurring strings. In contrast to conventional Hash Table [4] have been proposed for achieving high fill per-column dictionaries used in storage, the USSR is created factors while still providing good lookup performance. Here, anew for each query by inserting frequently-occurring strings we investigate how the size of each row can be reduced—a at query runtime. The USSR, which has a fixed size of topic that, despite its obvious importance for query processing, 768 kB (ensuring its cache residency), speeds up common has not received as much attention. string operations like hashing and equality checks. 1 Preprocessing on meta data we describe Domain-Guided Prefix Suppression in detail using Per-block Total Normalized #Bits min/max min/max domain required Figure 2 as an illustration. A B A B A B A B A. Domain Derivation [-4, 42] [3, 23] [-4, 42] [3, 1k] [0, 46] [0, 997]6 10 [1, 23] [3, 1k] A column in-flight in a query plan can originate directly Bitwise layout [23, 90] [0, 3k] X A B from a table scan or from a computation. If a value origi- ... ... Mask 0xF...F 0xF...F nates from a table scan, we determine its domain based on InShift 0 0 the scanned blocks. For each block we utilize per-column OutShift 0 6 minimum and maximum information (called ZoneMaps or Min/Max indices). This information is typically not stored Packing during query execution inside the block itself as this would require scanning the Data Normalized Packed u = (A[i] >> 0) block (potentially fetching it from disk) before this informa- & 0xF...F X A B A B tion can be extracted. Instead, the meta-data is stored “out- 42 3 46 0 46 v = (B[i] >> 0) of-band” (e.g. in a row-group header, file footer or inside -4 23 0 20 & 0xF...F 1280 the catalog). By knowing the range of blocks that will be 1 1k 5 997 C[i] = (u << 0) 63813 23 3 27 0 | (v << 6) 27 scanned, the domain can be calculated by computing the total minimum/maximum over the range. 32-bit 64-bit 32-bit On the other hand, if a value stems from a computa- Fig. 2: Domain-Guided Prefix Suppression tion, the domain minimum and maximum can be derived bottom up according to the functions used, based on the While each of the proposed techniques may appear simple minimum/maximum bounds on its inputs under assumption in isolation, they are highly complementary. For example, of the worst case. Consider, for example, the addition of using all three techniques, strings from a low-cardinality two integers a 2 [amin; amax] and b 2 [bmin; bmax] resulting domain can be represented using only a small number of in r 2 [rmin; rmax]. To calculate rmin and rmax we have bits. Furthermore, our techniques remap operations on wide to assume the worst-case that means the smallest (rmin), columns into operations on multiple thin columns using a few respective highest (rmax), result of the addition. In case of extra primitive functions (such as pack and unpack). As such, an addition this boils down to rmin = amin + bmin and they can easily be integrated into existing systems and do rmax = amax + bmax. not require extensive modifications of the query processing Depending on these domain bounds, an addition of two 32- algorithms or even hash table implementations. bit integer expressions could still fit in a 32-bit result, or less Rather than merely implementing our approach as a proto- likely, would have to be extended to 64-bit. This analysis of type, we fully integrated it into Vectorwise which originated minimum/maximum bounds often can allow implementations from the MonetDB/X100 project [7]. Describing how to to ignore overflow handling, as the choice of data types integrate the three techniques into industrial-strength database prevents overflow, rather than having to check for it. For systems is a key contribution of the paper. aggregation functions such as SUM, overflow avoidance is Based on Vectorwise, we performed an extensive experi- more challenging but in Section III-A, we discuss Optimistic mental evaluation using TPC-H, micro-benchmarks, and real- Splitting, which allows to do most calculations on small data world workloads. On TPC-H, we reduce peak memory con- types, also reducing the cache footprint of aggregates. sumption by 2–4× and improve performance by up to 1.5×. B. Prefix Suppression On a string-heavy real-world BI workload, we measured a Using the derived domain bounds, we can represent values 2.2× improvement in performance, and in micro-benchmarks compactly without losing any information by dropping the we even observed speedups of up to 25×. common prefix bits. To further reduce the number of bits and enable the compression of negative values, we first subtract II. DOMAIN-GUIDED PREFIX SUPPRESSION the domain minimum from each value. Consequently, each Domain-Guided Prefix Suppression reduces memory con- bit-packed value is a positive offset to the domain minimum. sumption by eliminating the unnecessary prefix bits of each We also pack multiple columns together such that the packed attribute. This enables us to cheaply compress rows without result fits a machine word. This is done by concatenating all affecting the implementation of the hash table itself, which compressed bit-strings, and (if necessary) chunk the result into makes it easy to integrate our technique into existing database multiple machine words. Each chunk of the result constitutes systems. In particular, while our system (Vectorwise) uses a compressed column which can be stored just like a regular a single-table hash join, Domain-Guided Prefix Suppression uncompressed column.
Details
-
File Typepdf
-
Upload Time-
-
Content LanguagesEnglish
-
Upload UserAnonymous/Not logged-in
-
File Pages12 Page
-
File Size-